Protein language models learn evolutionary statistics of interacting sequence motifs
biorxiv(2024)
摘要
Protein language models (pLMs) have emerged as potent tools for predicting and designing protein structure and function, and the degree to which these models fundamentally understand the inherent biophysics of protein structure stands as an open question. Motivated by a discovery that pLM-based structure predictors erroneously predict non-physical structures for protein isoforms, we investigated the nature of sequence context needed for contact predictions in the pLM ESM-2. We demonstrate by use of a "categorical Jacobian" calculation that ESM-2 stores statistics of coevolving residues, analogously to simpler modelling approaches like Markov Random Fields and Multivariate Gaussian models. We further investigated how ESM-2 "stores" information needed to predict contacts by comparing sequence masking strategies, and found that providing local windows of sequence information allowed ESM-2 to best recover predicted contacts. This suggests that pLMs predict contacts by storing motifs of pairwise contacts. Our investigation highlights the limitations of current pLMs and underscores the importance of understanding the underlying mechanisms of these models.
### Competing Interest Statement
The authors have declared no competing interest.
更多查看译文
AI 理解论文
溯源树
样例
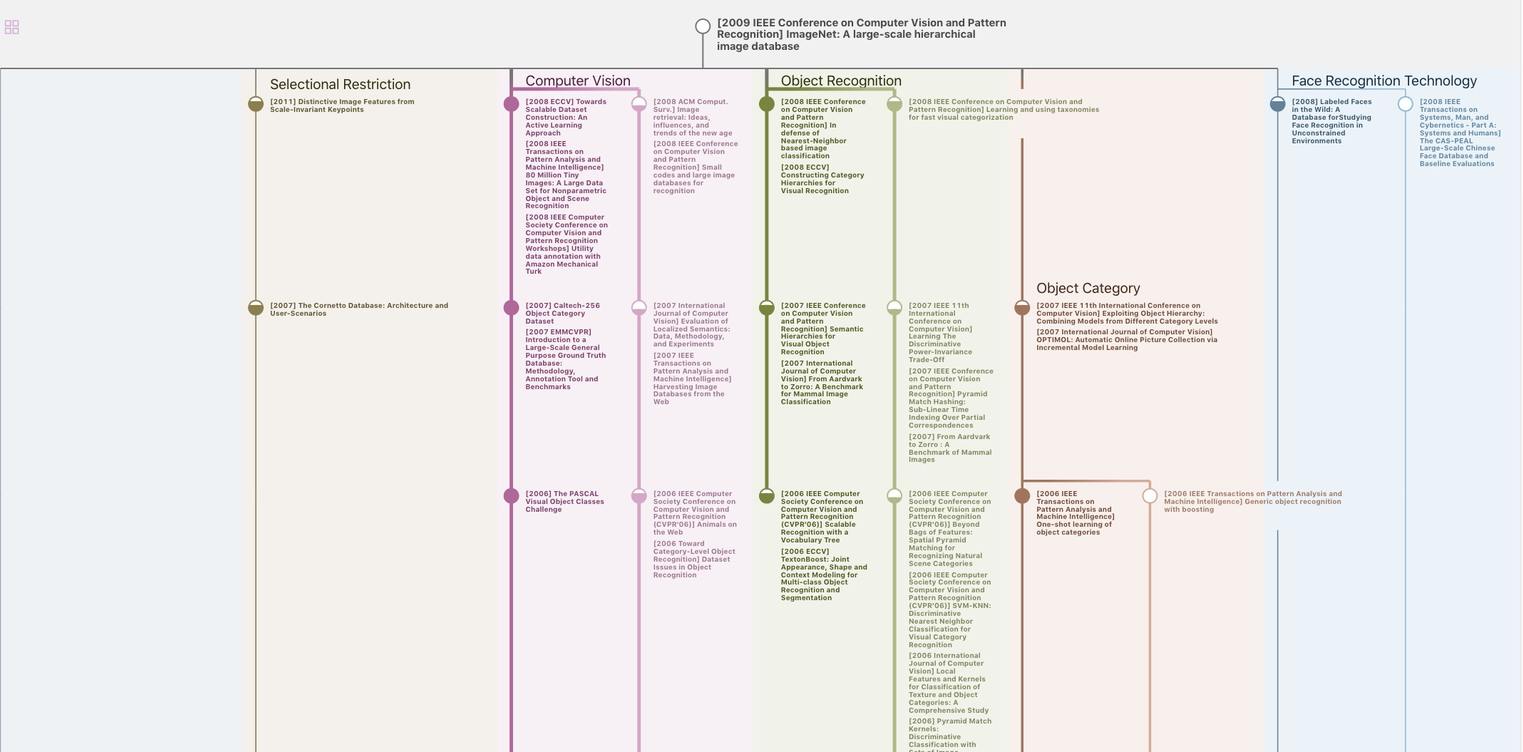
生成溯源树,研究论文发展脉络
Chat Paper
正在生成论文摘要