Training Minimal Complexity Support Vector Machines with Multiple Kernels.
2023 IEEE International Conference on Systems, Man, and Cybernetics (SMC)(2023)
摘要
The minimal complexity support vector machine (ML1 SVM), which is a fusion of the standard support vector machine (L1 SVM) and the minimal complexity machine (MCM), works to improve the generalization ability over the L1 SVM. In this paper, we introduce a three-kernel structure into the ML1 SVM. We call it ML1 SVM (MK). We speed up model selection by three-stage cross-validation: In the first stage, we determine the hyperparameters for the L1 SVM (MK) fixing the hyperparameters for multiple kernels. In the second stage we determine the hyperparameters for the multiple kernels, and in the third stage, we determine the hyperparameter for the ML1 SVM (MK) for controlling the maximum margin. We also discuss a method for accelerating the second stage of model selection. In the computer experiment using two-class and multiclass problems, the ML1 SVM (MK) showed statistically comparable to, or better than the L1 SVM (MK), ML1 SVM, and L1 SVM.
更多查看译文
关键词
maximum margin,minimum margin,support vector machines
AI 理解论文
溯源树
样例
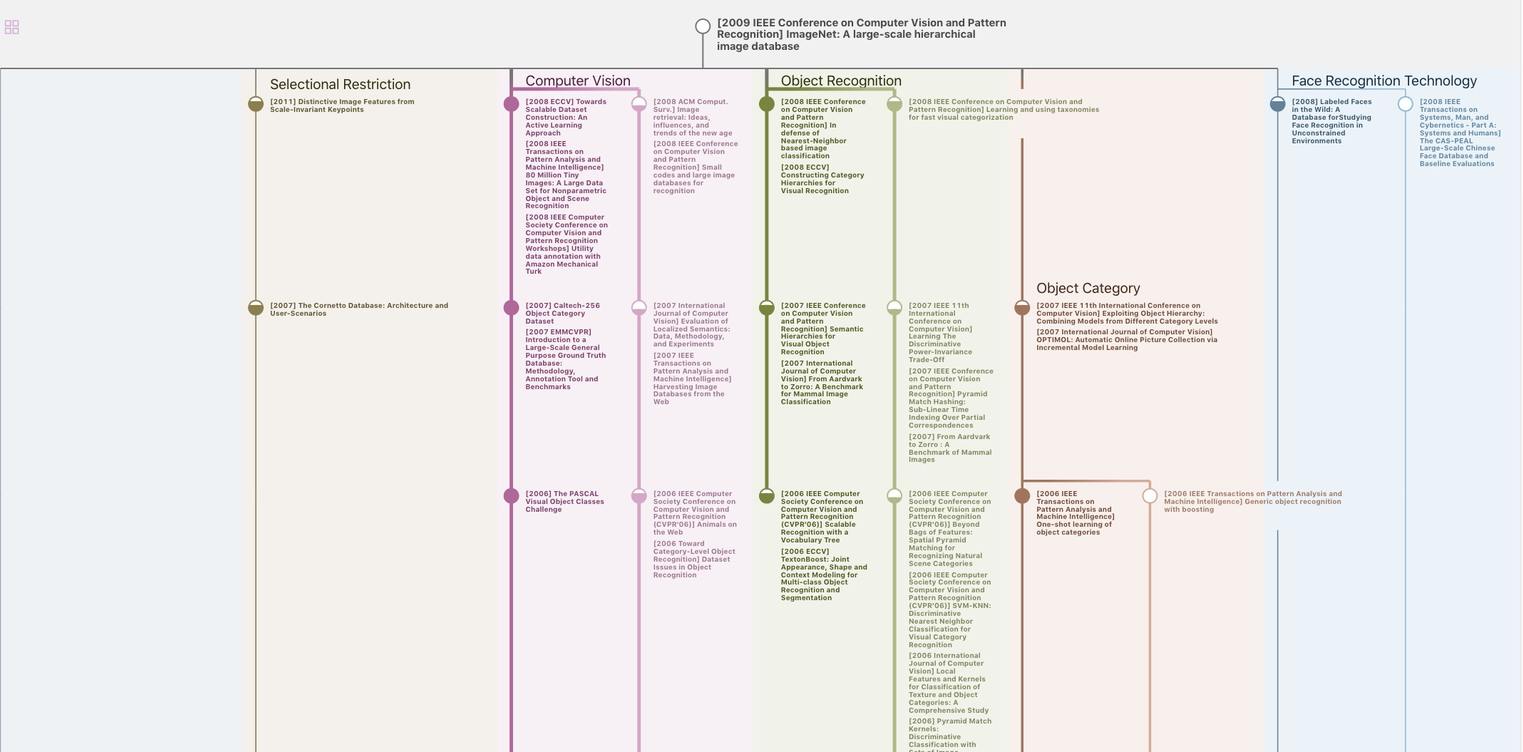
生成溯源树,研究论文发展脉络
Chat Paper
正在生成论文摘要