Convolutional neural network based on the fusion of image classification and segmentation module for weed detection in alfalfa
PEST MANAGEMENT SCIENCE(2024)
摘要
BACKGROUND: Accurate and reliable weed detection in real time is essential for realizing autonomous precision herbicide application. The objective of this research was to propose a novel neural network architecture to improve the detection accuracy for broadleaf weeds growing in alfalfa. RESULTS: A novel neural network, ResNet-101-segmentation, was developed by fusing an image classification and segmentation module with the backbone selected from ResNet-101. Compared with existing neural networks (AlexNet, GoogLeNet, VGG16, and ResNet-101), ResNet-101-segmentation improved the detection of Carolina geranium, catchweed bedstraw, mugwort and speedwell from 78.27% to 98.17%, from 79.49% to 98.28%, from 67.03% to 96.23%, and from 75.95% to 98.06%, respectively. The novel network exhibited high values of confusion matrices (>90%) when trained with sufficient data sets. CONCLUSION: ResNet-101-segmentation demonstrated excellent performance compared with existing models (AlexNet, GoogLeNet, VGG16, and ResNet-101) for detecting broadleaf weeds growing in alfalfa. This approach offers a promising solution to increase the accuracy of weed detection, especially in cases where weeds and crops have similar plant morphology. (c) 2024 Society of Chemical Industry.
更多查看译文
关键词
artificial intelligence,precision herbicide application,image classification,image segmentation,broadleaves
AI 理解论文
溯源树
样例
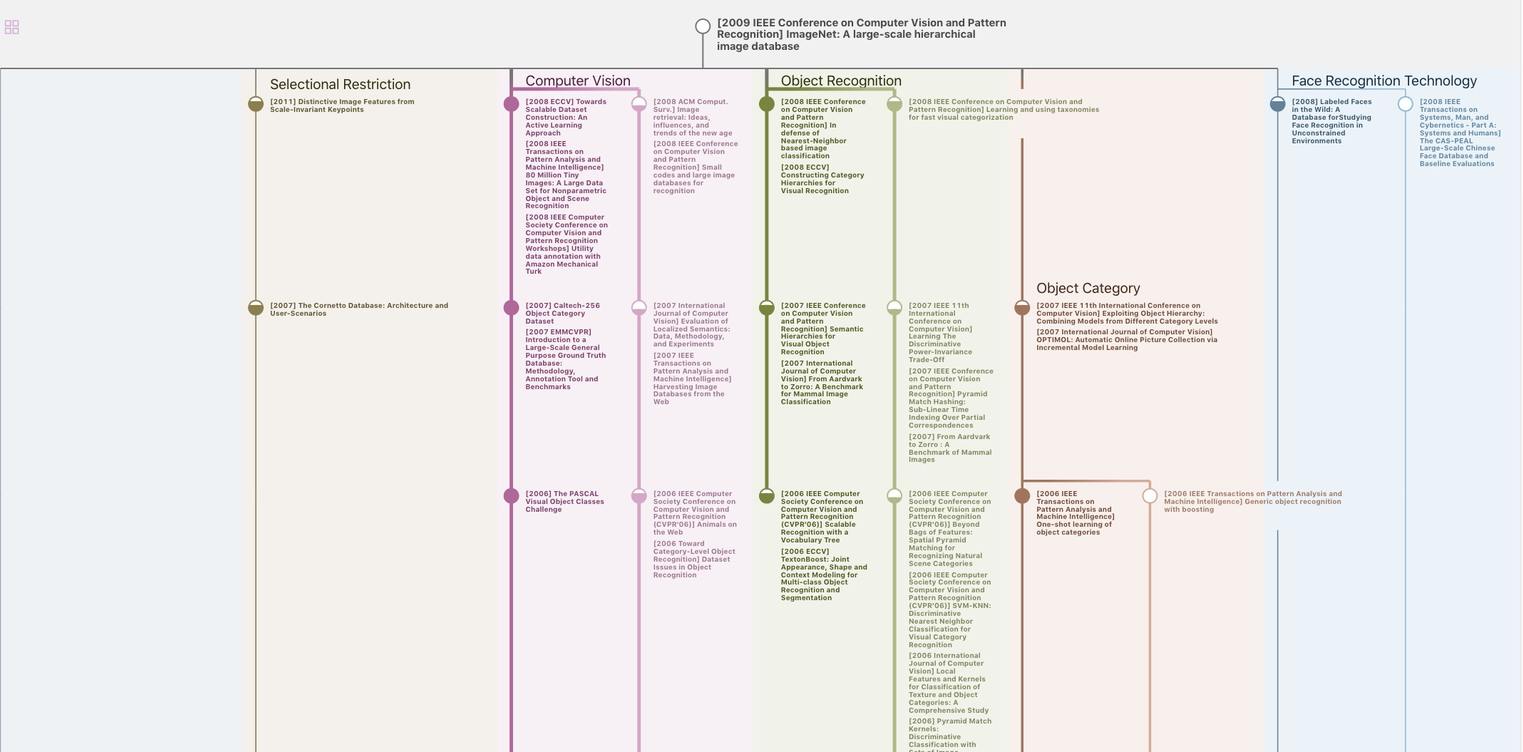
生成溯源树,研究论文发展脉络
Chat Paper
正在生成论文摘要