scGAT: A Cell-Type Annotation Framework for Single-Cell Transcriptomics Using Graph Attention Network and Meta Learning
2023 IEEE International Conference on Medical Artificial Intelligence (MedAI)(2023)
摘要
Single-cell RNA sequencing (scRNA-seq) technology has emerged as a valuable tool for classifying cell types across various species, tissues, and environmental conditions, thereby advancing the field of life sciences. Automatic cell annotation plays a crucial role in bioinformatics, addressing the challenges associated with time-consuming and labor-intensive manual cell annotation. Although numerous cell annotation algorithms exist, their performance is often constrained by the quality of reference data and inherent technical noise in biological experiments. To address these challenges, we propose the integration of a heterogeneous graph neural network into our cell annotation model, named scGAT. This integration leverages the intricate relationships between expressed genes and cells while disregarding unexpressed genes. Additionally, we introduce a novel approach that utilizes prototype cells to mitigate technical noise. However, the use of prototype cells may lead to limitations in sample size, prompting us to employ meta-learning techniques to determine the model's initial parameters. To assess the effectiveness of scGAT in cell annotation tasks, we conducted comparative analyses using real datasets against other models. The experimental results clearly demonstrate that scGAT significantly outperforms alternative methods.
更多查看译文
关键词
cell annotation,graph attention network,meta learning,scRNA-seq
AI 理解论文
溯源树
样例
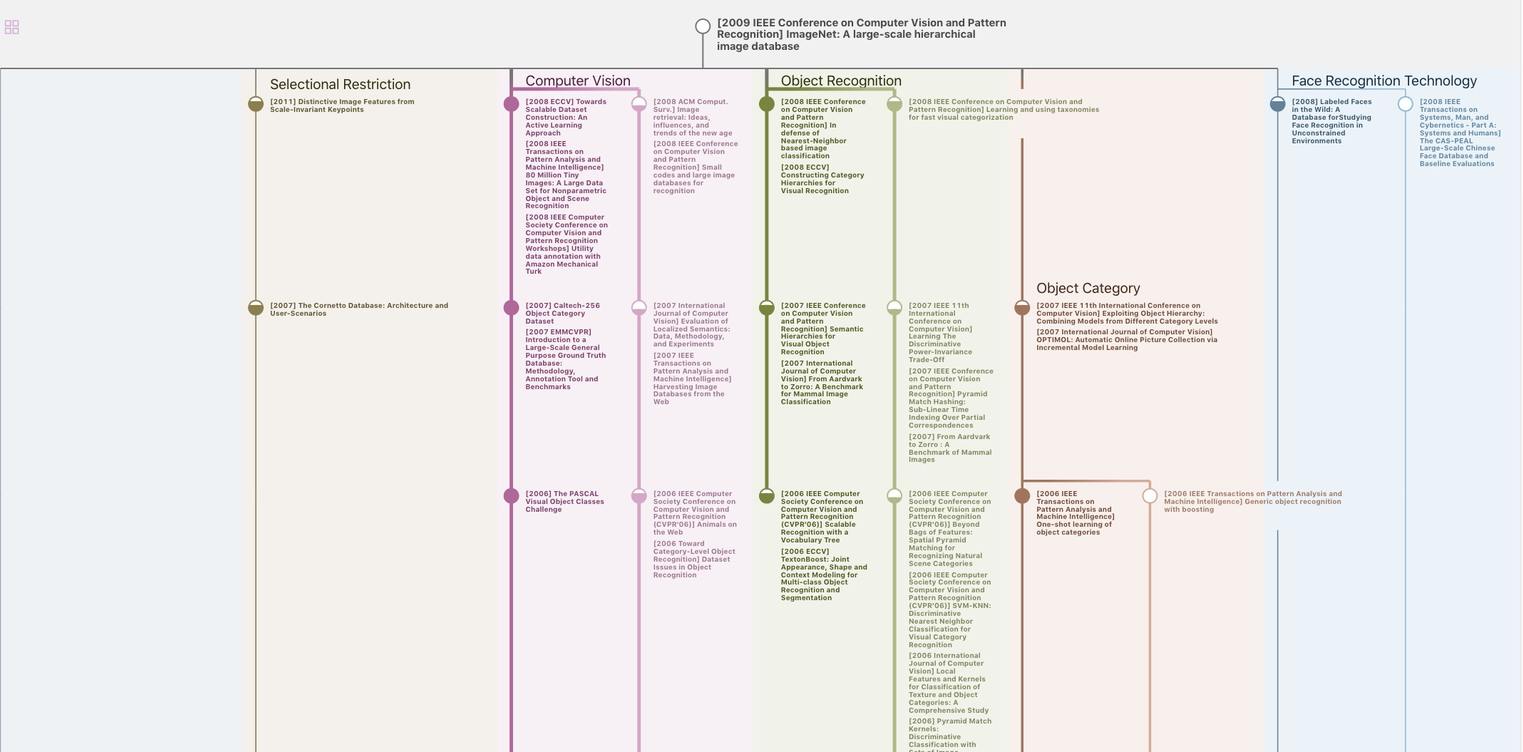
生成溯源树,研究论文发展脉络
Chat Paper
正在生成论文摘要