Deep Knowledge Tracing with Multiple Features
2023 Asia-Pacific Conference on Image Processing, Electronics and Computers (IPEC)(2023)
摘要
Knowledge tracing is an important task in online education platform. It aims to evaluate students' knowledge status and predict their future performance based on students' historical learning data. The existing methods only use interaction (exercise-response pair) sequence as model input and do not make full use of rich data collected by the platform. This paper proposes a Deep Knowledge Tracing model with Multiple Features (DKTMF), which considers three types of data that are easy to collect in online education platform: response time, exercise text and heterogeneous data. First, the negative exponential function about the interval time between responses is used to simulate students' forgetting behavior according to Ebbinghaus forgetting curve. Second, BERT and SimCSE are used to obtain the semantic features of the exercise text, and then the semantic similarity of the exercise is further calculated. Then, XGBoost is used to conduct feature engineering for heterogeneous data. Finally, the above three features are introduced into the deep knowledge tracing model based on Transformer. The experimental results show the effectiveness of DKTMF. Compared with the existing model, the average increase in AUC and ACC is 7.51% and 4.92% respectively, which can more accurately predict students' performance.
更多查看译文
关键词
knowledge tracing,deep learning,forgetting behavior,SimCSE,XGBoost
AI 理解论文
溯源树
样例
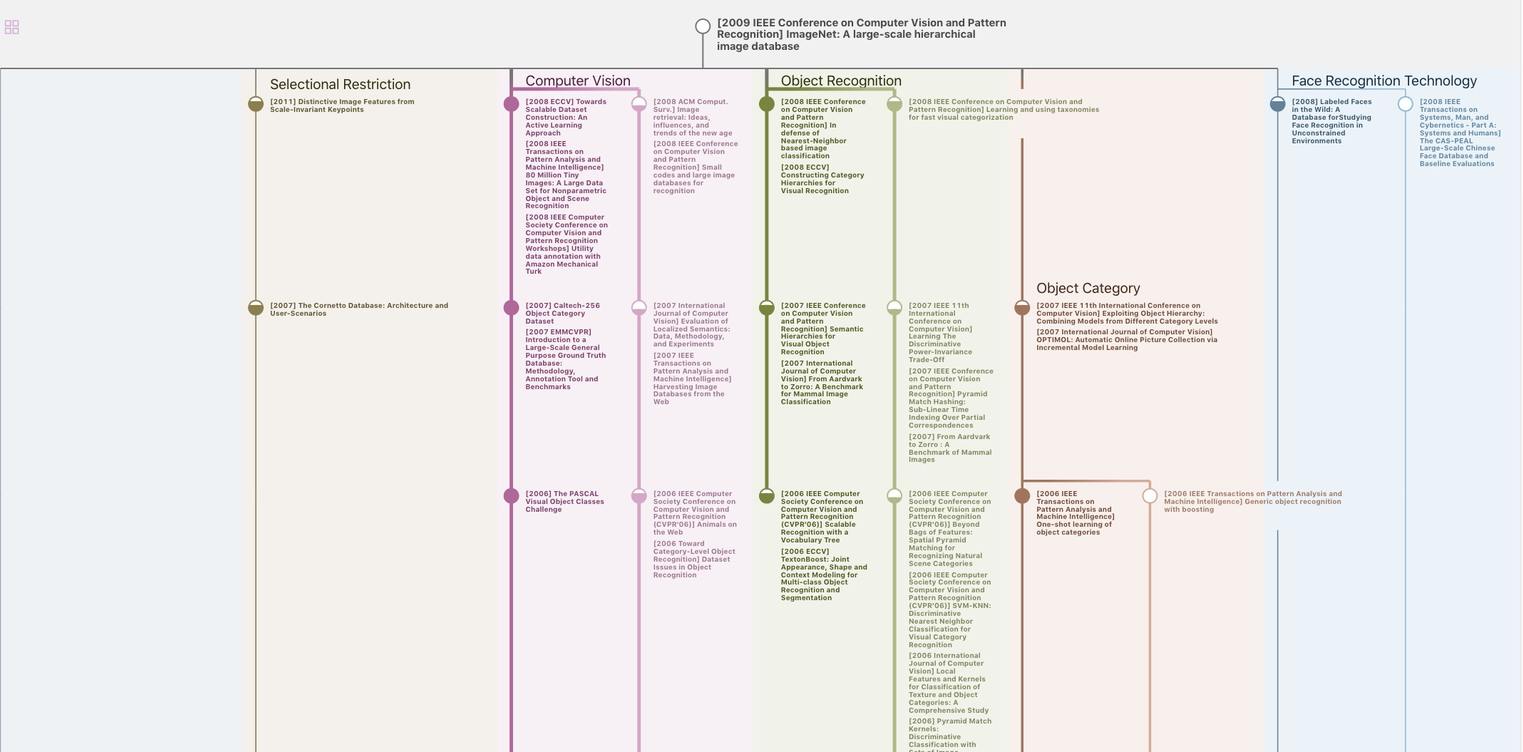
生成溯源树,研究论文发展脉络
Chat Paper
正在生成论文摘要