A Fundamental Discussion on Reward-Based Reinforcement Learning for Uplink Resource Allocation in NTN
2023 FOURTEENTH INTERNATIONAL CONFERENCE ON MOBILE COMPUTING AND UBIQUITOUS NETWORK, ICMU(2023)
摘要
The integrated usage of non-terrestrial network (NTN) nodes like high altitude platform (HAP), and low earth orbit (LEO) satellite plays a significant supplemental role in the upcoming sixth generation (6G) network to provide connectivity services for massive mobile users. Various network functions can be performed intelligently using potential artificial intelligence (AI) tools. Resilient and effective network channel accessibility and resource management, however, pose significant challenges due to the mobility, scarce resources, and complexity of integrated terrestrial networks (TNs) and NTNs, and massive mobile users on ground networks. Considering that many services demand realtime uplink (UL) data transmission, in this work, we consider the uplink of cooperated air-space segments (HAPs and LEO satellites) for large-sized data transmission where HAPs transmit data simultaneously to the LEO satellites through multiple subcarriers using non-orthogonal multiple access (NOMA) approach, and the LEO satellite receives the superimposed signal data from HAPs. To maximize the fair allocation of resources among HAPs while reflecting the LEO satellite's load and utility, the problem is formulated as nonlinear mixed-integer programming (MINLP). Given that NTN channels are dynamic, we advocate a novel deep reward-based multiple-agent reinforcement learning for dynamic channel allocation. Furthermore, to handle the short visibility of the LEO satellites in learning, we employ a prioritized experience reply (PER) through a priority sampling approach (PSA). Numerical results reveal that our proposed approach significantly outperforms learning-based and conventional baseline models.
更多查看译文
关键词
Deep reinforcement learning,fairness index,LEO,HAP,non-orthogonal multiple access (NOMA)
AI 理解论文
溯源树
样例
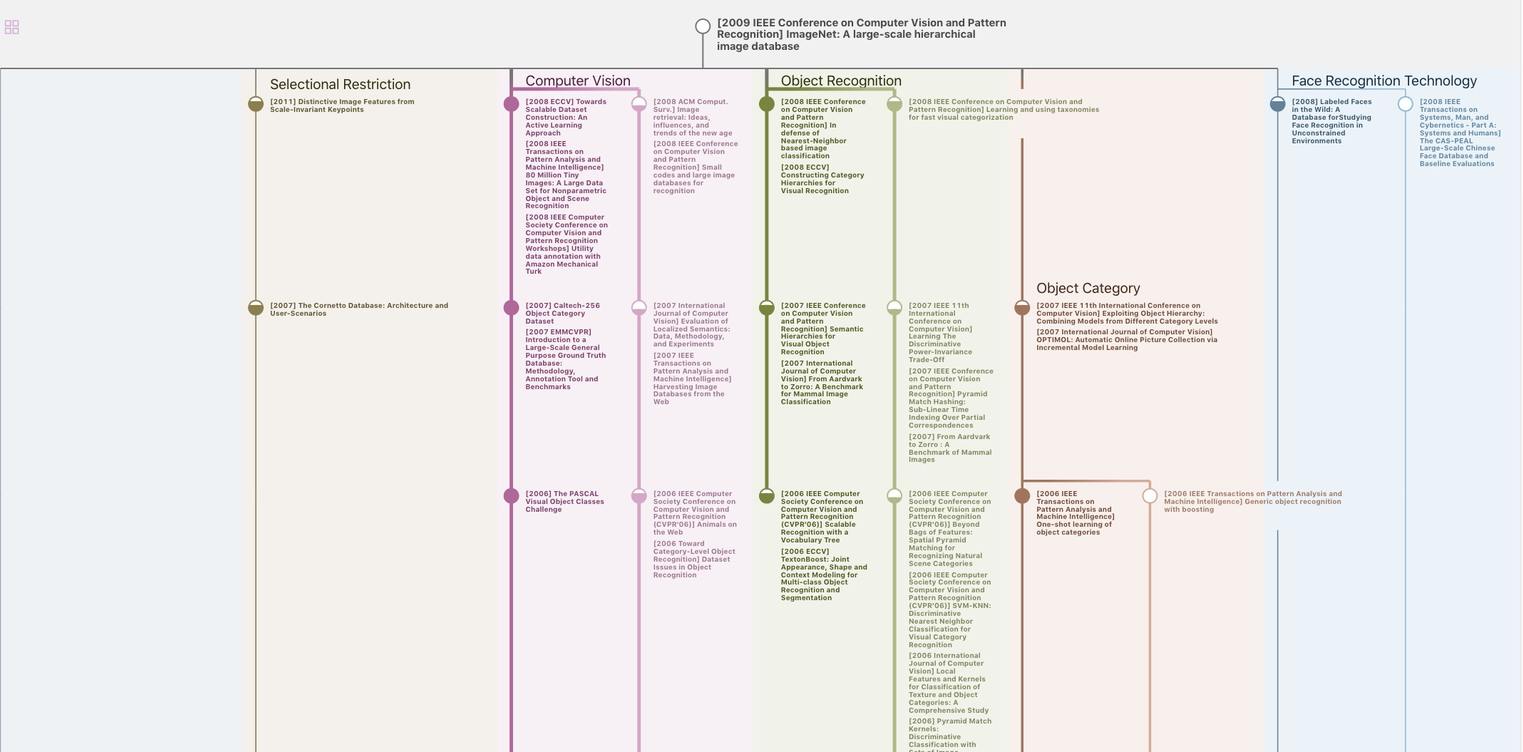
生成溯源树,研究论文发展脉络
Chat Paper
正在生成论文摘要