Epsilon Optimal Sampling.
Winter Simulation Conference(2023)
摘要
Epsilon Optimal Sampling (EOS) is a novel algorithm that seeks to reduce the computational complexity of selecting the best design using stochastic simulation. EOS is an Optimal Computing Budget Allocation (OCBA) type algorithm that reduces computational complexity by integrating machine learning (ML) models into the simulation optimization algorithm. EOS avoids the pitfall of trading computational overhead in simulation execution for computational overhead in ML model training by using a concept we call policy stability. In this paper, we present the concept of policy stability, how it can be used to improve dynamic sampling techniques, and how low-fidelity ML estimates can be integrated into the process. Numerical results are presented to provide evidence as to the improvement in computational efficiency that can be achieved when using EOS in conjunction with ML models over the standard OCBA algorithm.
更多查看译文
关键词
Optimal Sample,Machine Learning,Numerical Results,Computational Efficiency,Machine Learning Models,Optimal Allocation,Computational Overhead,Stochastic Simulations,Budget Allocation,Train Machine Learning Models,Simulation Optimization,Concept Of Stability,Integration Of Machine Learning,Improvement In Computational Efficiency,Policy Stability,Heuristic,Parameter Estimates,Confidence Level,Random Variables,Simulated Data,Error Reduction,Online Learning,Iterative Sampling,Classification Algorithms,Uniform Density,Learnable Parameters,Gaussian Mixture Model,Parallelization,General Case,Distribution Of Parameters
AI 理解论文
溯源树
样例
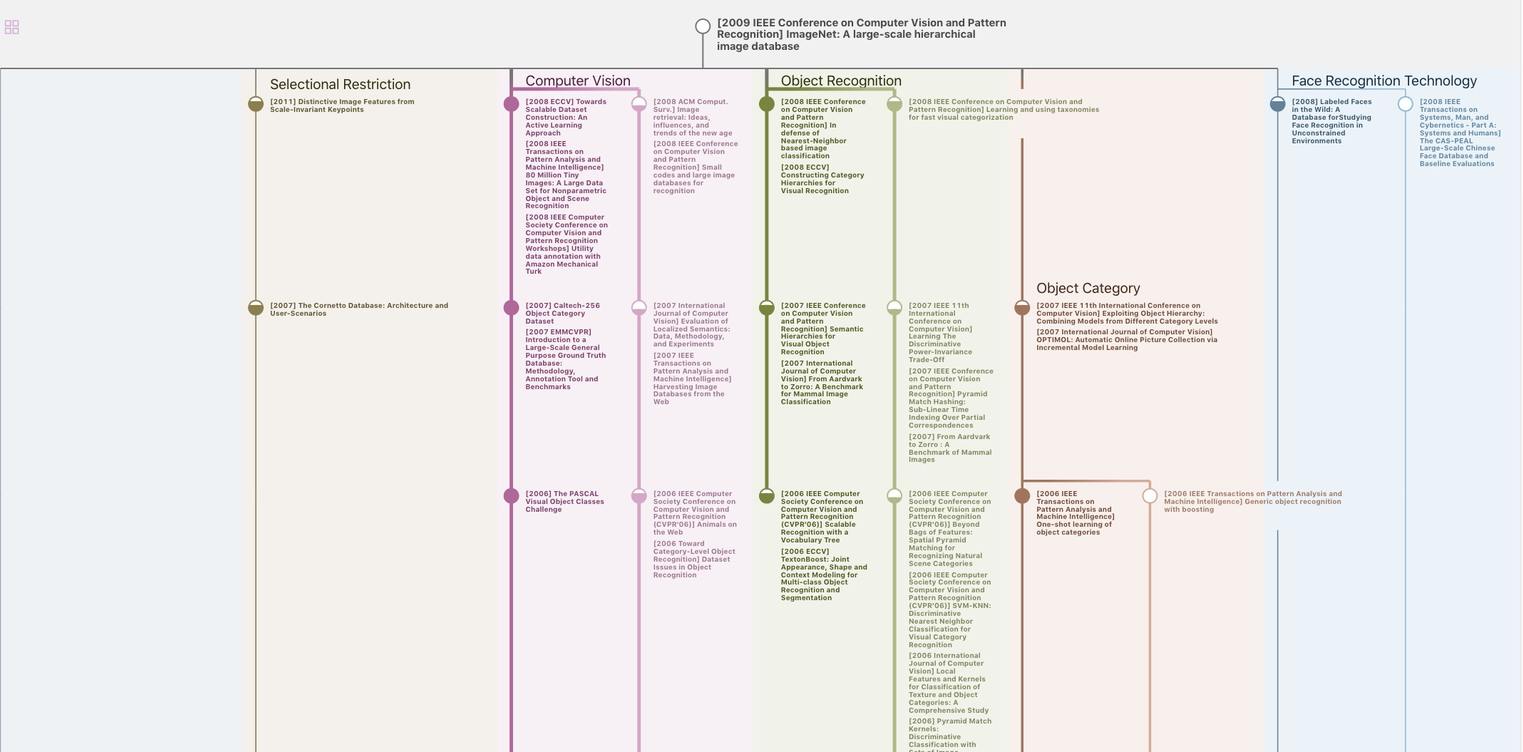
生成溯源树,研究论文发展脉络
Chat Paper
正在生成论文摘要