Sentence Reconstruction Leveraging Contextual Meaning from Speech-Related Brain Signals.
2023 IEEE International Conference on Systems, Man, and Cybernetics (SMC)(2023)
摘要
Brain-to-speech systems, which enable communication through neural activity, have gathered significant attention as potential neuroprosthesis for patients and as novel communication tools for broader individuals. To date, most non-invasive brain-to-speech research has focused on word-level decoding, while sentence-level reconstruction remains challenging. In this study, we introduce a sentence reconstruction method using a restricted range of 16 unique words and compare two different approaches: word-in-sentence reconstruction and natural sentence generation. The focus is on efficiently generating sentences by utilizing the temporal convolutional network model to extract features from EEG signals and create word embeddings that considers the contextual relevance between words. The language model and keyword density measuring are applied to evaluate the sentence reconstruction performance for each approach. The results show that the word-in-sentence approach with language model leads to a significant reduction in the word error rate of
$31.58\pm 18.58\ \%$
for spoken speech and
$56.01\pm 7.57\ \%$
for imagined speech. The natural sentence generation approach significantly improved the words per minute performance, enabling more natural mode of brain-to-speech. We conducted an online demo to verify the potential of the proposed approaches, generating audible speech from brain signals in real-time. These findings demonstrate the feasibility of natural brain-to-speech systems by considering the contextual relevance, allowing users to freely communicate natural sentences in real life.
更多查看译文
关键词
brain-to-speech,deep neural network,signal processing,brain-computer interface,electroencephalography,imagined speech,spoken speech
AI 理解论文
溯源树
样例
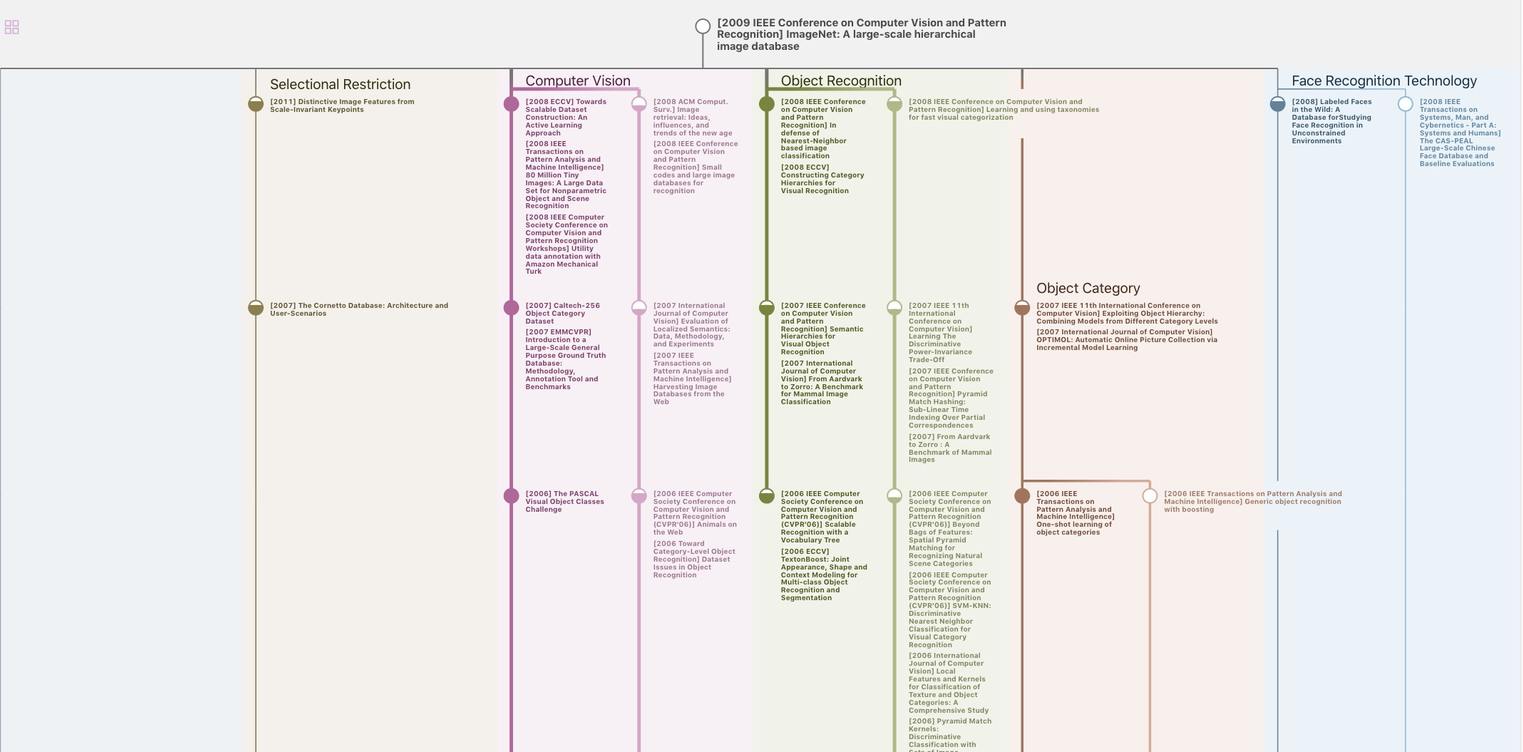
生成溯源树,研究论文发展脉络
Chat Paper
正在生成论文摘要