A Neighbor-Induced Graph Convolution Network for Undirected Weighted Network Representation.
2023 IEEE International Conference on Systems, Man, and Cybernetics (SMC)(2023)
摘要
Precise representation learning to an undirected weighted network (UWN) is the foundation of understanding its connection patterns and functional mechanisms. A graph convolution network (GCN) is frequently utilized to tackle this issue. However, it only considers the neighbor information in the forward propagation process, which unfortunately impairs its representation learning ability. Motivated by this discovery, this work proposes a novel Neighbor-induced Graph Convolution Network (N-GCN) that adopts two-fold ideas: a) employing the weighted forward propagation process, which aggregates the neighbor information by considering the interaction strength of node pair; b) incorporating a neighbor-regularizer into the loss function, which induces the neighbor information to illustrate a UWN's intrinsic symmetry, thereby boosting the representation learning ability. Experimental results on four UWNs validate the proposed N-GCN outperforms the state-of-the-art models in achieving a highly accurate representation of UWNs emerging from real applications.
更多查看译文
关键词
Undirected Weighted Network,Graph Convolutional Network,Neighbor-Induced,Weight Estimation
AI 理解论文
溯源树
样例
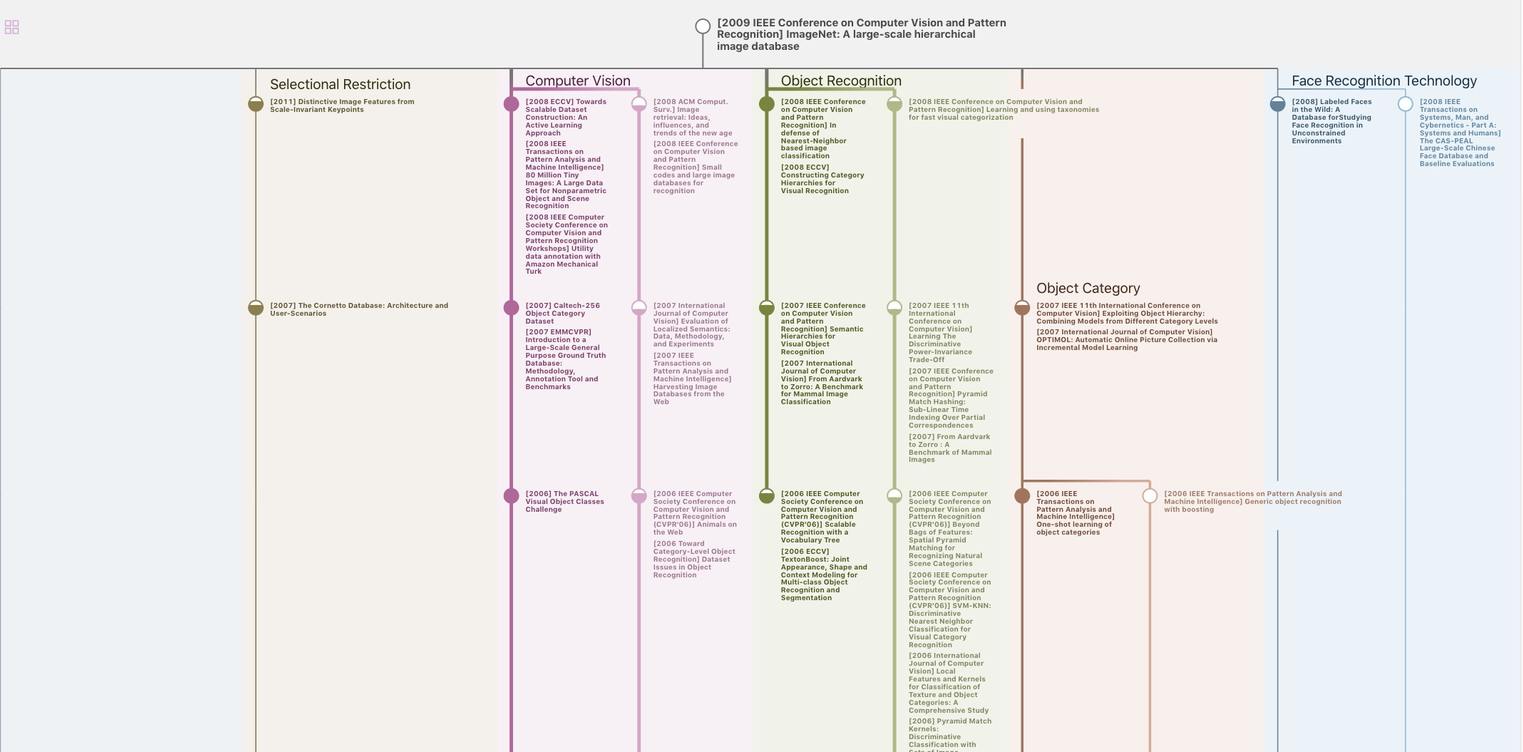
生成溯源树,研究论文发展脉络
Chat Paper
正在生成论文摘要