A robust combined weighted label fusion in multi-atlas pancreas segmentation
Multimedia Tools and Applications(2024)
摘要
Multi-atlas segmentation frameworks have proved to be a top-method as its good performance in medical image segmentation, which mainly consists of image registration and label fusion, namely, first register multiple atlases to target image, then fuse corresponding transformed labels into a final segmentation. However, previously weighted label fusion technique only uses the similarity between atlas image and target image, and the prior information of label image is directly ignored. In this work, we proposed a novel robust combined weighted label fusion model to alleviate the underutilization of atlas prior information in the label fusion stage. The features of this robust model are as follows:①a method for obtaining the segmentation object region (SOR) intensity images from atlas and target image is to calculate each atlas weight, which can more accurately express the importance and contribution of each atlas in the fusion process. ②a correlation of label images involved in fusion is firstly incorporated into label fusion as a weight, which makes up for the unused label prior information in the previous label fusion algorithm. ③a novel weight effectively combines the weight based on SOR similarity between atlas image and target image and the weight based on the label similarity among label images. The proposed model is evaluated on the NIH pancreas dataset and outperforms the classical method by achieving the mean ± std of 84.07
更多查看译文
关键词
Pancreas segmentation,Multi-atlas,Label fusion,Combined weighting
AI 理解论文
溯源树
样例
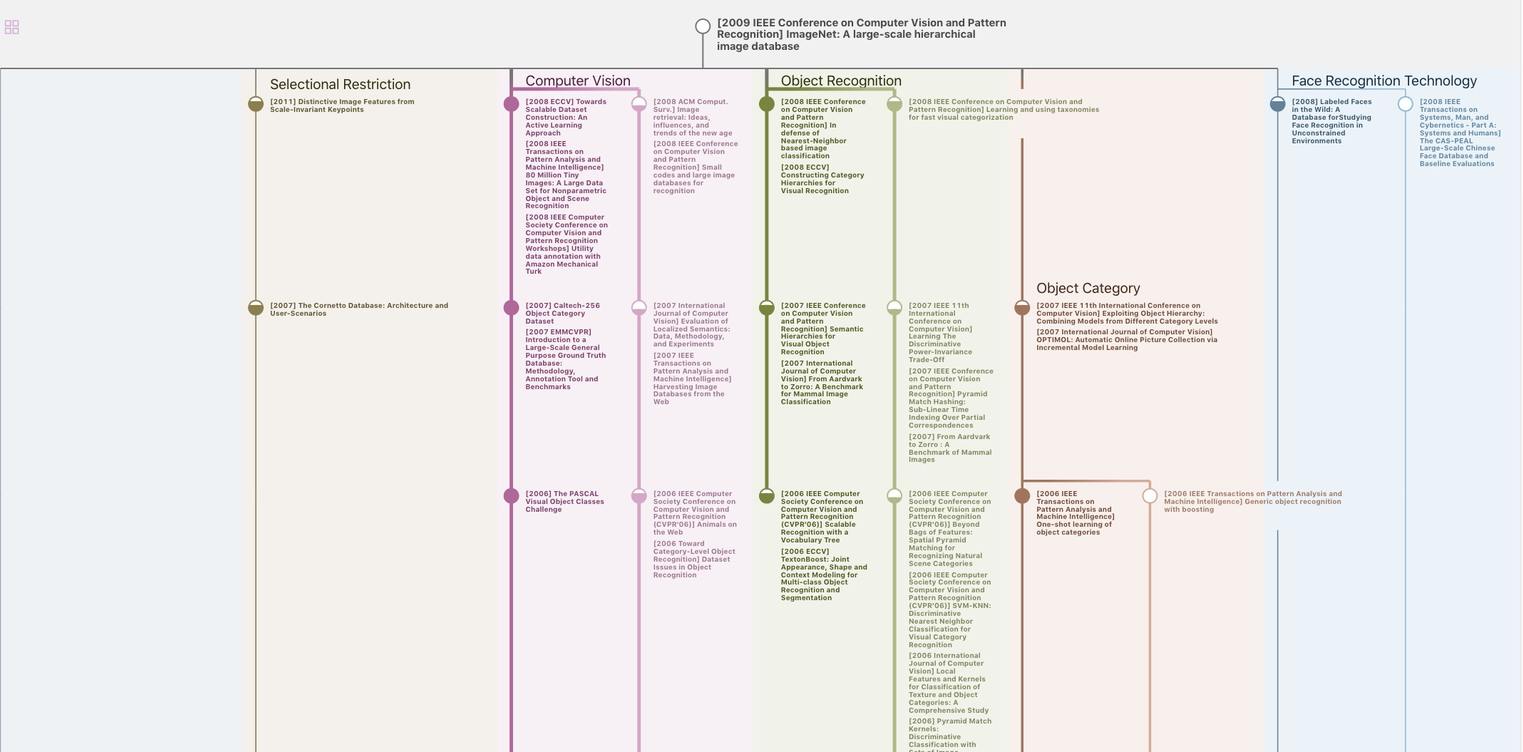
生成溯源树,研究论文发展脉络
Chat Paper
正在生成论文摘要