Short Calibrated SSVEP-BCI for Cross-Subject Transfer Learning via ELM-AE.
2023 IEEE International Conference on Systems, Man, and Cybernetics (SMC)(2023)
摘要
The Steady-State Visually Evoked Potential (SSVEP) is a robust paradigm used to build a high-speed Brain-Computer Interface (BCI). This technology can benefit disabled subjects, allowing them to interact with their surroundings without using their peripheral nerves. However, one challenge to address is reducing the time calibration of BCI for a new subject (target subject) because of the high brain EEG variability among subjects and within subjects in different sessions. This constraint restricts the application of SSVEP-based BCI in natural environments; thus, some approaches to endeavor this constraint propose a linear transformation of existing subjects over some trials of the target subject. In this paper, we propose an approach to a nonlinear transformation (NLT) using an Extreme Learning Machine Autoencoder (ELM-AE) of SSVEP trials to improve a cross-subject classification reducing the calibration time for the target subject. Our results reported that the recognition accuracy improved by 6.58% for all subjects using NLT. Also, these results exhibit the feasibility of NLT that using a few templates from the target subject can enhance the recognition accuracy over cross-subject classification without NLT.
更多查看译文
关键词
Brain-Computer Interface,ELM-AE,nonlinear transformation,SSVEP,Transfer Learning
AI 理解论文
溯源树
样例
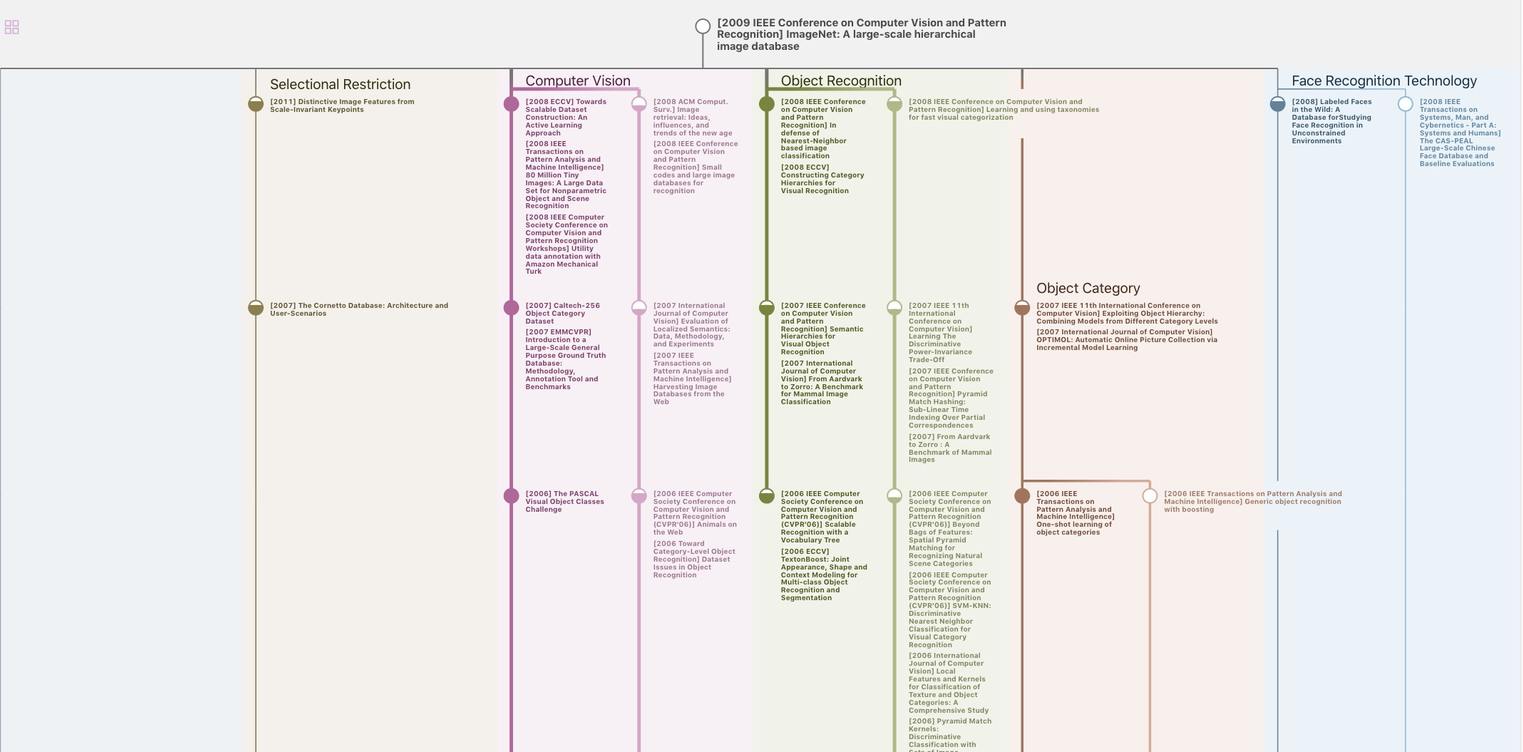
生成溯源树,研究论文发展脉络
Chat Paper
正在生成论文摘要