EgoFormer: Transformer-Based Motion Context Learning for Ego-Pose Estimation.
2023 IEEE International Conference on Systems, Man, and Cybernetics (SMC)(2023)
摘要
Ego-pose estimation, i.e. predicting 3D pose of the camera wearer, has an essential value in AR and VR applications. First-person video has an ambiguity in that similar video frames may correspond to totally different body poses because of the invisible body part. However, exploiting the context of a video and establishing a long-term temporal relationship can alleviate this ambiguity. To this end, this paper proposes EgoFormer, a Transformer-based model, to learn the motion context from egocentric videos. Moreover, dynamic features commonly used to characterize first-person video do not provide sufficient temporal information to remove the ambiguity inherent in such videos. Therefore, we present a method that can effectively extract temporal features in first-person videos. Results on real-scene and synthetic datasets show that our method could estimate a sequence of human poses with high accuracy and coherence.
更多查看译文
关键词
Motion Context,Ambiguity,Temporal Features,Video Frames,High Coherence,Video Features,Human Pose,3D Pose,Body Pose,Final Results,Sequence Length,Feature Representation,Local Coordinate,Redundant Information,Optical Flow,Transformer Model,Motion Features,Consecutive Frames,Video Sequences,Large Movements,Number Of Joints,Pose Estimation,Human Pose Estimation,Linear Layer,Adjacent Frames,Current Frame,Beginning Of Sequence,Decoding Stage,Reinforcement Learning Methods
AI 理解论文
溯源树
样例
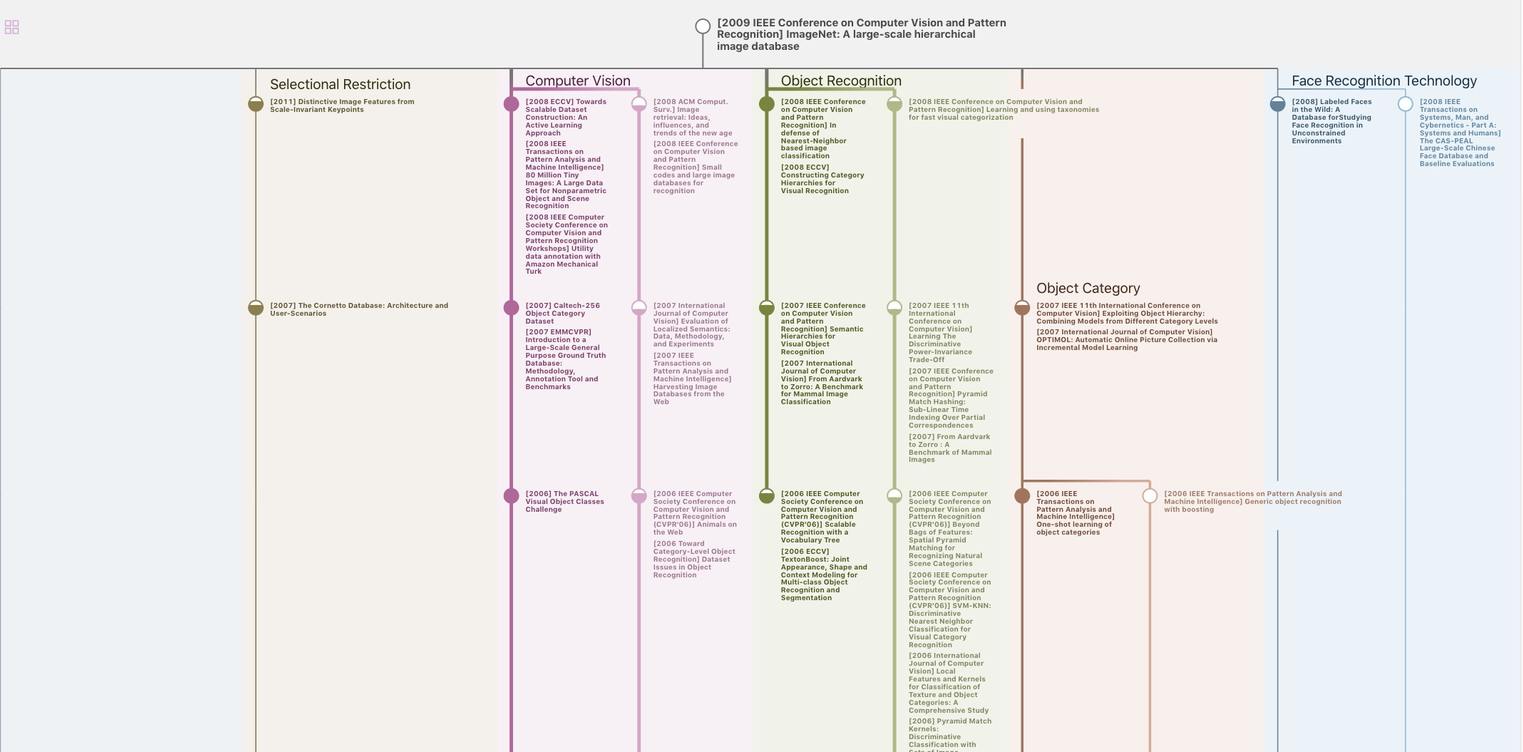
生成溯源树,研究论文发展脉络
Chat Paper
正在生成论文摘要