An Oversampling Method Based on KL-Divergence for Imbalanced Datasets and Credit Risk Assessment
2023 International Conference on New Trends in Computational Intelligence (NTCI)(2023)
摘要
Despite more than three decades of vigorous development, imbalanced learning is still attracted growing attention from both academic and finance. Skewed distributions for imbalanced datasets make classifiers biased towards the majority group. However, the opposite, the minority group, is more valuable in real world datasets. In this paper, we proposed an oversampling method E-SMOTE based on entropy theory to synthesis minority samples with designed weight over minority dangerous set. Firstly, positive dangerous samples are collected adaptively based on a division, where each minority sample is recognized as inner, danger or noise according to the distribution information of the nearest neighbors. Secondly, a sampling weight is designed based on KL-divergence of two distributions for one positive dangerous sample leaving or not. In other words, the sampling weight quantifies the importance of the positive dangerous sample. Finally, SMOTE synthesizes minority samples between positive dangerous sample and its nearest minority neighbors. In experiments, the proposed method is applied on 16 benchmark imbalanced datasets and the risk assessment problem of personal credit loan.
更多查看译文
关键词
imbalanced datasets,SMOTE,oversampling,KL-divergence,credit risk assessment
AI 理解论文
溯源树
样例
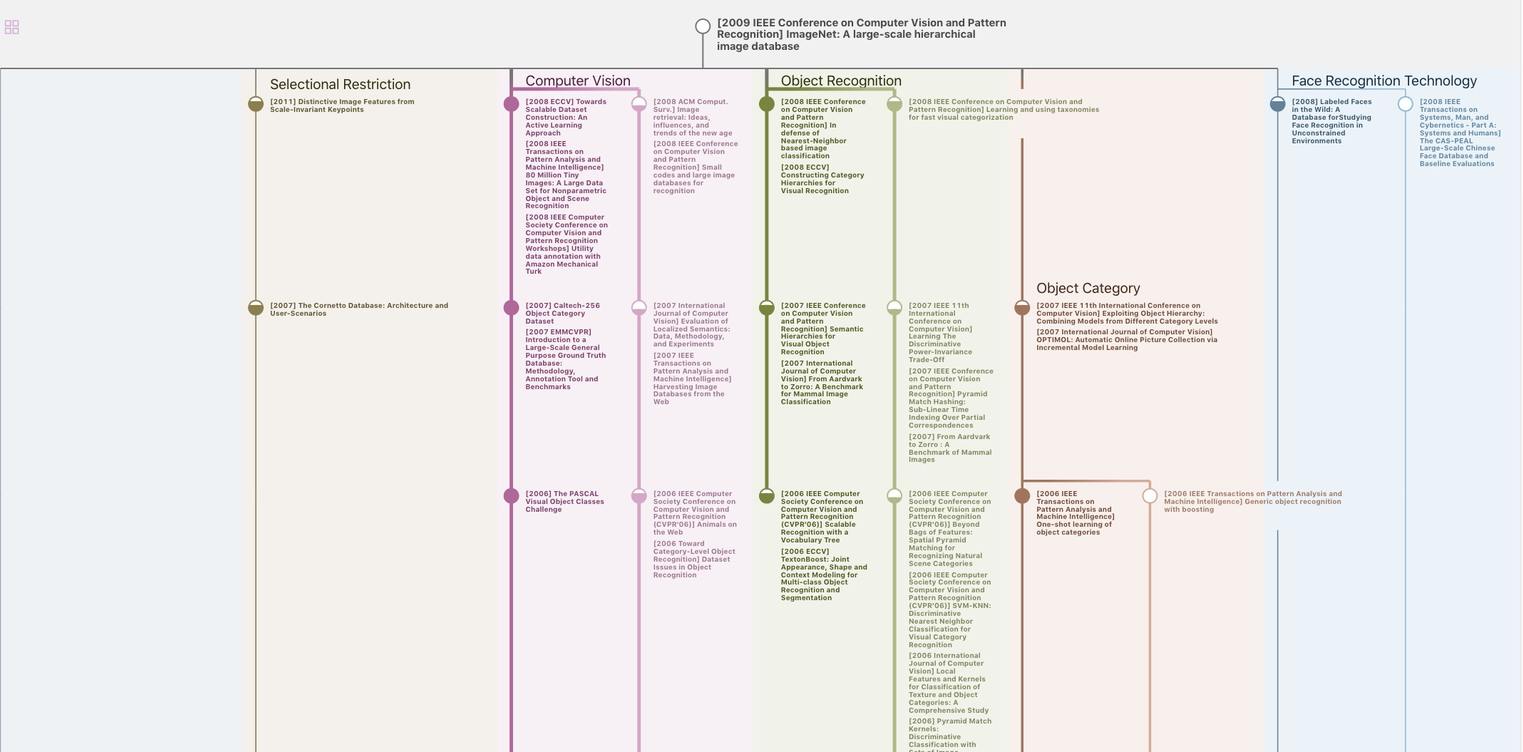
生成溯源树,研究论文发展脉络
Chat Paper
正在生成论文摘要