DA-MTAD: Capturing Intra- and Inter-Metric Dependencies for Multivariate Time Series Anomaly Detection.
2023 IEEE International Conference on Systems, Man, and Cybernetics (SMC)(2023)
摘要
Multivariate time series anomaly detection is a challenging task due to the intricate intra- and inter-metric dependencies present in the data. Previous methods have mainly focused on capturing intra-metric dependency, while neglecting the utilization of the dependency information across metrics. More recent approaches have considered inter-metric dependency, but have not been able to capture the complex inter-metric dynamics accurately and adaptively. To address these challenges, we propose a novel dual-attentional multivari-ate time series anomaly detection framework. Our approach improves on the original Dot-Product Attention to robustly capture intra-metric dependency. Additionally, we introduce automatic graph structure learning and graph attention mechanism to adaptively capture inter-metric dependency and utilize it effectively. Experiments on five public datasets from different domains demonstrate that comprehensive and accurate modeling of both dependencies enables our proposed method to outperform baseline methods in accurately detecting anomalies.
更多查看译文
关键词
Time Series,Anomaly Detection,Multivariate Time Series,Time Series Anomaly,Attention Mechanism,Learning Mechanisms,Graph Structure,Graph Attention,Automatic Learning,Neural Network,Values Of Variables,Convolutional Neural Network,F1 Score,Long Short-term Memory,Recurrent Neural Network,Nuclear Power Plant,Temporal Dependencies,Graph Neural Networks,Gated Recurrent Unit,Temporal Modulation,Anomaly Score,Isolation Forest,Cyber-physical Systems,Node Embeddings,Multivariate Time Series Data,Temporal Convolutional Network,Inductive Bias,Adversarial Training,Individual Metrics,Autoencoder
AI 理解论文
溯源树
样例
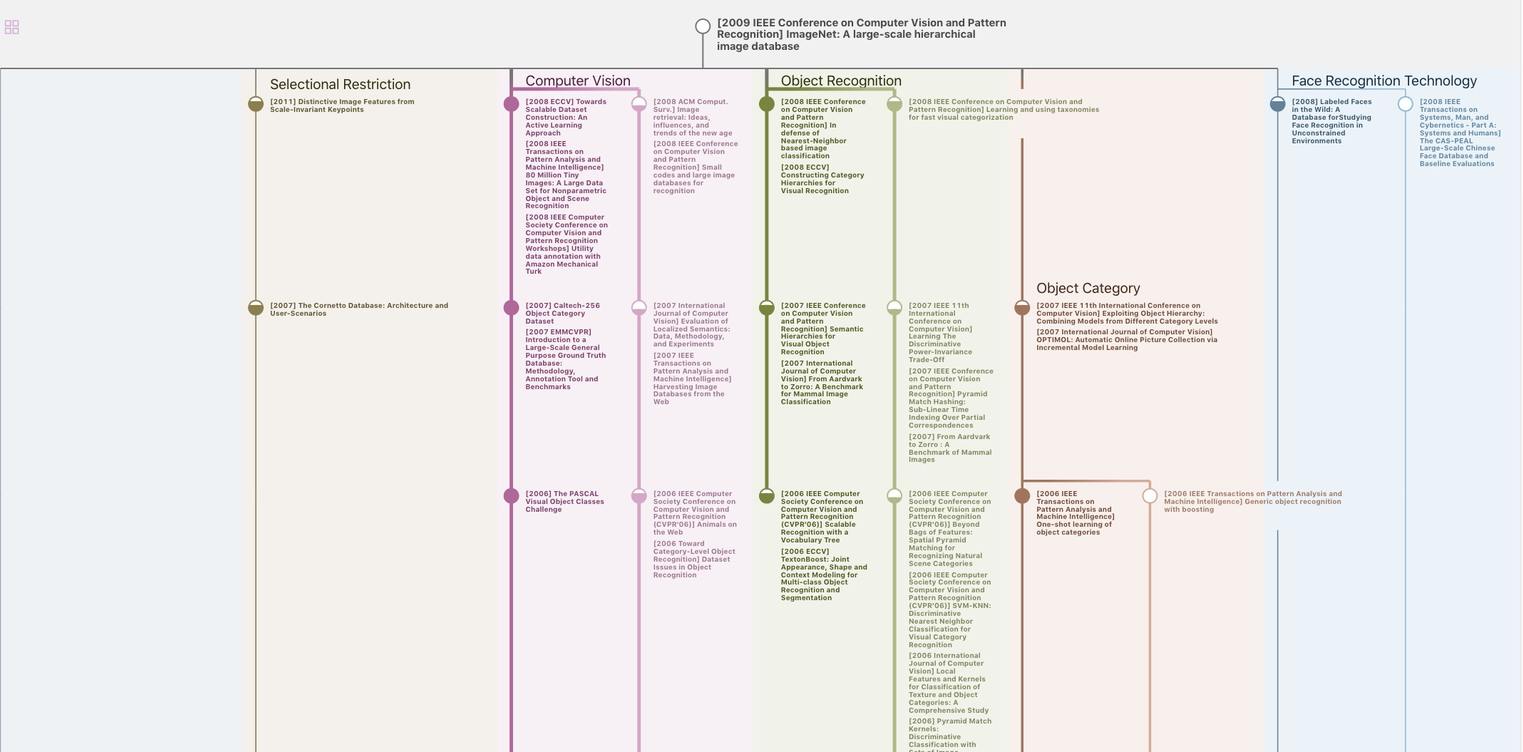
生成溯源树,研究论文发展脉络
Chat Paper
正在生成论文摘要