A hybrid approach of traffic simulation and machine learning techniques for enhancing real-time traffic prediction
TRANSPORTATION RESEARCH PART C-EMERGING TECHNOLOGIES(2024)
摘要
Accurate traffic prediction is important for efficient traffic operation, management, and user convenience. It enables traffic management authorities to allocate traffic resources efficiently, reducing traffic congestion and minimizing travel time for commuters. With the increase in data sources, traffic prediction methods have shifted from traditional model -based approaches to more data -driven methods. However, accurately predicting traffic under unforeseen events, such as crashes, adverse weather conditions, and road works, remains a challenging task. Hybrid traffic prediction models that combine data -driven and model -based approaches have emerged as promising solutions, considering the advantage of the model -based approach that can replicate unobserved scenarios. This paper proposes a hybrid traffic prediction framework named SMURP (Simulation and Machine -learning Utilization for Real-time Prediction), which overcomes the limitations of the existing methods. The SMURP is a framework that can be applied to any data -driven prediction method. When an event is detected during prediction, the SMURP complements the prediction outcomes with an additional predictor that uses simulated traffic data. The proposed framework is applied to various data -driven prediction models and evaluated in the actual road section. The results show that applying the SMURP to data -driven prediction methods can improve prediction accuracy.
更多查看译文
关键词
Real-time traffic prediction,Hybrid traffic prediction,Traffic simulation,Machine learning,Traffic prediction failure detection
AI 理解论文
溯源树
样例
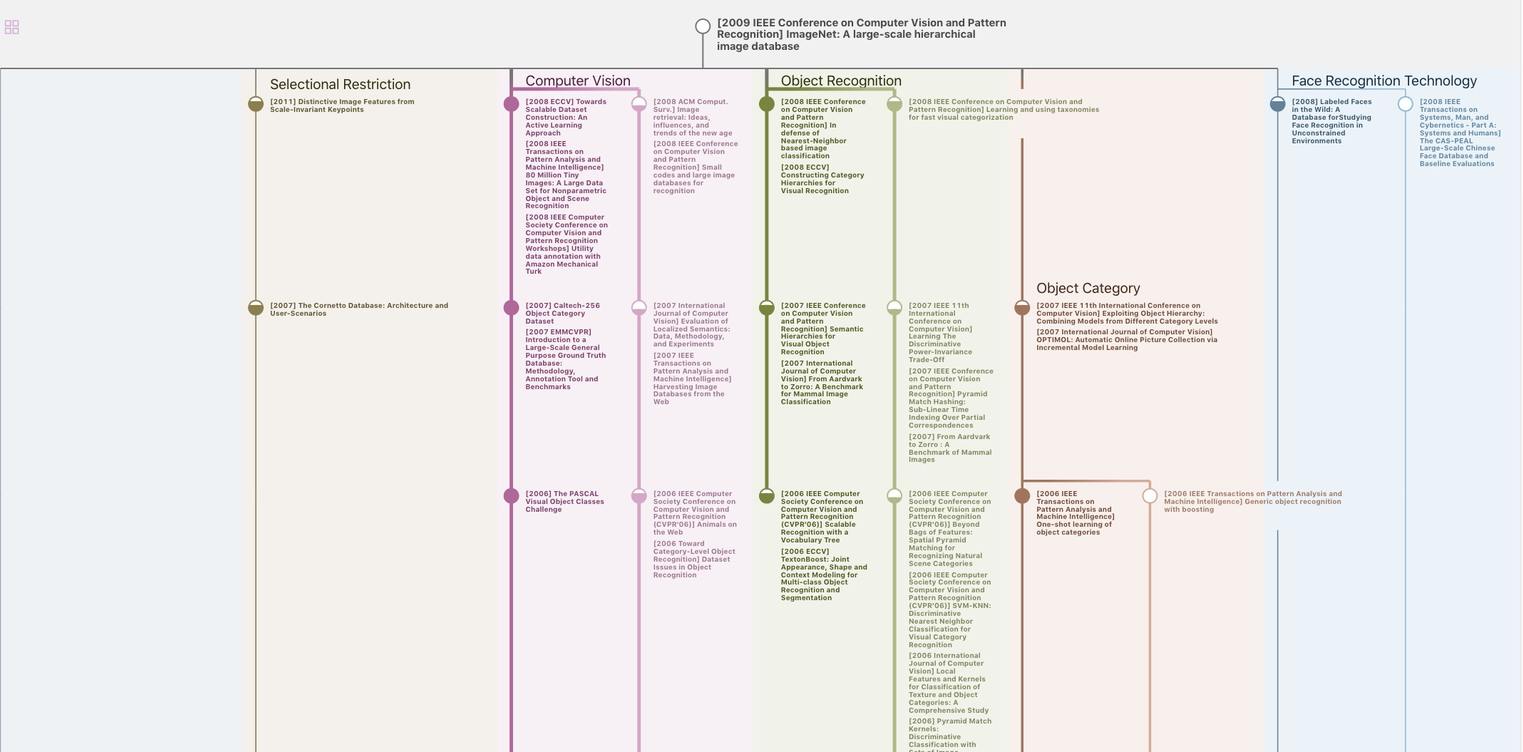
生成溯源树,研究论文发展脉络
Chat Paper
正在生成论文摘要