Sampling-based Stochastic Data-driven Predictive Control under Data Uncertainty
CoRR(2024)
摘要
We present a stochastic output-feedback data-driven predictive control scheme
for linear time-invariant systems subject to bounded additive disturbances and
probabilistic chance constraints. The approach uses data-driven predictors
based on an extension of Willems' fundamental lemma from behavioral systems
theory and a single persistently exciting input-output data trajectory.
Compared to current state-of-the-art approaches that rely on availability of
exact disturbance data, we deterministically approximate the chance constraints
in a sampling-based fashion by leveraging a novel parameterization of the
unknown disturbance data trajectory, considering consistency with the measured
data and the system class. A robust constraint on the first predicted step
guarantees recursive feasibility of the proposed controller as well as
constraint satisfaction in closed-loop. We show robust asymptotic stability in
expectation under further standard assumptions. A numerical example
demonstrates the efficiency of the proposed control scheme.
更多查看译文
AI 理解论文
溯源树
样例
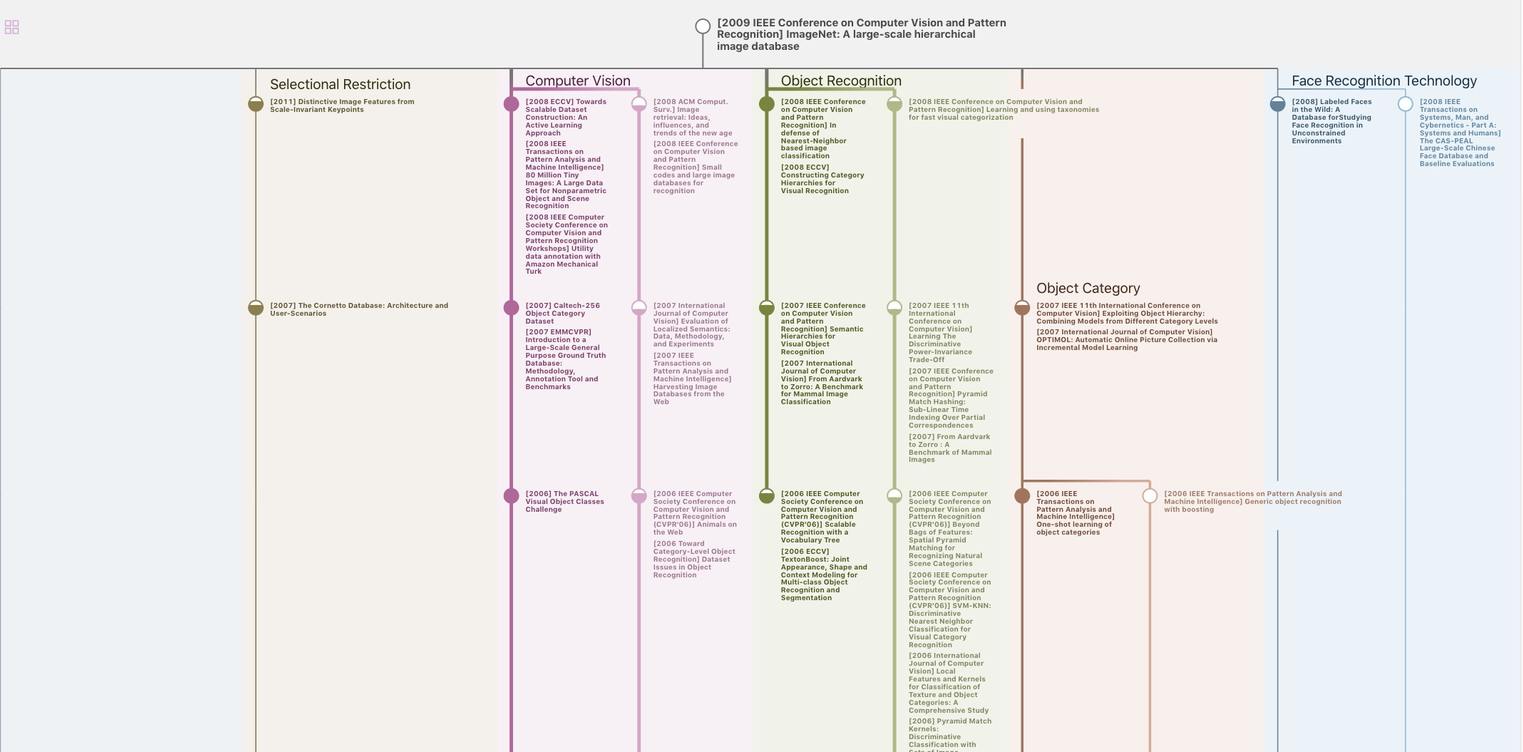
生成溯源树,研究论文发展脉络
Chat Paper
正在生成论文摘要