Investigating the Performance and Reliability, of the Q-Learning Algorithm in Various Unknown Environments
2023 11th RSI International Conference on Robotics and Mechatronics (ICRoM)(2023)
摘要
Self-reinforcement algorithms, especially Q-learning and value iteration have been popular and effective for navigating mobile robots. To provide a deeper insight through their performance and usage, this paper investigates solving a simple Q-Learning problem for various working environments for mobile robots. No matter how quick or reliable they are, all temporal difference algorithms employ iterative methods to produce desired results. For this reason, in this paper, we looked at the relationship between episode steps, mesh sizes, and-most importantly-the impact of the environment on both convergence rate and solution reliability. As previously indicated, the majority of the conclusions of this study about the relationship between computation cost and environment and also dependability can be transferred to more sophisticated temporal difference based algorithms because all methods are iterative. As many robotic applications for reinforcement learning have two or three-dimensional state space for path planning, knowing the approximate convergence episode count would be a useful tool for researchers to tackle non-optimal episodes, especially on embedded systems with computational constraints. The content also discussed the relationship between three mesh sizes and tried to guide researchers to implement their reinforcement learning pipeline more effectively. As shown by this work, the performance and reliability of iterative reinforcement learning algorithms depend deeply on the environment. In certain cases, increasing the number of episodes would not alter the results entirely. Therefore, when solving these kinds of maps, researchers would refrain from using Q-learning techniques.
更多查看译文
关键词
Reinforcement Learning,Q-Learning,Path Planning
AI 理解论文
溯源树
样例
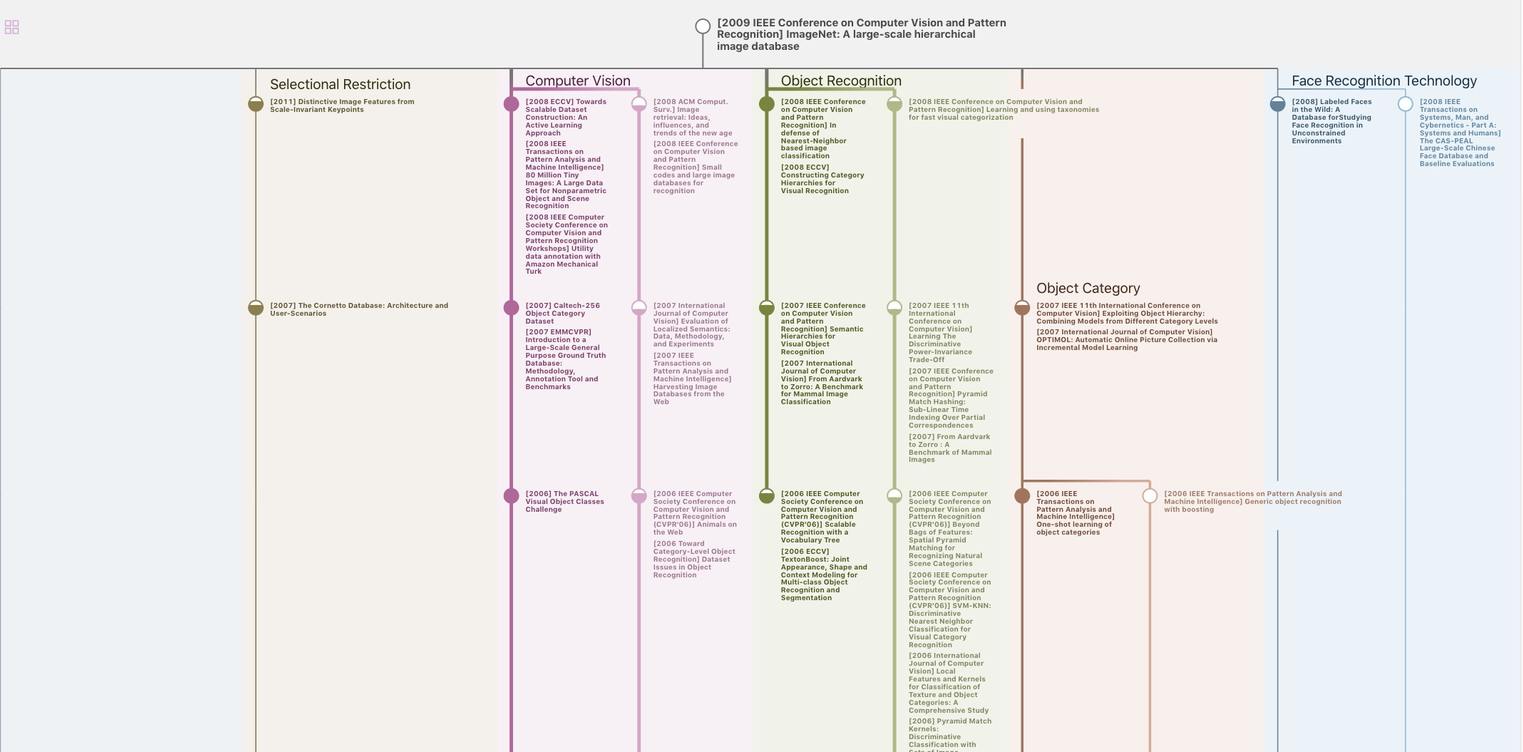
生成溯源树,研究论文发展脉络
Chat Paper
正在生成论文摘要