Prediction of concrete compressive strength using deep neural networks based on hyperparameter optimization
COGENT ENGINEERING(2024)
摘要
This paper describes deep neural network (DNN) models based on hyperparameter optimization for the prediction of the compressive strength of concrete. The novelty of this research lies in the implementation of optimized hyperparameters to train the DNN models with the aim to enhance their predictive accuracy. Utilizing the Keras Tuner library, the most effective hyperparameters for the DNN models were identified. These models are then trained and evaluated using an experimental dataset of 1030 instances, encompassing nine quantitative variables that include various concrete mix ingredients and age. The target variable for all models is the compressive strength of concrete. The assessment of model performance, based on metrics like mean squared error (MSE) and R2 values, was conducted on previously unseen test data. The optimal configuration for the hidden layers in the model was identified as five layers, containing 12, 16, 16, 40, and 26 neurons, respectively. The optimal learning rate for the model was 0.001. With this set of optimal hyperparameters, the best DNN model achieved an MSE of 28.76 and an R2 value of 0.89 on the testing data. The results demonstrate a significant improvement in the DNN models' performance when trained with the optimized hyperparameters. In comparison to the regression model, the performance of the DNN models was significantly better. Adopting the predictive models developed in this research offers the potential for substantial cost and time savings by circumventing the need for labour-intensive and time-consuming laboratory tests.
更多查看译文
关键词
Concrete,deep,neural,networks,hyperparameter,optimization,Sanjay Kumar Shukla, Edith Cowan University, Australia,Artificial Intelligence,Computer Science (General),Civil, Environmental and Geotechnical Engineering
AI 理解论文
溯源树
样例
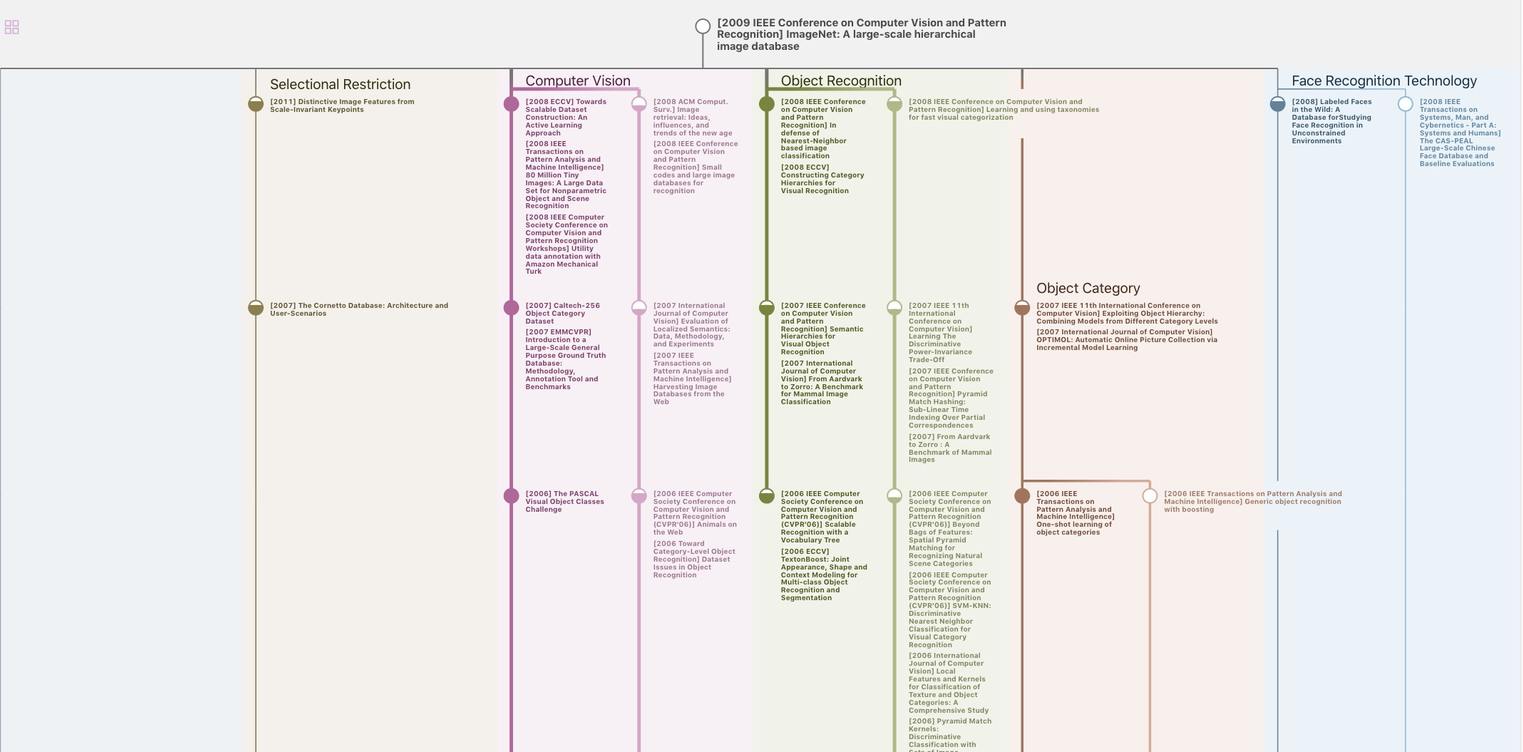
生成溯源树,研究论文发展脉络
Chat Paper
正在生成论文摘要