Positive Sample Augmentation with Graph Mixup for DDI Prediction
2023 IEEE International Conference on Medical Artificial Intelligence (MedAI)(2023)
摘要
Unexpected drug-drug interactions (DDIs) may occur when drugs are taken at the same time. Harmful DDIs can reduce drug efficacy and even increase unintended toxicity, putting patients treated with different drugs at risk. Previous works on DDI prediction mainly rely on hand-engineered domain knowledge, which is laborious to obtain and cannot handle the problem of sparse positive samples in DDI prediction. Even though some works attempt to handle data sparsity from the perspective of data distribution to improve the generalization ability of the model, they are limited to negative samples distribution. To address these limitations, we propose a novel method, Graph Mixup for Positive Sample Augmentation (GMPSA), to effectively combine positive drug molecule graphs and extend the training distribution to alleviate the problem of sparse positive samples. To model the graph signals and graph structures jointly, we map source graphs to the fused gromov-wasserstein metric space, then minimizes the weighted sum of transportation distances between the distributions of the source graphs and the objective graph in this metric space. By doing so, interpolated positive samples improve the the generalization error of the model. Experiments results on a real-word dataset show that our method effectively improve the perfomermance in DDI prediction on a state-of-the-art architecture. Further studies also verify the robustness and generalizability of GMPSA under different GNN backbones and parameter settings.
更多查看译文
关键词
drug-drug interaction prediction,data augmentation,imbalance problem,graph neural networks
AI 理解论文
溯源树
样例
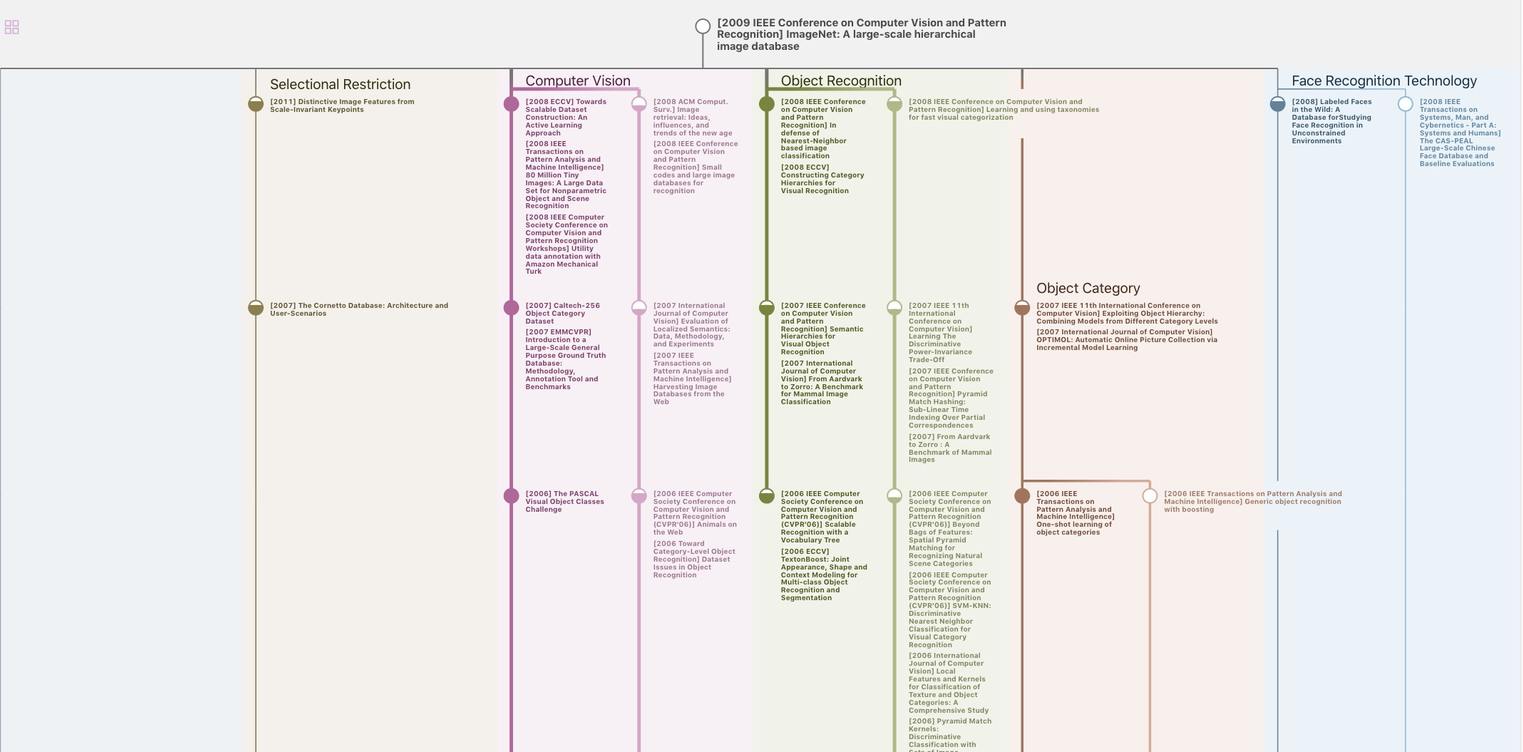
生成溯源树,研究论文发展脉络
Chat Paper
正在生成论文摘要