Mechanistic and Data-Driven Modelling of Operational Parameters Prediction on Oil and Gas Transportation Pipeline Network
2023 International Conference on New Trends in Computational Intelligence (NTCI)(2023)
摘要
Collection and transportation pipeline network of Crude oil is one of the critical infrastructures in a largescale oilfield. Fewer sensors are installed in the pipeline network and the collection is not timely, which makes it difficult to control the transportation of crude oil in the pipeline network in real time. Due to the lack of standards for data collection, traditional data-driven methods are difficult to achieve expected results. Therefore, a dual-driven modeling method of both mechanism and data is proposed to realize the integrated prediction of operating parameters at each place of the pipeline network. The real-time production data from each wellhead are input into the model. The pressure and temperature at each place of the pipeline are calculated by the Beggs-Bril and Leapienzon equations. The measured and calculated values are input into the transformer-based model with the constraints of storage and transportation mechanism. The calculated results of the equations are corrected for errors to achieve the purpose of accuracy. Results from experiments in oilfield gathering and transportation pipeline networks show that our model has the best prediction accuracy and generalization ability. Through a detailed ablation study, we demonstrate the effectiveness of each contribution in the overall architecture and methodology. The results show that the mechanism and data modeling approach outperform the storage and transportation mechanism model and the purely data-driven model.
更多查看译文
关键词
Collection and transportation pipeline network,operational parameters prediction,transformer model,deep learning
AI 理解论文
溯源树
样例
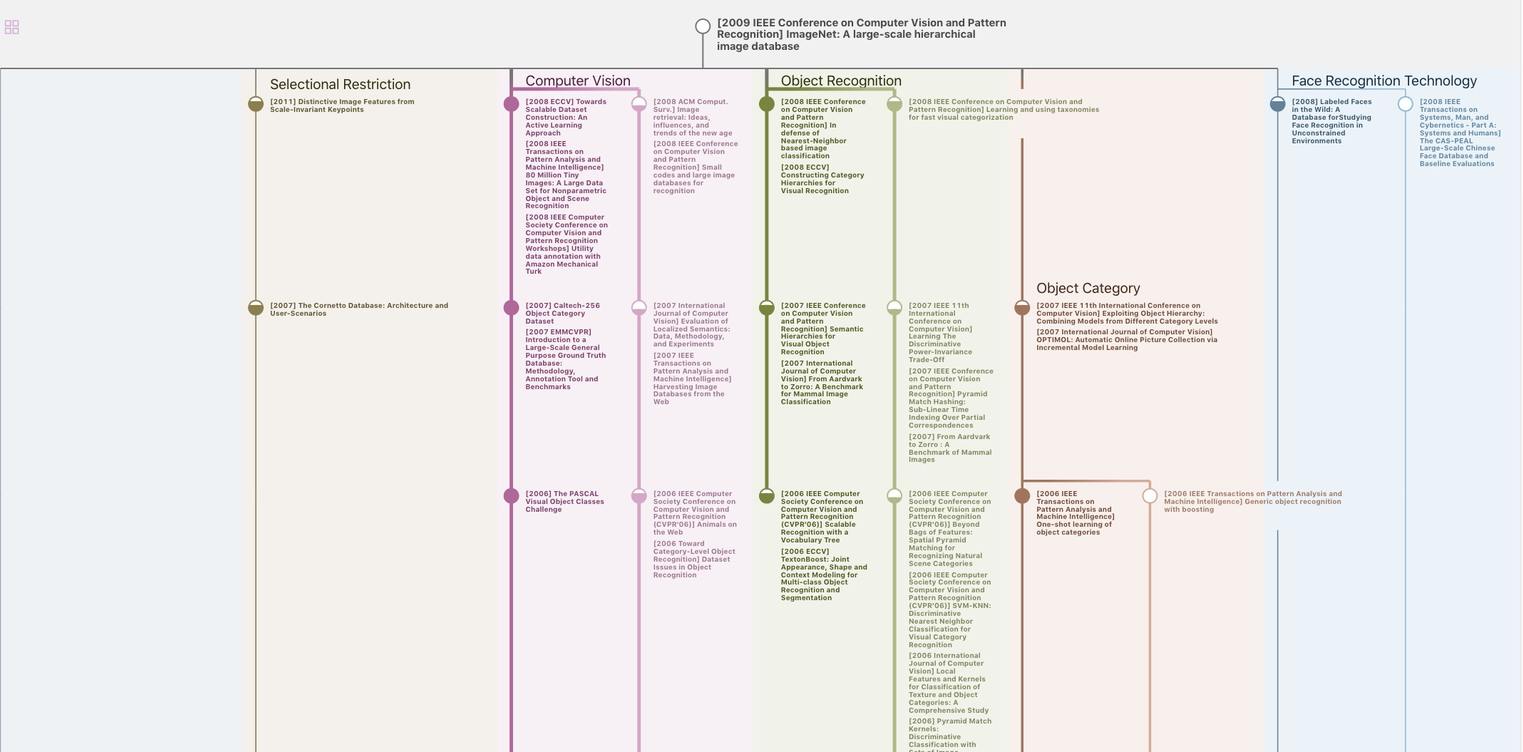
生成溯源树,研究论文发展脉络
Chat Paper
正在生成论文摘要