Automated Segmentation and Quantification of the Right Ventricle in 2-D Echocardiography
ULTRASOUND IN MEDICINE AND BIOLOGY(2024)
摘要
Objective: The right ventricle receives less attention than its left counterpart in echocardiography research, practice and development of automated solutions. In the work described here, we sought to determine that the deep learning methods for automated segmentation of the left ventricle in 2-D echocardiograms are also valid for the right ventricle. Additionally, here we describe and explore a keypoint detection approach to segmentation that guards against erratic behavior often displayed by segmentation models. Methods: We used a data set of echo images focused on the right ventricle from 250 participants to train and evaluate several deep learning models for segmentation and keypoint detection. We propose a compact architecture (UNet KP) employing the latter approach. The architecture is designed to balance high speed with accuracy and robustness. Results: All featured models achieved segmentation accuracy close to the inter -observer variability. When computing the metrics of right ventricular systolic function from contour predictions of U -Net KP, we obtained the bias and 95% limits of agreement of 0.8 +/- 10.8% for the right ventricular fractional area change measurements, -0.04 +/- 0.54 cm for the tricuspid annular plane systolic excursion measurements and 0.2 +/- 6.6% for the right ventricular free wall strain measurements. These results were also comparable to the semi-automatically derived inter -observer discrepancies of 0.4 +/- 11.8%, -0.37 +/- 0.58 cm and -1.0 +/- 7.7% for the aforementioned metrics, respectively. Conclusion: Given the appropriate data, automated segmentation and quantification of the right ventricle in 2-D echocardiography are feasible with existing methods. However, keypoint detection architectures may offer higher robustness and information density for the same computational cost.
更多查看译文
关键词
Deep learning,Echocardiography,Right ventricle,Segmentation,Ultrasound
AI 理解论文
溯源树
样例
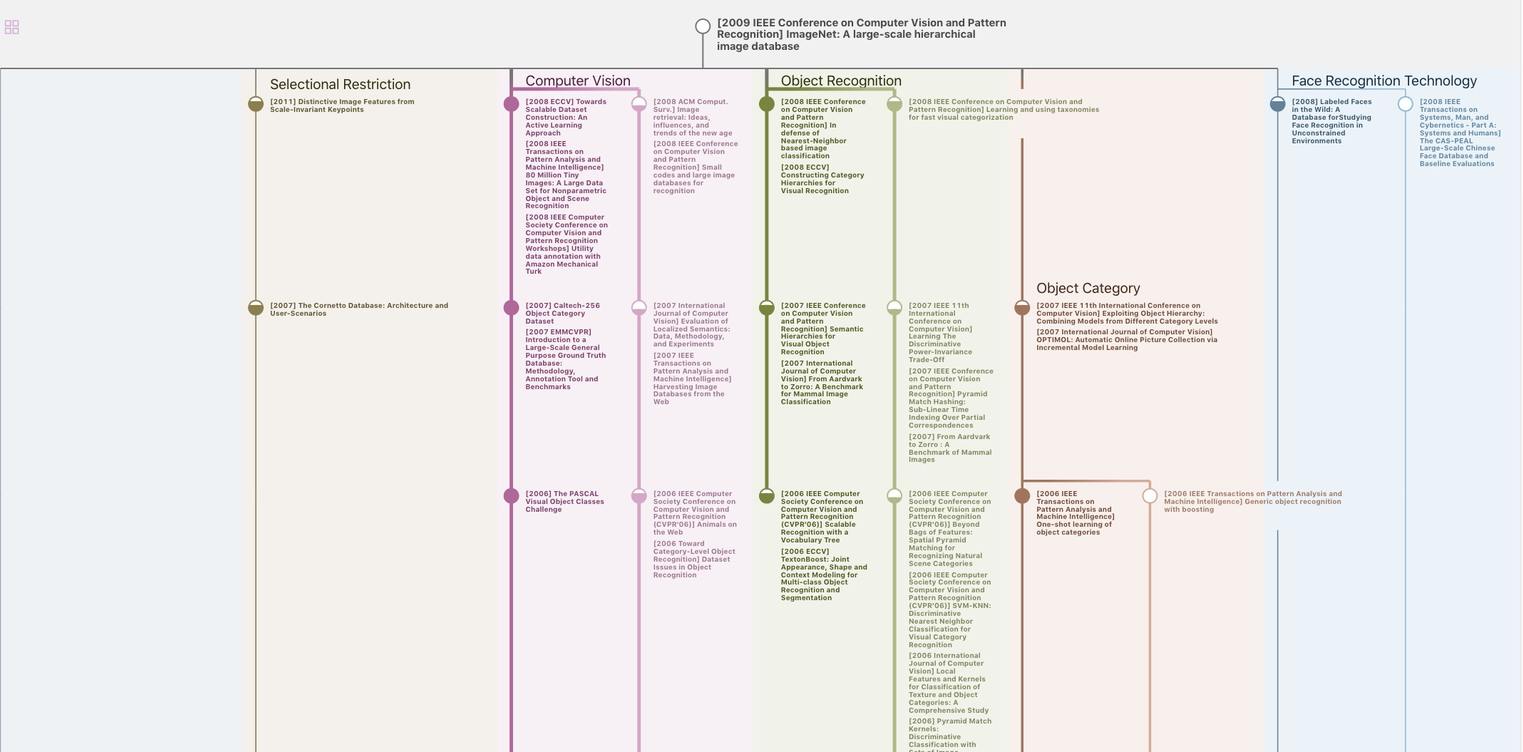
生成溯源树,研究论文发展脉络
Chat Paper
正在生成论文摘要