Rolling Bearing Fault Diagnosis Based on Attention LSTM
2023 38th Youth Academic Annual Conference of Chinese Association of Automation (YAC)(2023)
关键词
Fault diagnosis,LSTM,Attention,Rolling bearings
AI 理解论文
溯源树
样例
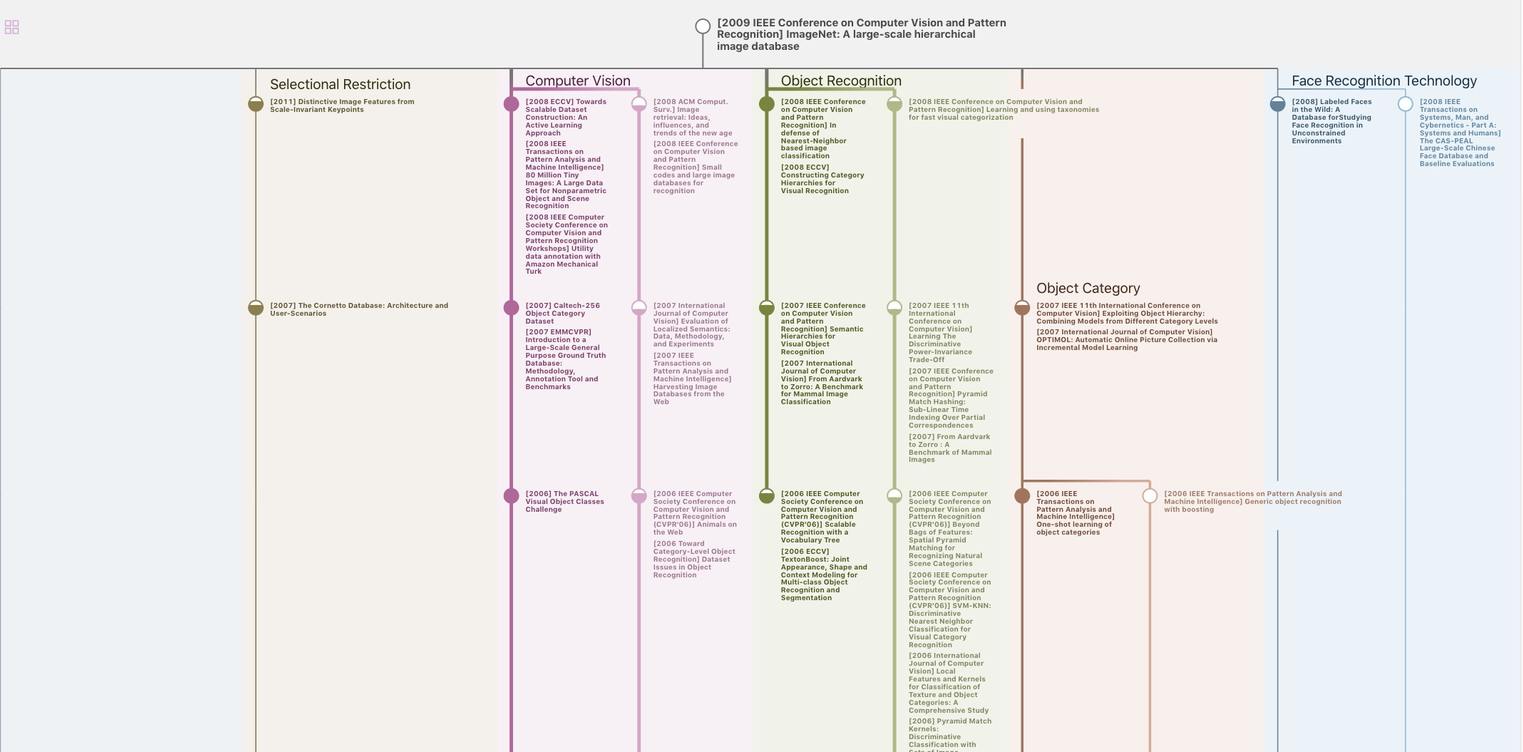
生成溯源树,研究论文发展脉络
Chat Paper
正在生成论文摘要
2023 38th Youth Academic Annual Conference of Chinese Association of Automation (YAC)(2023)