Stereo matching of binocular laparoscopic images with improved densely connected neural architecture search
International journal of computer assisted radiology and surgery(2024)
摘要
PurposeStereo matching is a crucial technology in the binocular laparoscopic-based surgical navigation systems. In recent years, neural networks have been widely applied to stereo matching and demonstrated outstanding performance. however, this method heavily relies on manual feature engineering meaning that professionals must be involved in the feature extraction and matching. This process is both time-consuming and demands specific expertise.MethodsThis paper introduces a novel stereo matching framework DCStereo that realizes a fully automatic neural architecture design for the stereo matching of binocular laparoscopic images. The proposed framework utilizes a densely connected search space which enables a more flexible and diverse architecture composition. Furthermore, the proposed algorithm leverages the channel and path sampling strategies to reduce memory consumption during searching.ResultsEmpirically, our searched DCStereo on the SCARED training dataset achieves a mean absolute error of 3.589 mm on the test dataset, which outperforms hand-crafted stereo matching methods and other approaches. Furthermore, when directly testing on the SERV-CT dataset, our DCStereo demonstrates better generalization ability than other methods.ConclusionOur proposed approach leverages the neural architecture search technique and a densely connected search space for automatic neural architecture design in stereo matching of binocular laparoscopic images. Our method delivers advanced performance on the SCARED dataset and promising results on the SERV-CT dataset. These findings demonstrate the potential of our approach for improving clinical surgical navigation systems.
更多查看译文
关键词
Binocular laparoscopic images,Stereo matching,Neural architecture search,Surgical navigation
AI 理解论文
溯源树
样例
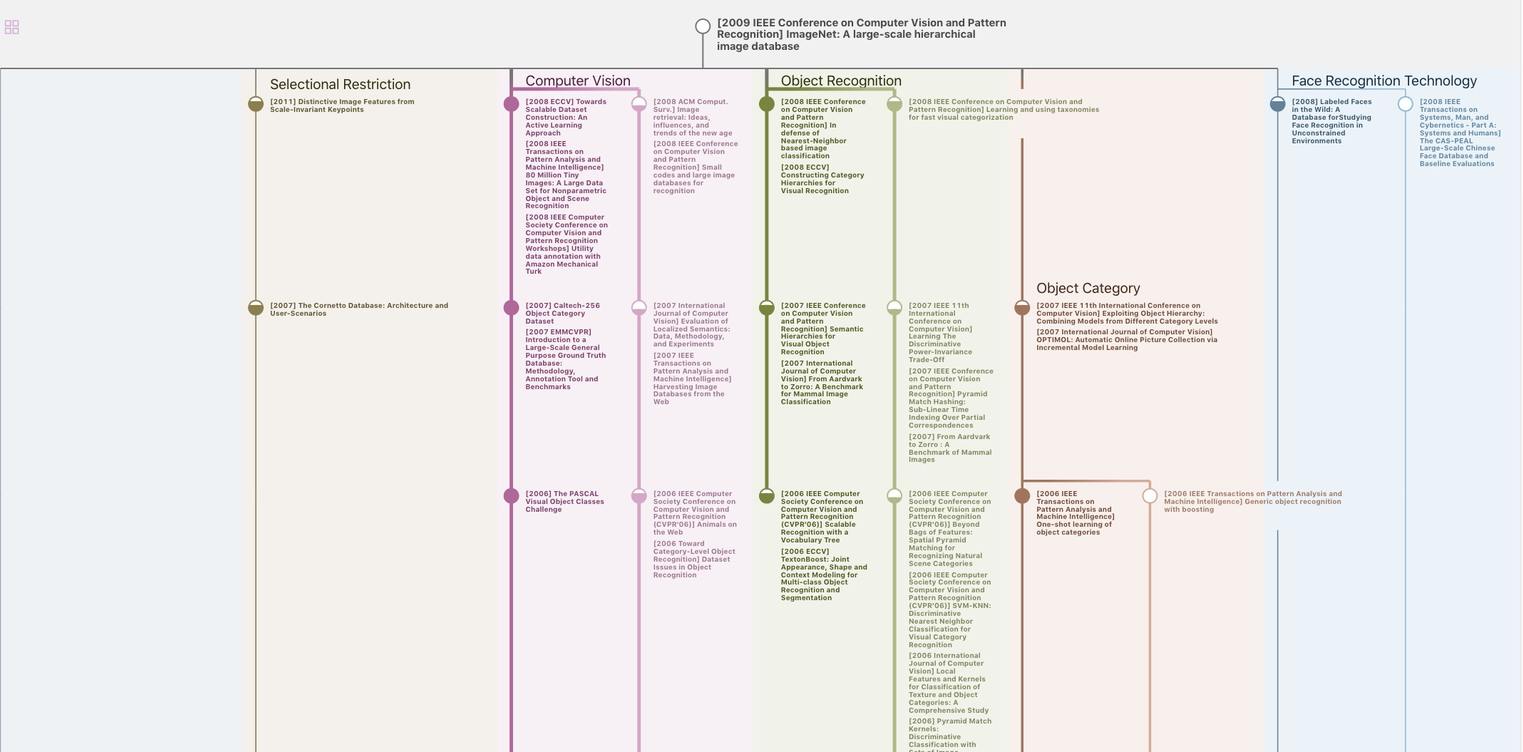
生成溯源树,研究论文发展脉络
Chat Paper
正在生成论文摘要