Kernel Probabilistic Dependent-Independent Canonical Correlation Analysis
INTERNATIONAL JOURNAL OF INTELLIGENT SYSTEMS(2024)
摘要
There is growing interest in developing linear/nonlinear feature fusion methods that fuse the elicited features from two different sources of information for achieving a higher recognition rate. In this regard, canonical correlation analysis (CCA), cross-modal factor analysis, and probabilistic CCA (PCCA) have been introduced to better deal with data variability and uncertainty. In our previous research, we formerly developed the kernel version of PCCA (KPCCA) to capture both nonlinear and probabilistic relation between the features of two different source signals. However, KPCCA is only able to estimate latent variables, which are statistically correlated between the features of two independent modalities. To overcome this drawback, we propose a kernel version of the probabilistic dependent-independent CCA (PDICCA) method to capture the nonlinear relation between both dependent and independent latent variables. We have compared the proposed method to PDICCA, CCA, KCCA, cross-modal factor analysis (CFA), and kernel CFA methods over the eNTERFACE and RML datasets for audio-visual emotion recognition and the M2VTS dataset for audio-visual speech recognition. Empirical results on the three datasets indicate the superiority of both the PDICCA and Kernel PDICCA methods to their counterparts.
更多查看译文
AI 理解论文
溯源树
样例
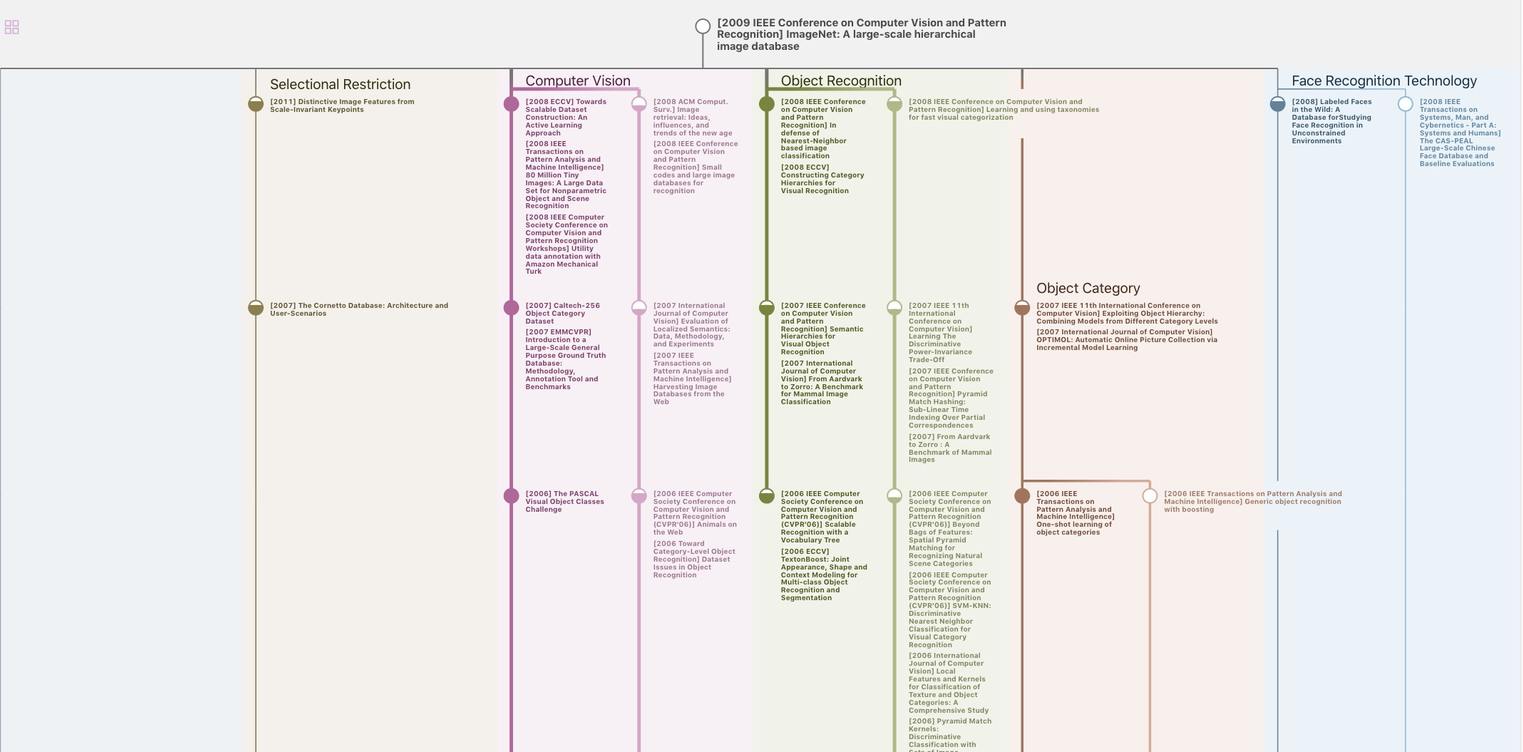
生成溯源树,研究论文发展脉络
Chat Paper
正在生成论文摘要