Bayesian Self-Supervised Learning Using Local and Global Graph Information
2023 IEEE 9TH INTERNATIONAL WORKSHOP ON COMPUTATIONAL ADVANCES IN MULTI-SENSOR ADAPTIVE PROCESSING, CAMSAP(2023)
摘要
Graph-guided learning has well-documented impact in a gamut of network science applications. A prototypical graph-guided learning task deals with semi-supervised learning over graphs, where the goal is to predict the nodal values or labels of unobserved nodes, by leveraging a few nodal observations along with the underlying graph structure. This is particularly challenging under privacy constraints or generally when acquiring nodal observations incurs high cost. In this context, the present work puts forth a Bayesian graph-driven self-supervised learning (Self-SL) approach that: (i) learns powerful nodal embeddings emanating from easier to solve auxiliary tasks that map local to global connectivity information; and, (ii) adopts an ensemble of Gaussian processes (EGPs) with adaptive weights as nodal embeddings are processed online. Unlike most existing deterministic approaches, the novel approach offers accurate estimates of the unobserved nodal values along with uncertainty quantification that is important especially in safety critical applications. Numerical tests on synthetic and real graph datasets showcase merits of the novel EGP-based Self-SL method.
更多查看译文
关键词
Local Information,Global Information,Self-supervised Learning,Global Graph Information,Gaussian Process,Graph Structure,Numerical Tests,Value Of Node,Semi-supervised Learning,Global Connectivity,Uncertainty Quantification,Safety-critical Applications,Unobserved Values,Neural Network,Model Parameters,Predictor Variables,Local Features,Input Features,Radial Basis Function,Local Connectivity,Graph Neural Networks,Embedding Learning,Minimum Mean Square Error,Eigenvector Centrality,Information Embedding,Low-dimensional Embedding,Network Delay,Conditional Likelihood,Temperature Dataset,Extra Features
AI 理解论文
溯源树
样例
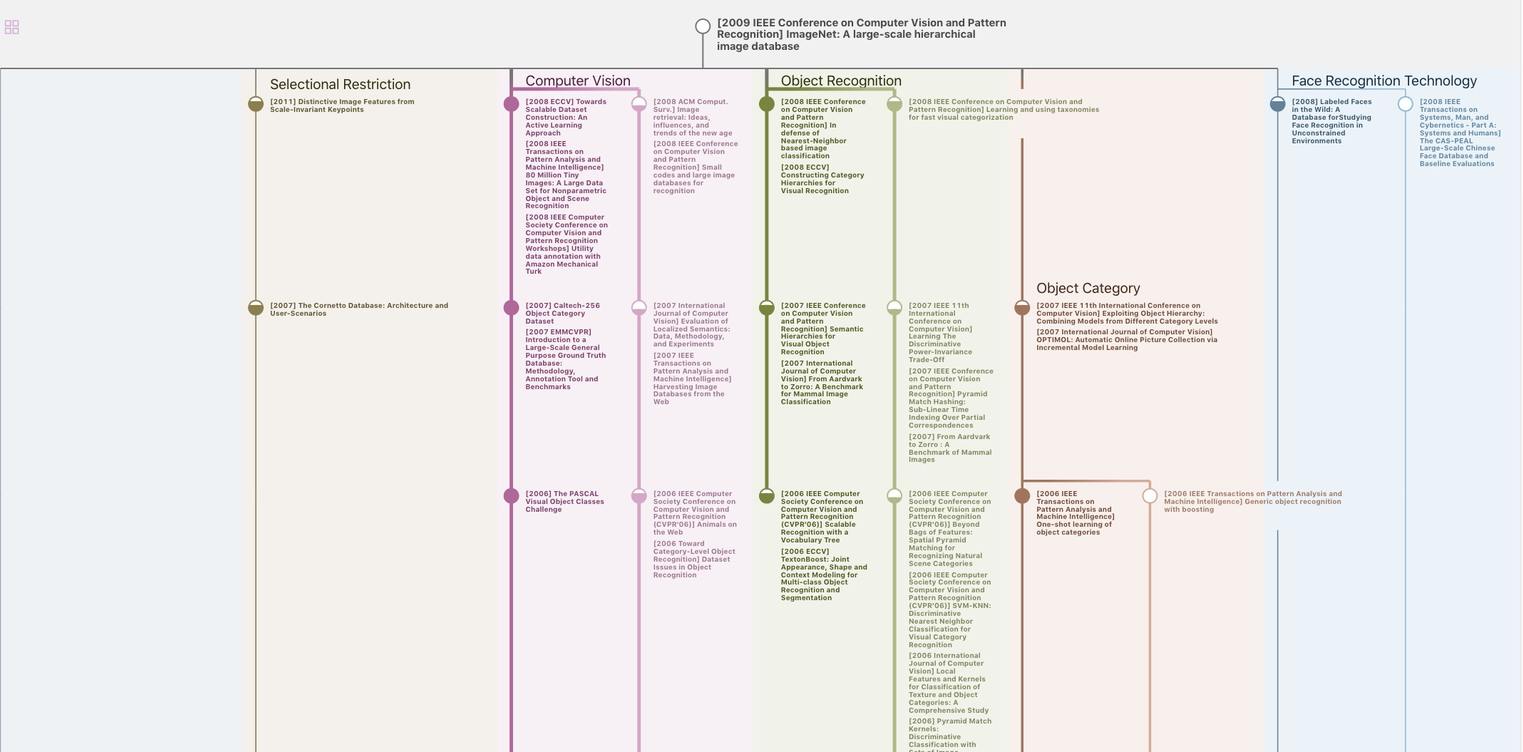
生成溯源树,研究论文发展脉络
Chat Paper
正在生成论文摘要