A deep-learning strategy to identify cell types across species from high-density extracellular recordings.
bioRxiv : the preprint server for biology(2024)
摘要
High-density probes allow electrophysiological recordings from many neurons simultaneously across entire brain circuits but fail to determine each recorded neuron's cell type. Here, we develop a strategy to identify cell types from extracellular recordings in awake animals, opening avenues to unveil the computational roles of neurons with distinct functional, molecular, and anatomical properties. We combine optogenetic activation and pharmacology using the cerebellum as a testbed to generate a curated ground-truth library of electrophysiological properties for Purkinje cells, molecular layer interneurons, Golgi cells, and mossy fibers. We train a semi-supervised deep-learning classifier that predicts cell types with greater than 95% accuracy based on waveform, discharge statistics, and layer of the recorded neuron. The classifier's predictions agree with expert classification on recordings using different probes, in different laboratories, from functionally distinct cerebellar regions, and across animal species. Our approach provides a general blueprint for cell-type identification from extracellular recordings across the brain.
更多查看译文
AI 理解论文
溯源树
样例
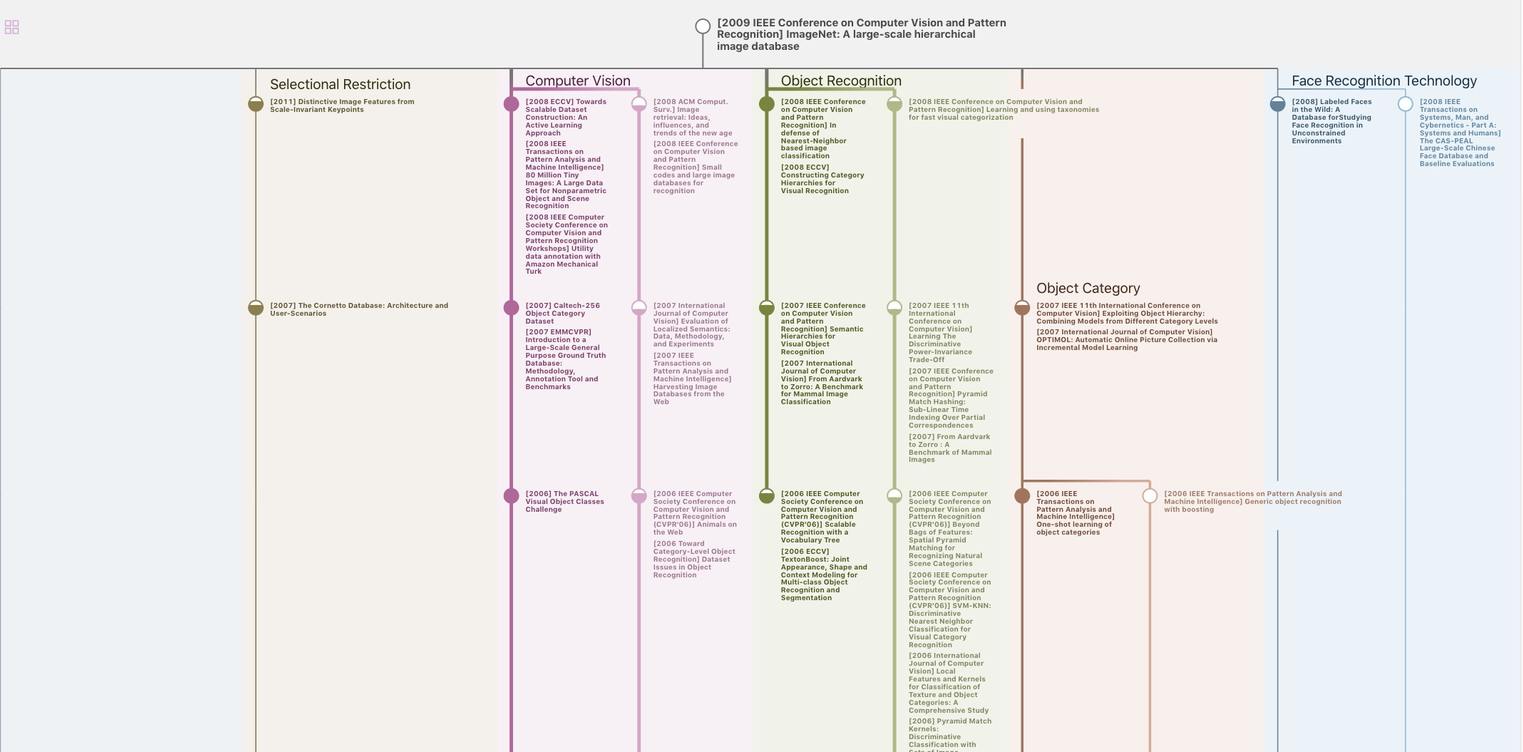
生成溯源树,研究论文发展脉络
Chat Paper
正在生成论文摘要