A Multi-Distance Feature Dissimilarity-Guided Encoder-Decoder Network for Polyp Segmentation.
2023 IEEE International Conference on Systems, Man, and Cybernetics (SMC)(2023)
摘要
Most colorectal cancers originate from adenomatous polyps, which start as single asymptomatic polyps and develop into malignant tumors. In clinical practice, colonoscopy is an extremely effective method for detecting polyps, and it provides important visual information for the accurate identification and removal of polyps. However, it is highly challenging to achieve accurate segmentation of various polyps due to the complex and variable size, shape, color, number, and growth background of polyps at different periods. To address these dilemmas, we propose a multi-distance feature dissimilarity-guided encoder-decoder network for automatic polyp segmentation, mainly consisting of the Multi-Distance Differential Module (MDDM) and the Hybrid Loss Module (HLM). The former mainly utilizes the multilayer feature subtraction (MLFS) operations to extract the difference information between short-distance adjacent layer features and short-distance and long-distance cross-layer features. Given this, the pyramid-inspired MDDM obtains discriminative features continuously between adjacent/cross layers, enhancing complementary features between different layers. The latter supervises the feature maps extracted at each network level to achieve finer predictions. Experiments on four challenge datasets confirm that the proposed model outperforms most state-of-the-art methods in six evaluation metrics while yielding reasonably accurate segmentation results.
更多查看译文
关键词
Polyp Segmentation,Colorectal Cancer,Feature Maps,Colonoscopy,Segmentation Results,Segmentation Accuracy,Automatic Segmentation,Subtraction Operation,Accurate Segmentation Results,Colon Cancer,Convolutional Neural Network,Sigmoid Function,Image Segmentation,Training Phase,Target Area,Receptive Field,Stochastic Gradient Descent,Segmentation Model,High-level Features,Low-level Features,Rich Contextual Information,Mean Intersection Over Union,Dice Similarity Coefficient,Pyramidal Layer,Adjacent Levels,Fully Convolutional Network,Generate Feature Maps,Weight Coefficient
AI 理解论文
溯源树
样例
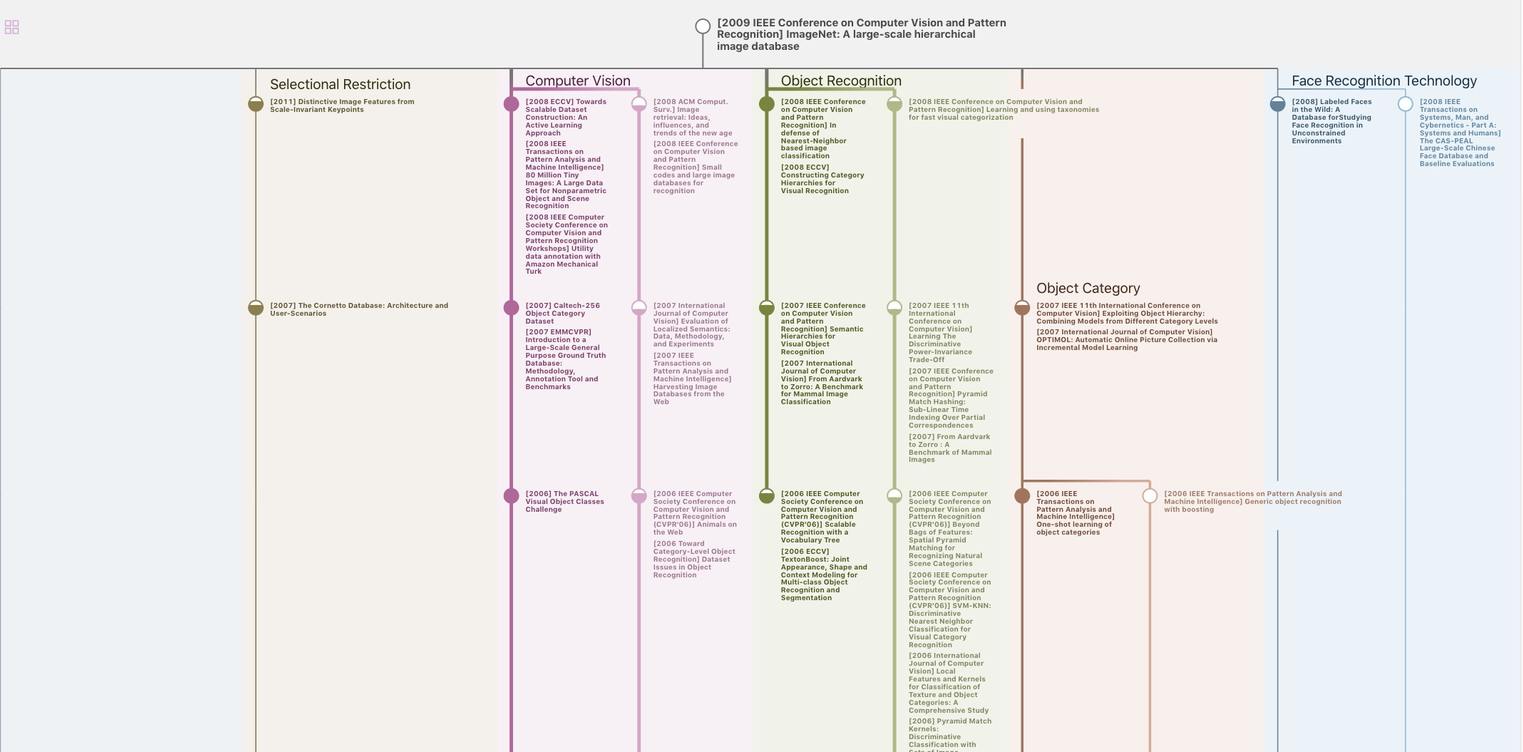
生成溯源树,研究论文发展脉络
Chat Paper
正在生成论文摘要