A sequential monte carlo method for parameter estimation in nonlinear stochastic pde's with periodic boundary conditions
2023 IEEE 9TH INTERNATIONAL WORKSHOP ON COMPUTATIONAL ADVANCES IN MULTI-SENSOR ADAPTIVE PROCESSING, CAMSAP(2023)
摘要
We tackle the problem of Bayesian inference for stochastic partial differential equations (SPDEs) with unknown parameters. We assume that the signal of interest can only be observed partially, possibly subject to some transformation, and contaminated by noise. For all practical purposes involving numerical computation, the SPDE has to be discretised using a numerical scheme that depends itself on an additional set of parameters (e.g., the number of coefficients and the time step for a spectral decomposition method). Within this setup, we address the Bayesian estimation of the complete parameter set, including both the SPDE parameters and the numerical scheme parameters, using a nested particle filter. A simple version of the proposed methodology is described and numerically demonstrated for a Kuramoto-Sivashinsky SPDE with periodic boundary conditions and a Fourier spectral-decomposition numerical scheme.
更多查看译文
关键词
Stochastic partial differential equations,Bayesian inference,particle filtering,Kuramoto-Sivashinsky.
AI 理解论文
溯源树
样例
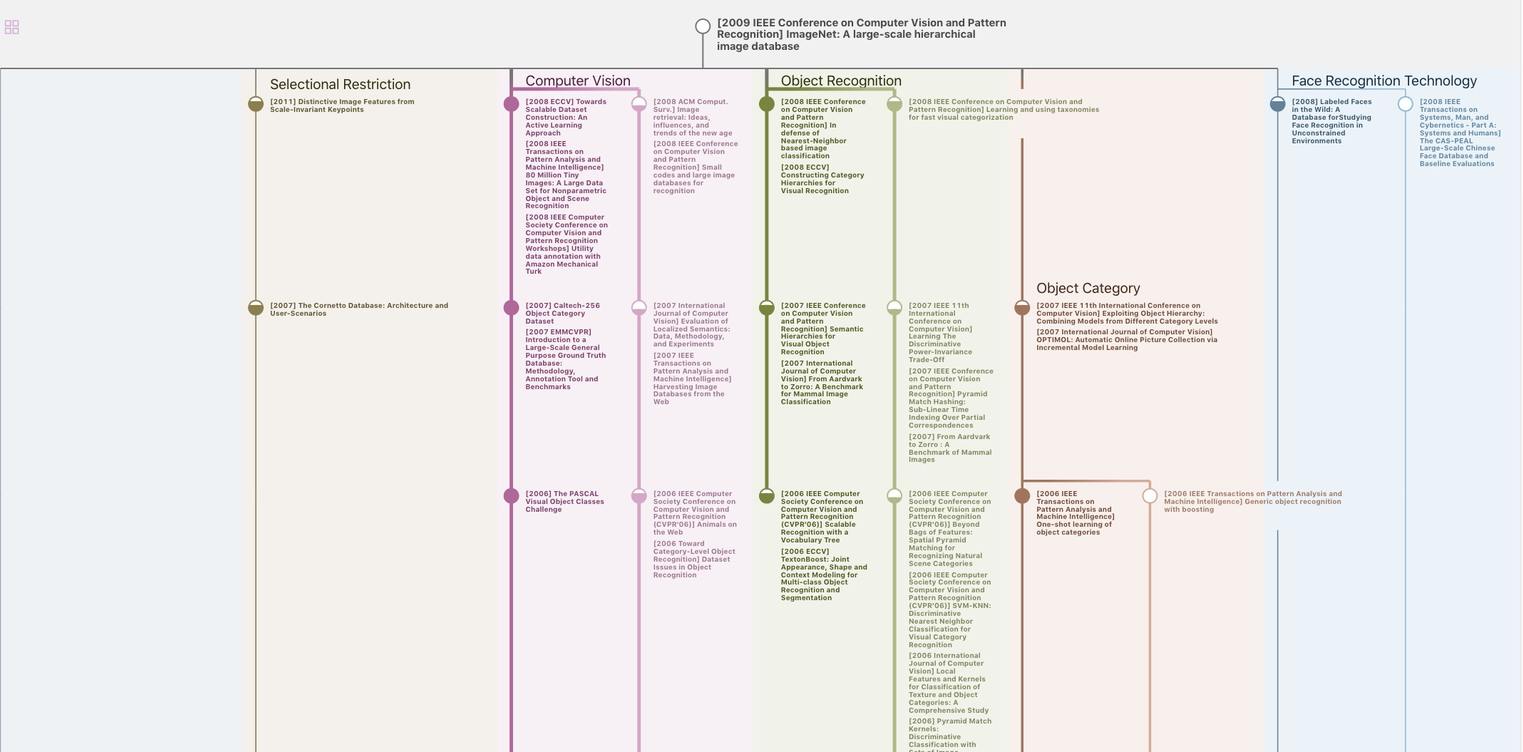
生成溯源树,研究论文发展脉络
Chat Paper
正在生成论文摘要