Comparative Analysis of Word vs. Character Embedding for Machine Learning Based Detection of Malicious URLs and DGA-Generated Domains
2023 IEEE 15th International Conference on Computational Intelligence and Communication Networks (CICN)(2023)
摘要
This study presents a comprehensive comparative analysis of the effectiveness of word-level and character-level embeddings in the context of machine learning-based detection of malicious URLs and DGA-generated domains. Utilizing distinct datasets comprising DGA-generated domains and Spam URLs, we systematically evaluate various machine learning models coupled with word-level and character-level tokenization techniques. Our findings indicate that character-level tokenization yields superior results in identifying DGA-generated domains, particularly due to the random character composition of these URLs. Conversely, both word-level and character-level embeddings exhibit comparable success rates in classifying Spam URLs, owing to the non-random nature of their URL structures. The study sheds light on the importance of tailoring tokenization strategies based on the unique characteristics of the data. We recommend character-level embeddings for detecting DGA-generated domains characterized by random characters. In contrast, the choice between word-level and character-level embeddings is less critical when dealing with Spam URLs, as both approaches yield effective results.
更多查看译文
关键词
Malicious URL detection,DGA domain detection,word embedding,character embedding,term frequency-inverse document frequency,n-grams,Bag-of-words,machine learning
AI 理解论文
溯源树
样例
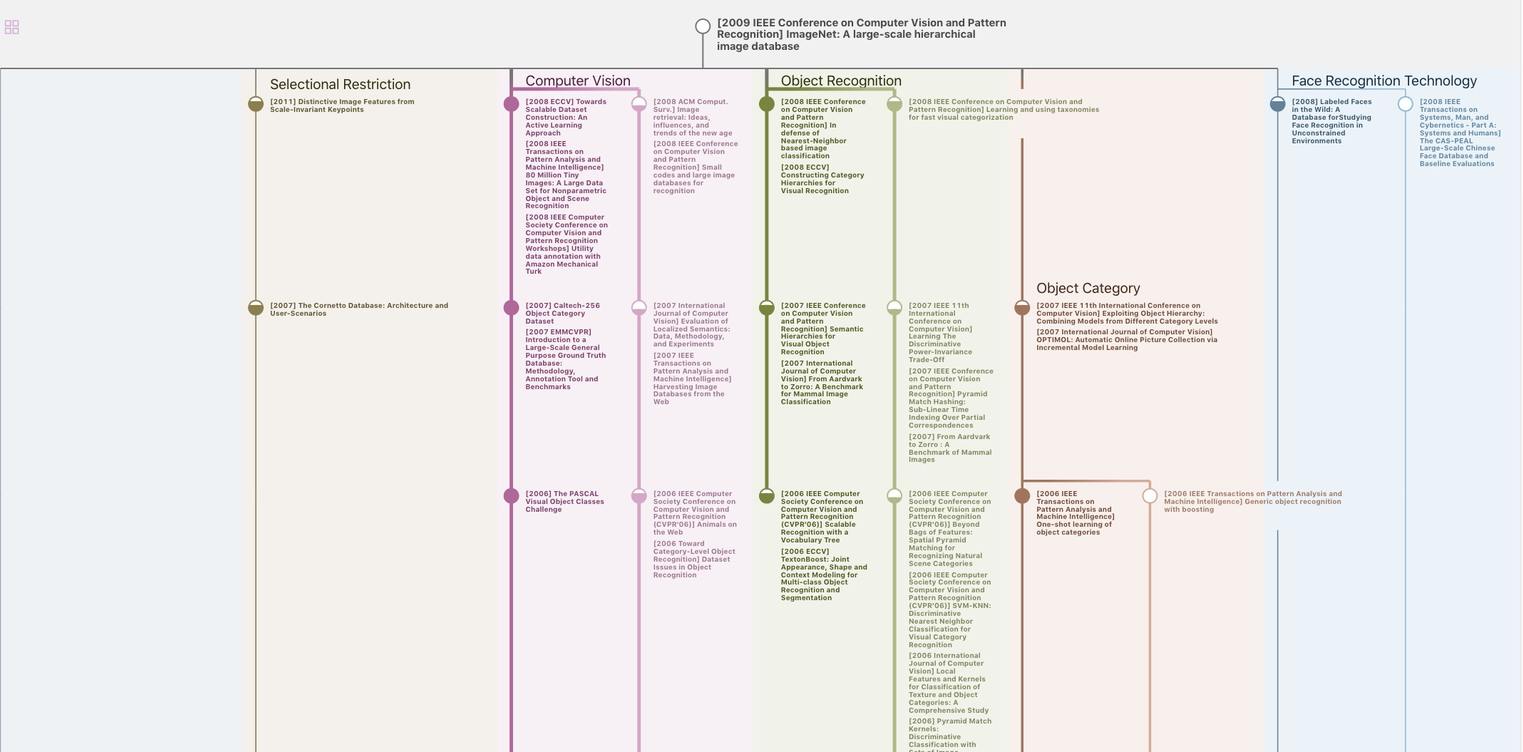
生成溯源树,研究论文发展脉络
Chat Paper
正在生成论文摘要