MCMC: Multi-Constrained Model Compression via One-Stage Envelope Reinforcement Learning
IEEE TRANSACTIONS ON NEURAL NETWORKS AND LEARNING SYSTEMS(2024)
摘要
Model compression methods are being developed to bridge the gap between the massive scale of neural networks and the limited hardware resources on edge devices. Since most real-world applications deployed on resource-limited hardware platforms typically have multiple hardware constraints simultaneously, most existing model compression approaches that only consider optimizing one single hardware objective are ineffective. In this article, we propose an automated pruning method called multi-constrained model compression (MCMC) that allows for the optimization of multiple hardware targets, such as latency, floating point operations (FLOPs), and memory usage, while minimizing the impact on accuracy. Specifically, we propose an improved multi-objective reinforcement learning (MORL) algorithm, the one-stage envelope deep deterministic policy gradient (DDPG) algorithm, to determine the pruning strategy for neural networks. Our improved one-stage envelope DDPG algorithm reduces exploration time and offers greater flexibility in adjusting target priorities, enhancing its suitability for pruning tasks. For instance, on the visual geometry group (VGG)-16 network, our method achieved an 80% reduction in FLOPs, a 2.31x reduction in memory usage, and a 1.92x acceleration, with an accuracy improvement of 0.09% compared with the baseline. For larger datasets, such as ImageNet, we reduced FLOPs by 50% for MobileNet-V1, resulting in a 4.7x faster speed and 1.48x memory compression, while maintaining the same accuracy. When applied to edge devices, such as JETSON XAVIER NX, our method resulted in a 71% reduction in FLOPs for MobileNet-V1, leading to a 1.63x faster speed, 1.64x memory compression, and an accuracy improvement.
更多查看译文
关键词
Hardware,Reinforcement learning,Optimization,Task analysis,Search problems,Mathematical models,Computational modeling,Deep neural networks (DNNs),model compression,multi-objective reinforcement learning (MORL),network pruning,Pareto frontier
AI 理解论文
溯源树
样例
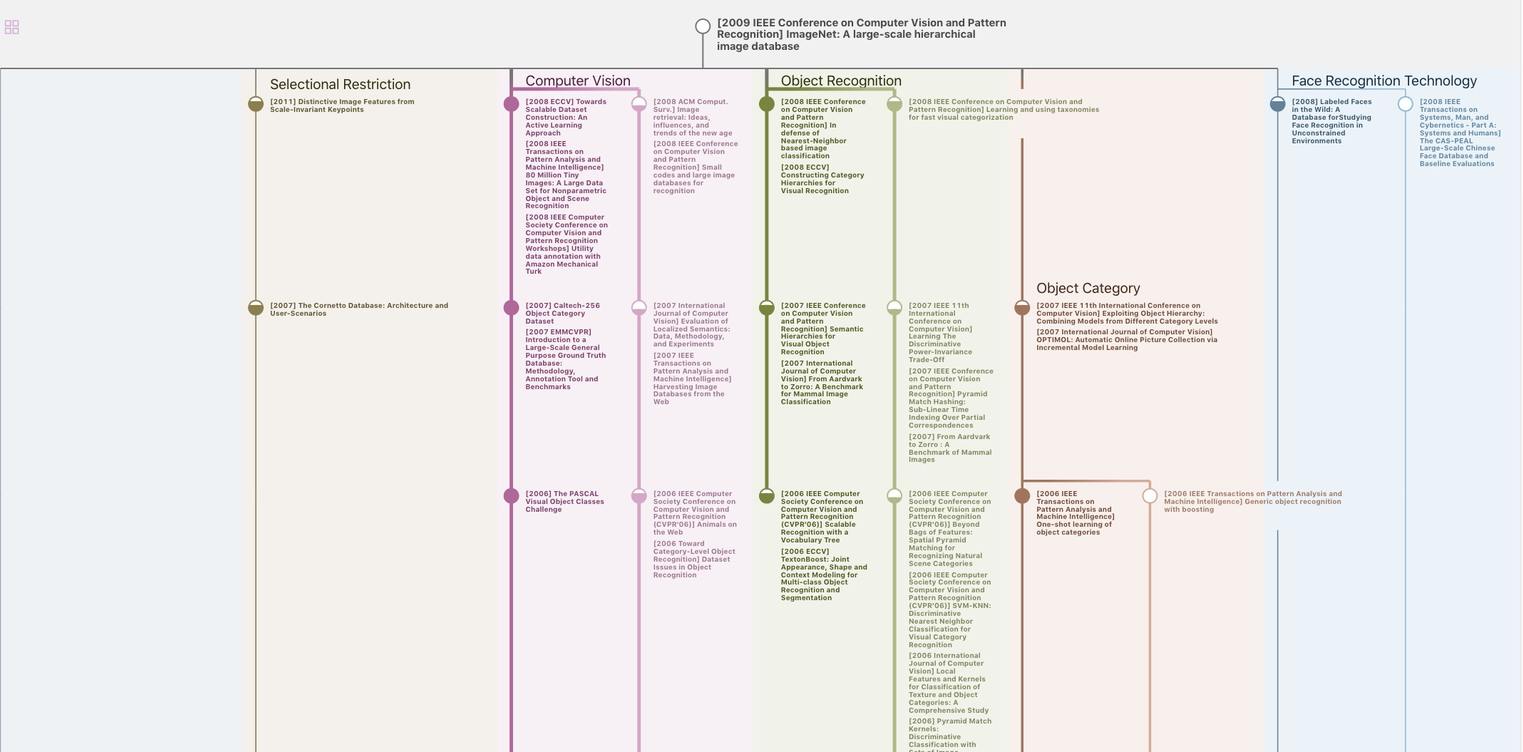
生成溯源树,研究论文发展脉络
Chat Paper
正在生成论文摘要