Digital Twin Based Learning Framework for Adaptive Fault Diagnosis In Microgrids with Autonomous Reconfiguration Capabilities.
Winter Simulation Conference(2023)
摘要
The world is increasingly reliant on energy systems, making them a critical infrastructure for various essential services. However, this also makes them vulnerable to attacks, which can result in significant disruptions and damage. Microgrid (MG) monitoring systems play a crucial role in ensuring the safety and reliability of energy systems. However, traditional fault diagnosis techniques are limited to already established faults due to the use of only historical data, making it challenging to keep up with the increasing demand for safety and reliability. This paper proposes a digital twin based machine learning (DTML) framework for fault diagnosis in MG monitoring systems, with a focus on assessing the resilience of MG end-to-end systems to potential disruptions from adversaries. The proposed framework utilizes digital twin based random forest (RF) and support vector machine (SVM) and logistic regression (LR) model and shows that the RF based model outperforms other models with an accuracy of 95%.
更多查看译文
关键词
Learning Framework,Fault Diagnosis,Digital Twin,Diagnosis Framework,Fault Diagnosis Framework,Adaptive Fault Diagnosis,Machine Learning,Logistic Regression Model,Support Vector Machine,Energy System,Support Vector Machine Model,Critical Infrastructure,Machine Learning Framework,Support Vector Machine Regression,Prophylaxis,Training Dataset,Test Dataset,Decision Tree,Machine Learning Models,Binary Classification,Physical System,Natural Disasters,Random Forest Model,Renewable Energy Sources,Data-driven Models,Internet Of Things,Data-driven Methods,F1 Score,Power System,Fault Scenarios
AI 理解论文
溯源树
样例
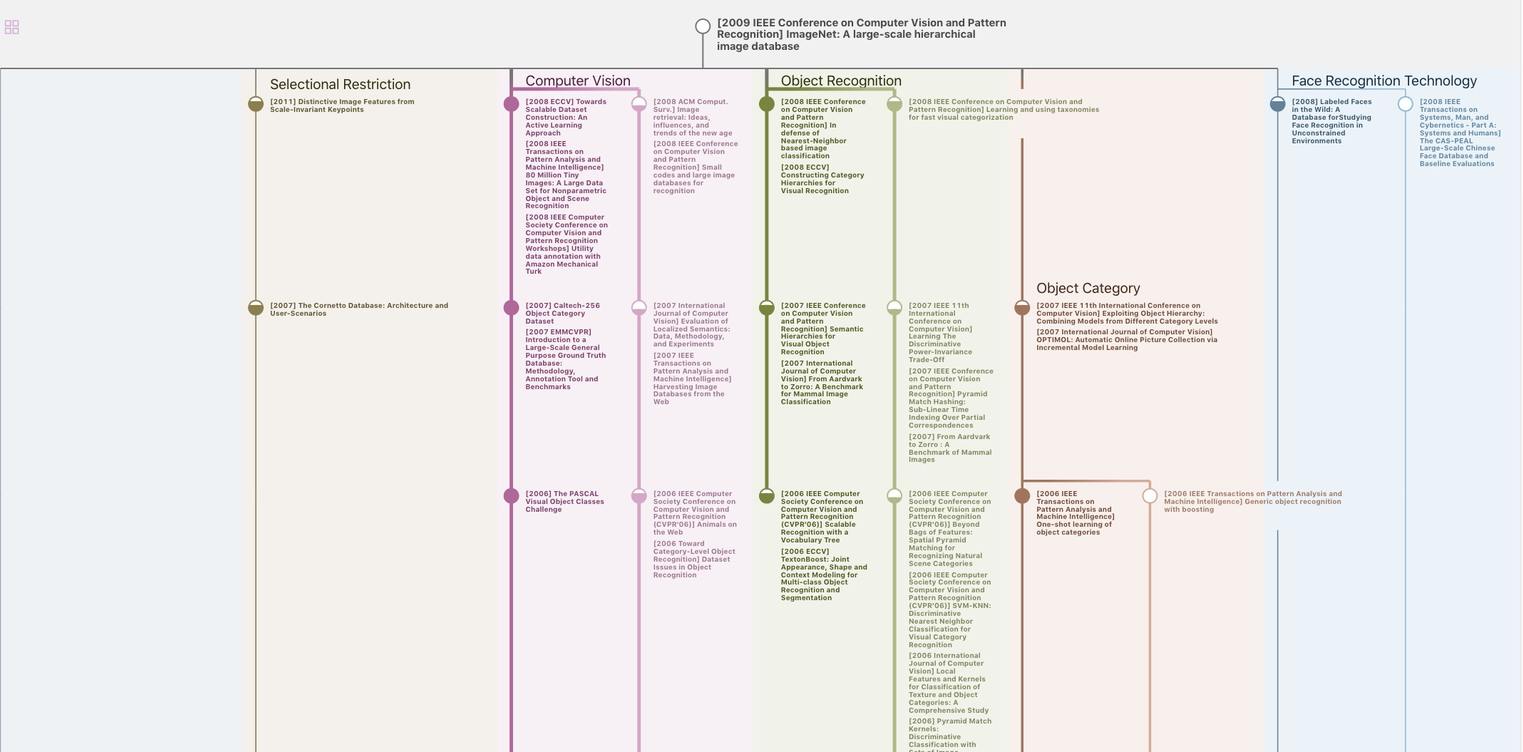
生成溯源树,研究论文发展脉络
Chat Paper
正在生成论文摘要