An analytic approach for understanding mechanisms driving breakthrough infections
arxiv(2024)
摘要
Real world data is an increasingly utilized resource for post-market
monitoring of vaccines and provides insight into real world effectiveness.
However, outside of the setting of a clinical trial, heterogeneous mechanisms
may drive observed breakthrough infection rates among vaccinated individuals;
for instance, waning vaccine-induced immunity as time passes and the emergence
of a new strain against which the vaccine has reduced protection. Analyses of
infection incidence rates are typically predicated on a presumed mechanism in
their choice of an "analytic time zero" after which infection rates are
modeled. In this work, we propose an explicit test for driving mechanism
situated in a standard Cox proportional hazards framework. We explore the
test's performance in simulation studies and in an illustrative application to
real world data. We additionally introduce subgroup differences in infection
incidence and evaluate the impact of time zero misspecification on bias and
coverage of model estimates. In this study we observe strong power and
controlled type I error of the test to detect the correct infection-driving
mechanism under various settings. Similar to previous studies, we find
mitigated bias and greater coverage of estimates when the analytic time zero is
correctly specified or accounted for.
更多查看译文
AI 理解论文
溯源树
样例
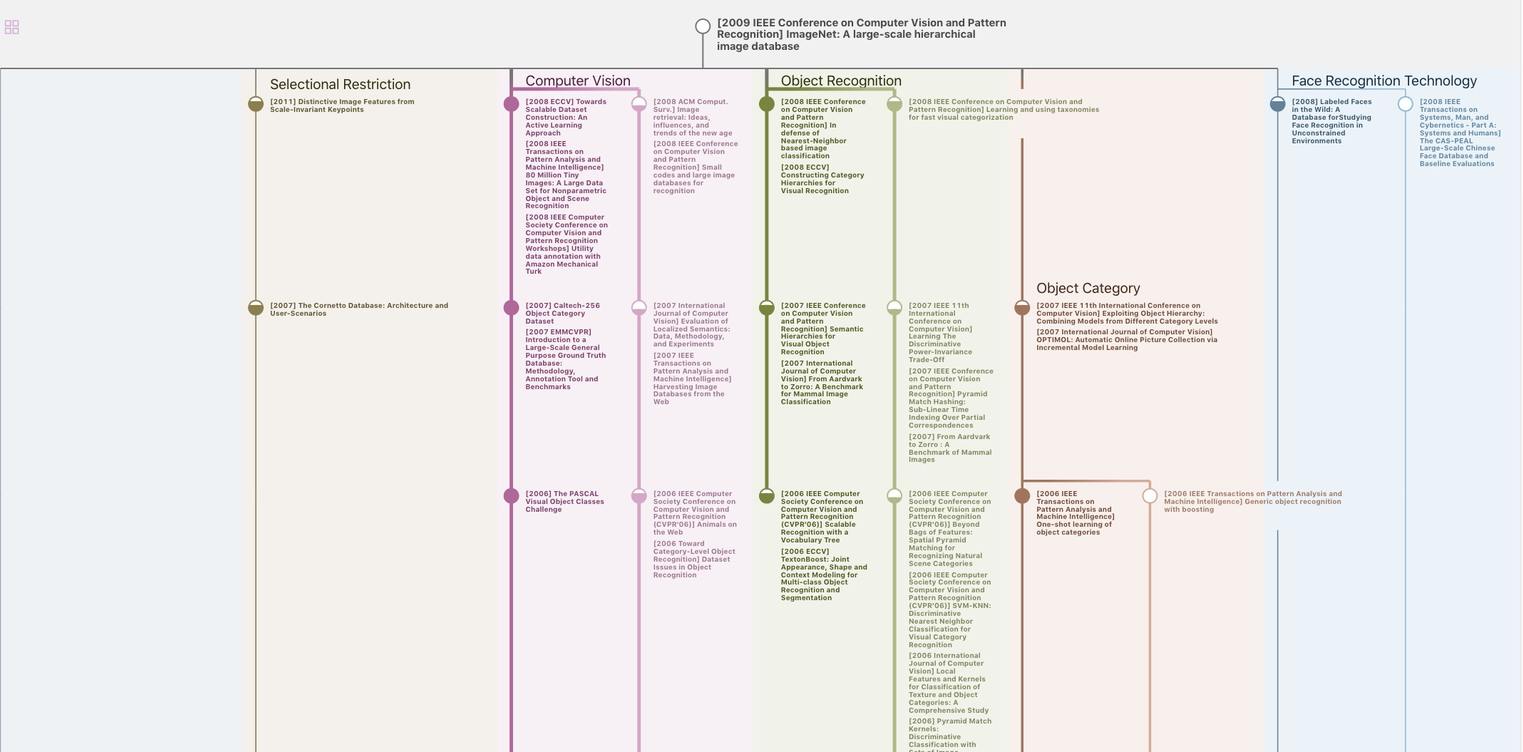
生成溯源树,研究论文发展脉络
Chat Paper
正在生成论文摘要