Learning Quantum Distributions Based on Normalizing Flow.
2023 IEEE International Conference on Systems, Man, and Cybernetics (SMC)(2023)
摘要
Learning many-body quantum systems is of fundamental importance in quantum information processing, however, it is a challenging task which typically requires estimating quantum distributions of dimensionality exponentially scaling to the system size. As generative models have shown a great scalability to learn high-dimensional distributions and found wide applications in the domain of image and text, they can be a powerful tool to facilitate us to accomplish the challenging quantum tasks. In this work, we propose using normalizing flow (NF) models with fast sampling to learn discrete quantum distributions for quantum state tomography. Particularly, three NF models, including denoising flow, argmax flow, and tree flow, are first adapted to the task of explicit quantum probability density estimation. We then perform extensive experiments on a large scale of quantum systems, and our numerical results demonstrate that these discrete NFs admit an excellent sampling efficiency in the sense that they are insensitive to the system size to learn the high-dimensional quantum distributions, without compromising the learning performance. Finally, in comparison to the other generative models, such as autoregressive, the NFs avoid the problem of slow sequential sampling.
更多查看译文
关键词
quantum distribution,quantum state tomography,normalizing flow,autoregressive models
AI 理解论文
溯源树
样例
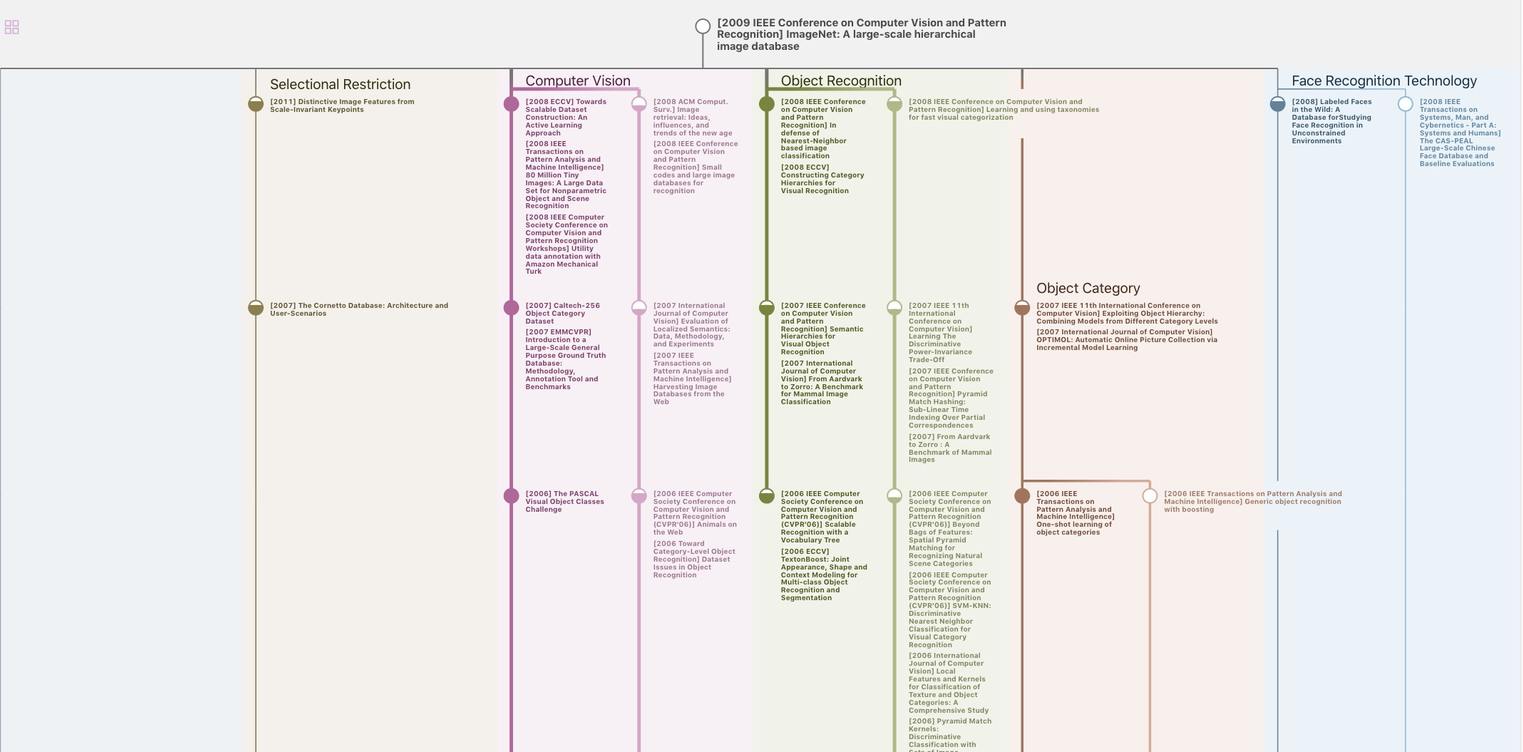
生成溯源树,研究论文发展脉络
Chat Paper
正在生成论文摘要