Dynamic Equilibrium-Based Continual Learning Model with Disentangled Meta-features.
2023 IEEE International Conference on Systems, Man, and Cybernetics (SMC)(2023)
摘要
The field of artificial intelligence research has witnessed remarkable advancements in recent decades. How-ever, conventional approaches in AI research primarily depend on fixed datasets and stationary settings, which have limited applicability to real-world scenarios. In order to address this limitation, there is an increasing need to develop and study algorithms and methods for continual learning, which enables artificial systems to learn from a continuous stream of data. One of the key challenges in continual learning is to strike a balance between transfer and interference, and to identify an equilibrium solution that can effectively learn from non-stationary data. This paper presents a canonical model that is specifically designed for continual learning, utilizing the derivative of the loss function to evaluate parameter changes between tasks and achieve dynamic equilibrium. Additionally, to improve the efficiency of limited training samples in continual tasks, a feature learning method based on meta-feature disentangling is proposed. By leveraging the second derivative term of the canonical model, the parameter vector can be decoupled and meta-features can be discovered. Experimental results demon-strate the superiority of the proposed method over state-of-the-art methods in continual lifelong supervised learning benchmarks. The validity of the proposed canonical model is further supported by these experimental results. As the demands of available settings become increasingly stringent, the advantages of disentangling meta-features become more prominent, resulting in a significant performance gap with other continual learning methods.
更多查看译文
关键词
Continual learning,Meta-feature,Disentangling
AI 理解论文
溯源树
样例
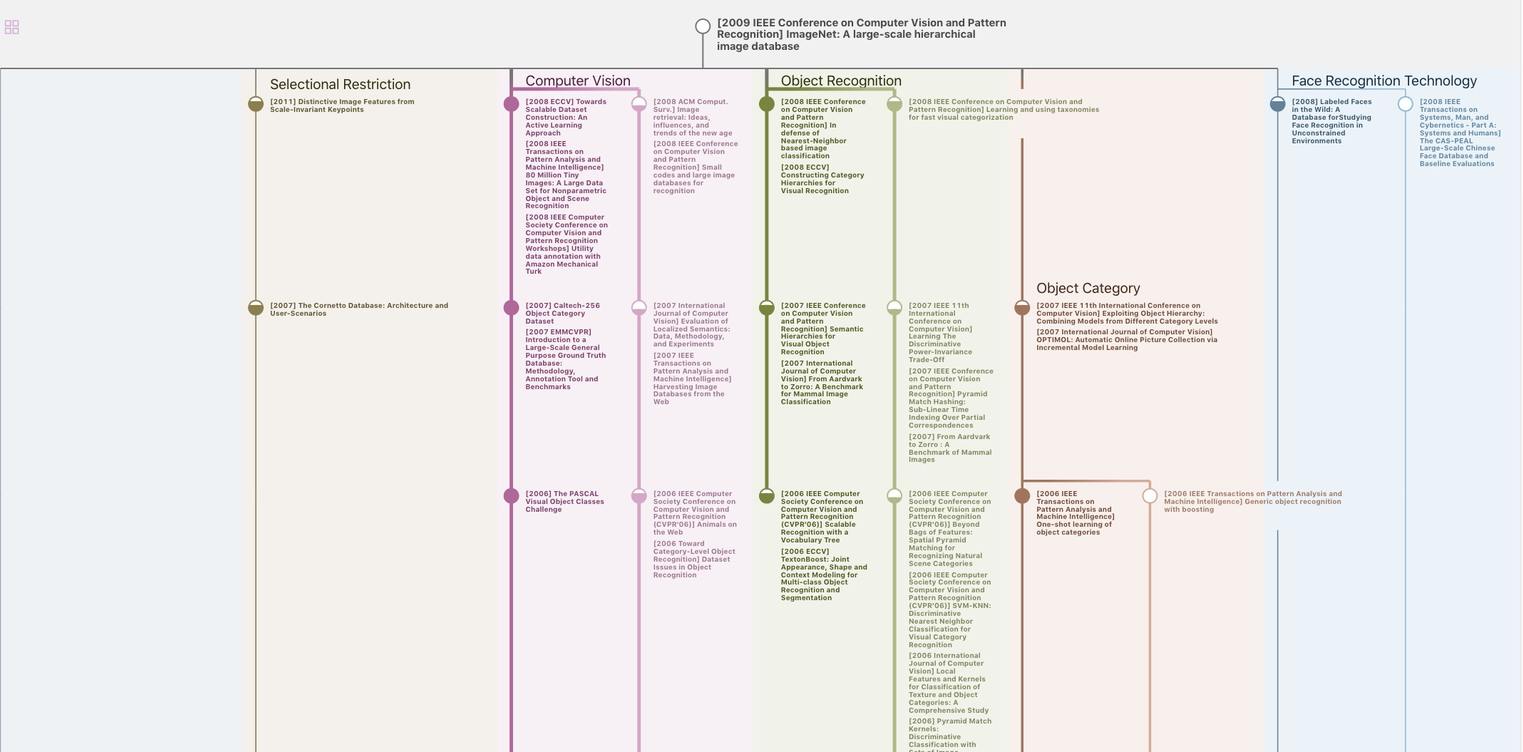
生成溯源树,研究论文发展脉络
Chat Paper
正在生成论文摘要