BERTE: High-precision hierarchical classification of transposable elements by a transfer learning method with BERT pre-trained model and convolutional neural network
biorxiv(2024)
摘要
Transposable Elements (TEs) are abundant repeat sequences found in living organisms. They play a pivotal role in biological evolution and gene regulation and are intimately linked to human diseases. Existing TE classification tools can classify classes, orders, and superfamilies concurrently, but they often struggle to effectively extract sequence features. This limitation frequently results in subpar classification results, especially in hierarchical classification. To tackle this problem, we introduced BERTE, a tool for TE hierarchical classification. BERTE encoded TE sequences into distinctive features that consisted of both attentional and cumulative k-mer frequency information. By leveraging the multi-head self-attention mechanism of the pre-trained BERT model, BERTE transformed sequences into attentional features. Additionally, we calculated multiple k-mer frequency vectors and concatenate them to form cumulative features. Following feature extraction, a parallel Convolutional Neural Network (CNN) model was employed as an efficient sequence classifier, capitalizing on its capability for high-dimensional feature transformation. We evaluated BERTE’s performance on filtered datasets collected from 12 eukaryotic databases. Experimental results demonstrated that BERTE could improve the F1-score at different levels by up to 21% compared to current state-of-the-art methods. Furthermore, the results indicated that not only could BERT better characterize TE sequences in feature extraction, but also that CNN was more efficient than other popular deep learning classifiers. In general, BERTE classifies TE sequences with greater precision. BERTE is available at .
### Competing Interest Statement
The authors have declared no competing interest.
更多查看译文
AI 理解论文
溯源树
样例
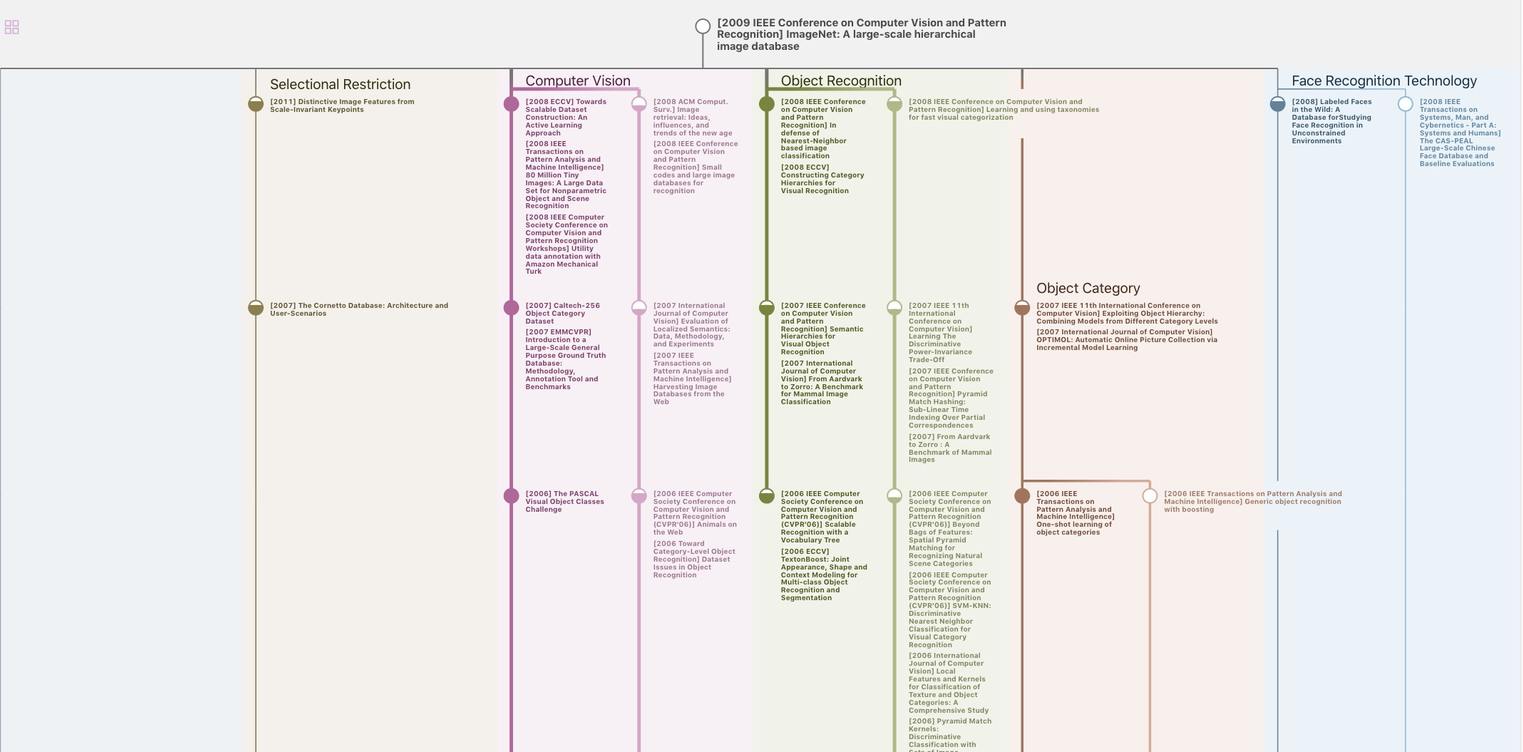
生成溯源树,研究论文发展脉络
Chat Paper
正在生成论文摘要