A Deep Dive Into Capsulorhexis Segmentation: From Dataset Creation to SAM Fine-tuning
2023 11th RSI International Conference on Robotics and Mechatronics (ICRoM)(2023)
摘要
Capsulorhexis is the most fateful phase of cataract surgery. The ability to automatically segment its main regions, and thereby, extract information can lead to image-guided surgery. Image-guided surgery has a wide range of applications that contribute to improved surgical outcomes and lower clinical risks. Despite the significant importance of capsulorhexis segmentation, a dedicated dataset focusing on this phase of cataract surgery is currently unavailable. This paper bridges this gap by creating a comprehensive dataset, which is named ARAS-CaSe, developed exclusively for capsulorhexis segmentation. ARAS-CaSe wide variety dataset is an invaluable resource for training computer vision models and achieving advancements in surgical skill assessment. Furthermore, certain state-of-the-art segmentation models were trained and fine-tuned on this dataset. Among the evaluated models, the SAM model had the highest level of performance, with an Intersection over Union (IoU) score of 91% and a Dice coefficient of 95.11%. To further analyze the models, we have performed a comparative analysis to find the efficient loss function for the U-Net segmentation model in the eye surgery domain. This study was conducted independently for each dataset class, and the presented results show that the Lovász-Softmax loss function will produce the best outcomes for capsulorhexis segmentation. ARAS-CaSe dataset, saved models, and codes of this research will be available by submitting a request through this website (aras.kntu. ac.ir/ai).
更多查看译文
关键词
Capsulorhexis,Cataract Surgery,Image Seg-mentation,Medical Imaging,Computer-Aided Surgery,Dataset
AI 理解论文
溯源树
样例
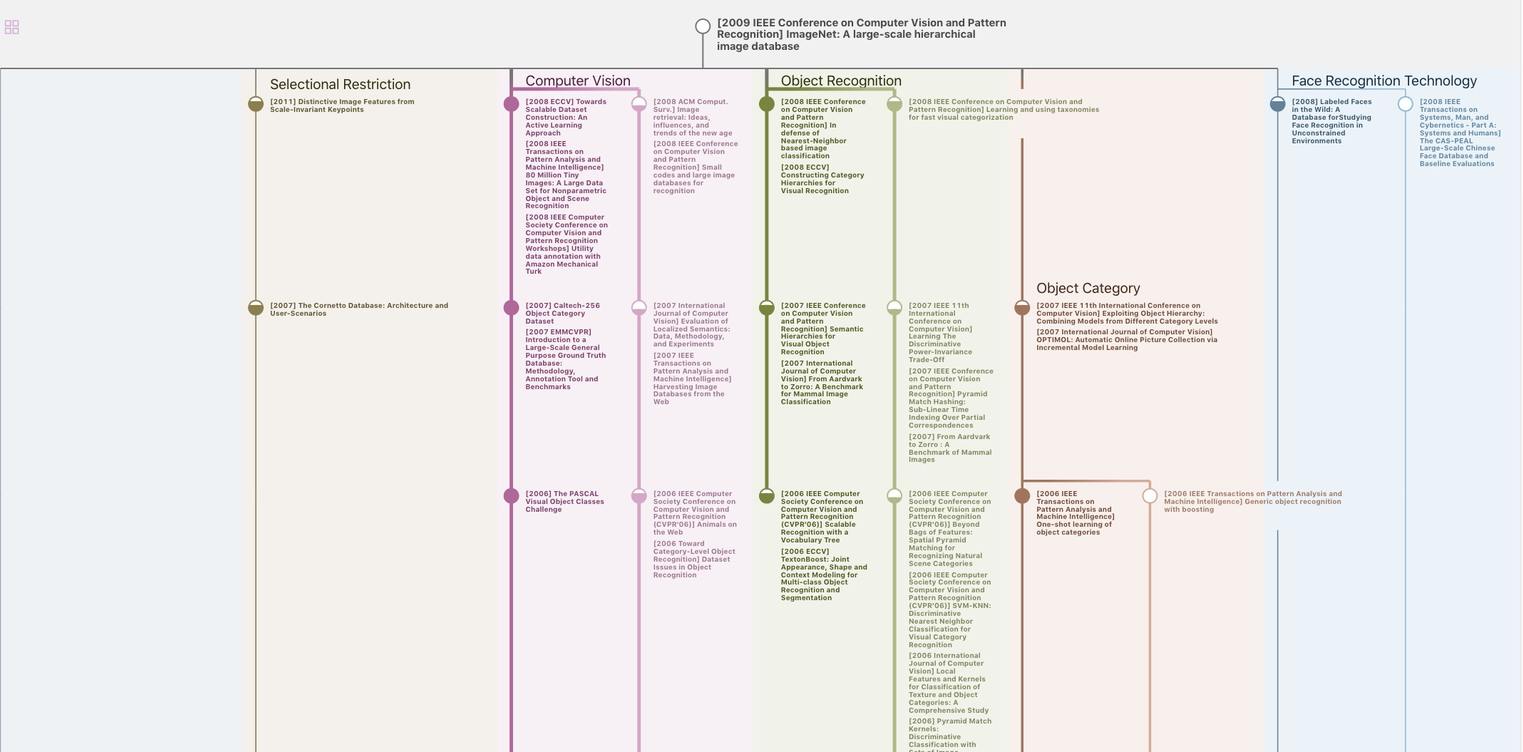
生成溯源树,研究论文发展脉络
Chat Paper
正在生成论文摘要