Hierarchical Cross-Scale Graph Reasoning Network for Retinal Vessel Segmentation.
2023 IEEE International Conference on Systems, Man, and Cybernetics (SMC)(2023)
摘要
Retinal vessel segmentation holds considerable importance in clinical diagnostics for microvascular and oph-thalmological diseases. Deep learning-based methodologies have demonstrated impressive results in this task. However, challenges persist due to the complex structures exhibiting scale variation and the limited capacity of CNN models for long-range relationships. In this paper, we propose a U-shaped network that incorporates our proposed hierarchical cross-scale graph reasoning module for retinal vessel segmentation. After encoding, the features are transformed from spatial domain to graph domain with different scale setting to capture various long-range features and model correlations global contextual information between graphs at different scales. Graph convolution networks are utilized to propagate node features in intra-graph and cross-scale graph interaction is adopted to reason and enhance features for target graph. After that, we concatenate all features transformed back from the graph domain and decode. We evaluated our proposed method on two widely-used datasets DRIVE, CHASE_DB1, and compared it with multiple approaches. Experimental results demonstrate the effectiveness of our proposed approach in retinal vessel segmentation.
更多查看译文
关键词
Retinal Vessel Segmentation,Hierarchical Graph Reasoning,Cross-Scale Graph
AI 理解论文
溯源树
样例
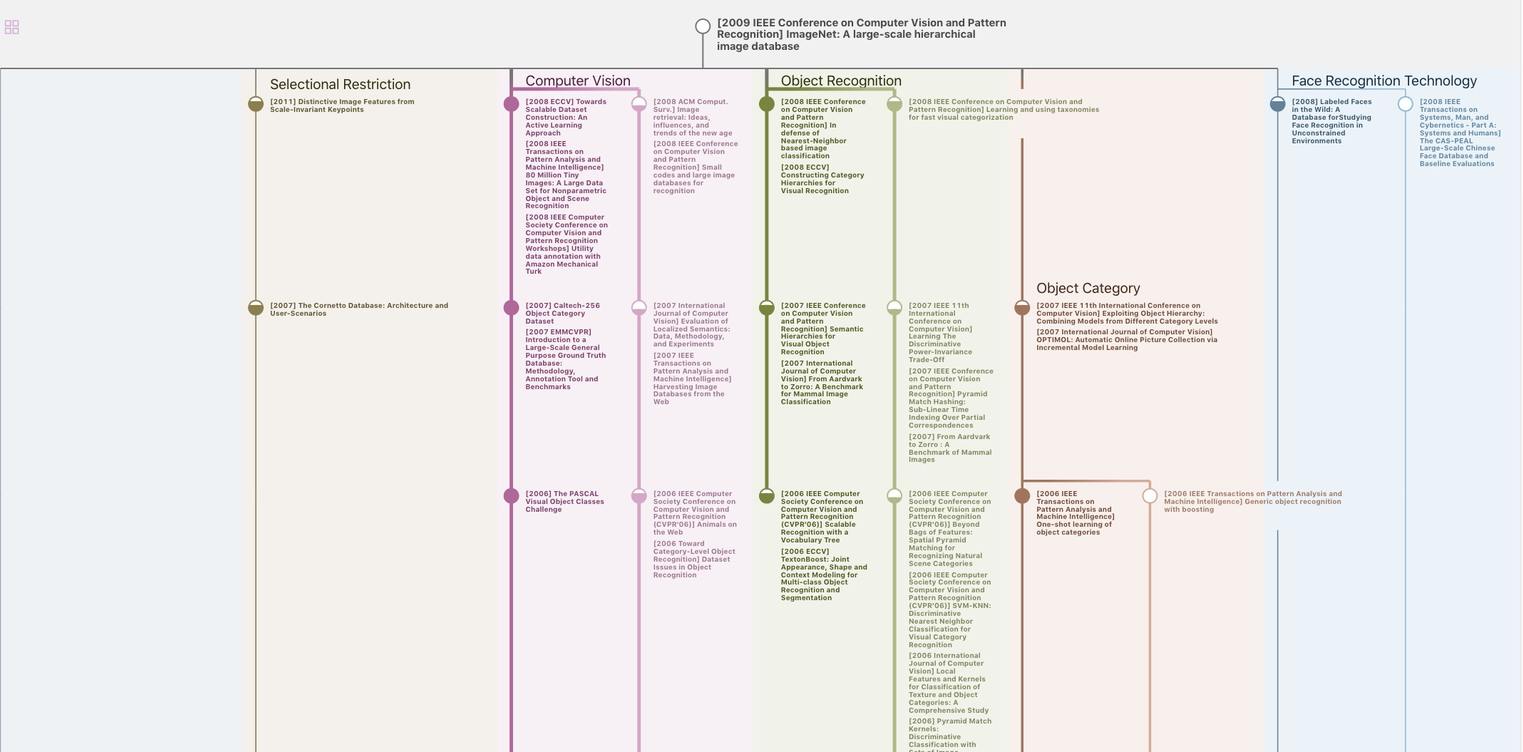
生成溯源树,研究论文发展脉络
Chat Paper
正在生成论文摘要