Power and Memory Efficient High-Speed RL Based Run time Power Manager for Edge Computation.
Midwest Symposium on Circuits and Systems(2023)
摘要
Run-time power management poses severe challenges in modern-day edge computing and the adaptability of these run-time power managers to new workloads has been a major concern. Reinforcement learning (RL) based algorithms are able to address this issue of adaptability to unseen load scenarios in the area of High-performance computing (HPC). However, the performance of RL-based run time power managers on the edge degrades due to the constraints it faces post-deployment. The primary reasons for the performance degradation of the RL-based run time power manager on edge are the random actions taken during the long exploratory phase and the considerable amount of memory required for its smooth execution. This motivated us to propose a power and memory-efficient high-speed RL-based run time manager for the edge computation. This reduces the exploratory time by offline-online co-optimization policy and memory consumption post-deployment by removing sparse states. Subsequently, the proposed methodology is implemented on the edge device Jetson Xavier board. It reduces exploratory time by 36% with a reduction in memory footprint by 40% compared to state-of-the-art approaches with average power savings of 29.21% compared to OS_Performance mode, 26.77% compared to OS_Schedutil mode, 19.24% compared to OS_Ondemand mode and 15.42% compared to state-of-the-art Q-learning on edge computing platforms.
更多查看译文
关键词
Edge computing,Reinforcement learning (RL),Power manager,Exploratory phase,Memory,Offline,Online,Co-optimization,Sparse state
AI 理解论文
溯源树
样例
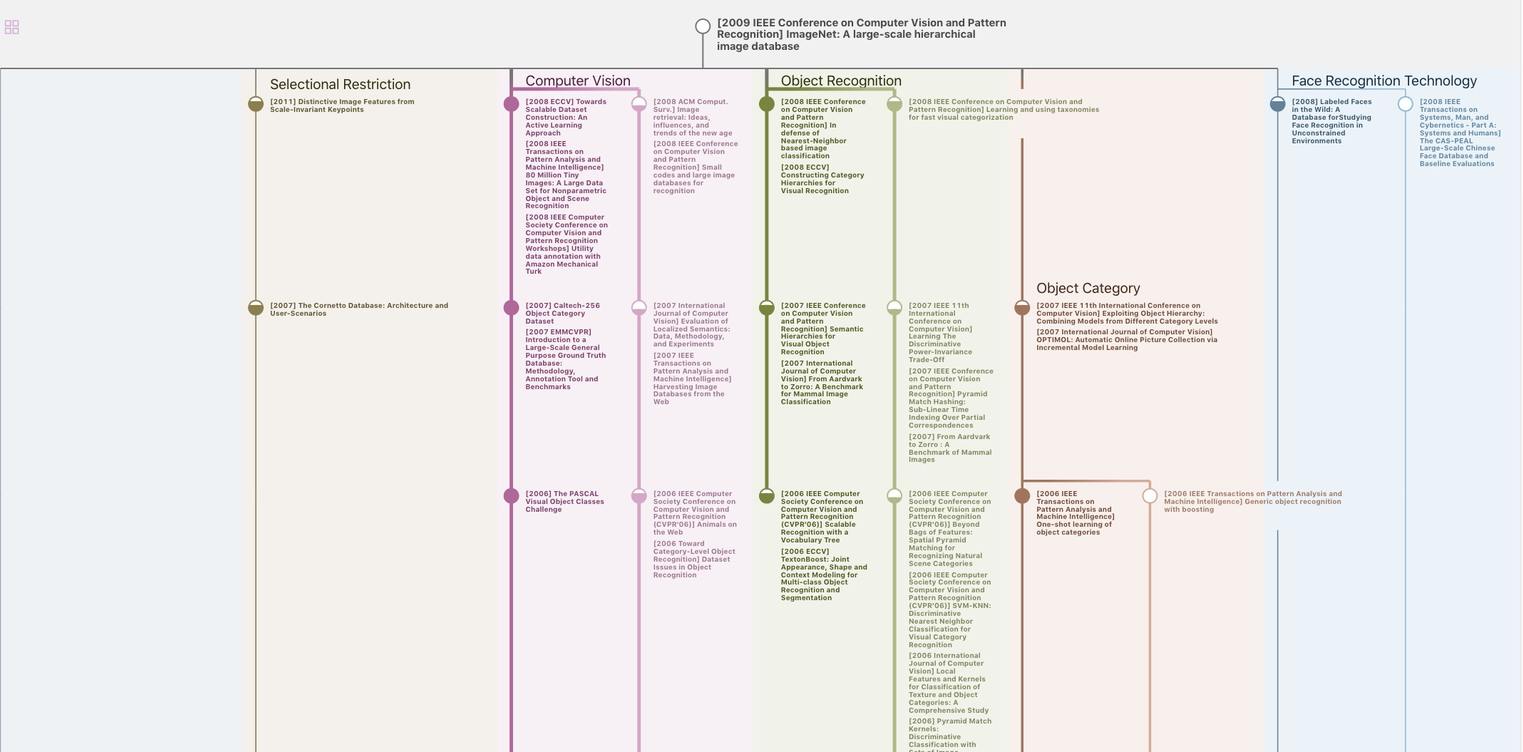
生成溯源树,研究论文发展脉络
Chat Paper
正在生成论文摘要