Reusing Historical Observations in Natural Policy Gradient.
Winter Simulation Conference(2023)
摘要
Reinforcement learning provides a mathematical framework for learning-based control, whose success largely depends on the amount of data it can utilize. The efficient utilization of historical samples obtained from previous iterations is essential for expediting policy optimization. Empirical evidence has shown that offline variants of policy gradient methods based on importance sampling work well. However, existing literature often neglect the interdependence between observations from different iterations, and the good empirical performance lacks a rigorous theoretical justification. In this paper, we study an offline variant of the natural policy gradient method with reusing historical observations. We show that the biases of the proposed estimators of Fisher information matrix and gradient are asymptotically negligible, and reusing historical observations reduces the conditional variance of the gradient estimator. The proposed algorithm and convergence analysis could be further applied to popular policy optimization algorithms such as trust region policy optimization. Our theoretical results are verified on classical benchmarks.
更多查看译文
关键词
Historical Observations,Policy Gradient,Natural Gradient,Natural Policy Gradient,Variety Of Conditions,Gradient Approximation,Optimal Policy,Popular Algorithms,Fisher Information Matrix,Policy Gradient Method,Neural Network,Unbiased,Likelihood Ratio,Step Size,Gradient Descent,Identity Matrix,State Space,Parameter Space,Transition Probabilities,Ordinary Differential Equations,Policy Gradient Algorithm,Variance Reduction,Solution Trajectory,Stochastic Gradient Descent,Policy Parameters,Markov Decision Process,Simulation Error,Bias Term,Stochastic Gradient,Fixed State
AI 理解论文
溯源树
样例
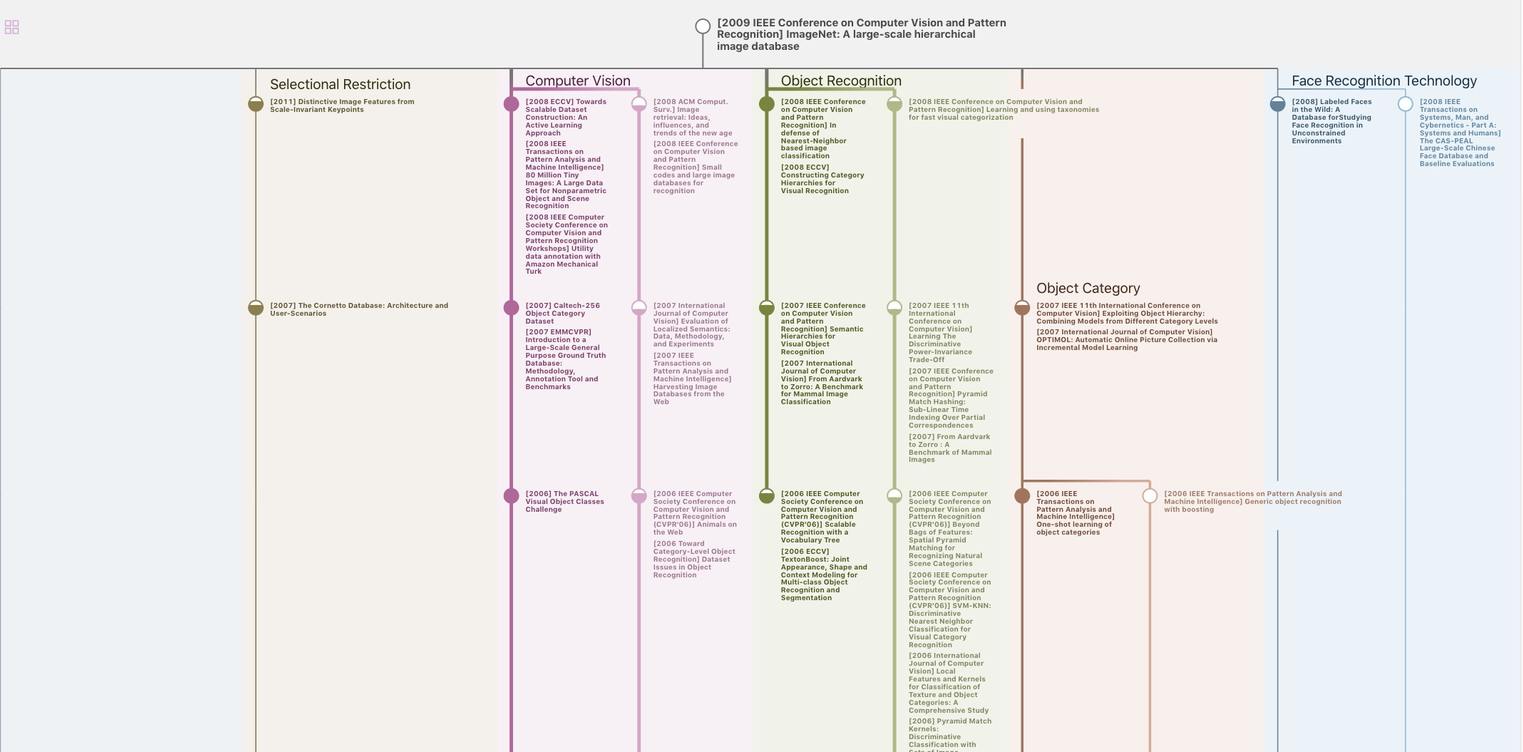
生成溯源树,研究论文发展脉络
Chat Paper
正在生成论文摘要