Improving Communication Efficiency: A Dual Adaptive Federated Learning Framework
2023 38th Youth Academic Annual Conference of Chinese Association of Automation (YAC)(2023)
摘要
As internet technology continues to advance, there is a growing recognition of the significance of safeguarding data privacy. Federated Learning (FL) has emerged as a noteworthy approach for preserving data privacy. Nevertheless, a common challenge in FL is the non-Independently and Identically Distributed (non-IID) nature of the data. This can lead to a misalignment between the optimal solution achieved by each client and the globally optimal solution. The client drift problem greatly reduces the convergence rate and communication efficiency of FL. This paper introduces a dual adaptive FL framework (FedDA) to achieve efficient communication for FL. During client training, FedDA uses adaptive temporal correction factors to alleviate client drift and avoid optimizing towards local optima, thereby improving model training quality and convergence rate. During server aggregation, FedDA uses a forward-looking adaptive optimization algorithm to accelerate global model aggregation, resulting in enhanced communication efficiency achieved through a reduction in the requisite number of communication rounds for model convergence. Extensive experimentation across various datasets validates that the introduced FedDA can significantly accelerate model convergence, improve model communication efficiency, and exhibit robustness to different FL settings.
更多查看译文
关键词
federated learning,communication efficiency,dual adaptation,client drift
AI 理解论文
溯源树
样例
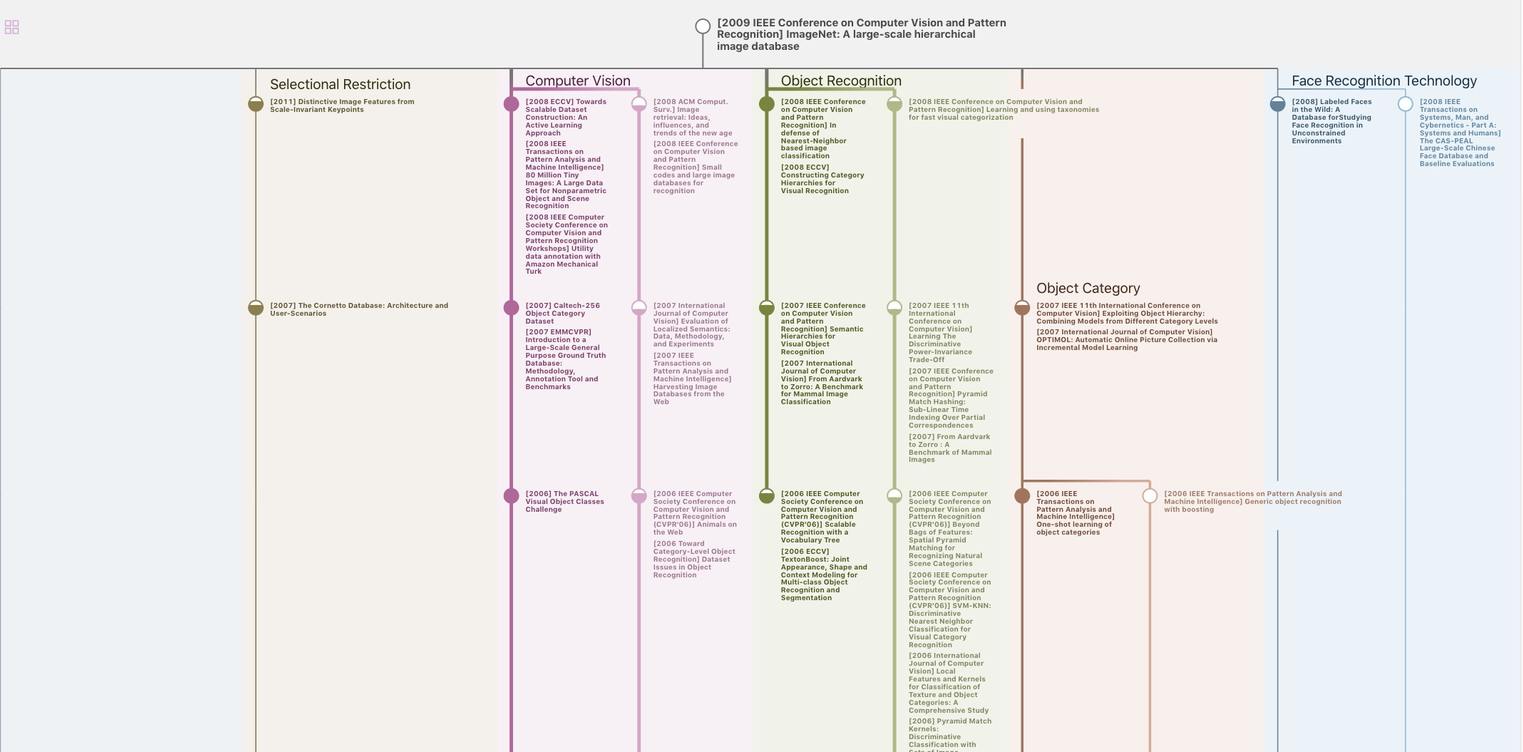
生成溯源树,研究论文发展脉络
Chat Paper
正在生成论文摘要