Predicting Crowdedness Level of the Mass Rapid Transit (MRT) Platform Using Big Data Framework: A Case Study in Singapore
2023 IEEE International Conference on Industrial Engineering and Engineering Management (IEEM)(2023)
Abstract
A reliable and cost-effective public transportation system plays an essential role in many cities. Understanding the crowd density for public transport is crucial for smart city and urban planner. The main objective of this paper is to monitor and predict the crowdedness level on the Mass Rapid Transit (MRT) platforms in Singapore. Firstly, we design and implement a scalable big data framework to support this task. Secondly, in order to address the issue of class imbalance, Synthetic Minority Over-Sampling Technique (SMOTE) is integrated with a balanced random forest classifier to predict the crowdedness level of station platforms. Extensive experiments are conducted to evaluate the performance of the proposed approach on real datasets from Singapore Land Transport Authority (LTA) DataMall API. The results demonstrate the accuracy and efficiency of the proposed approach.
MoreTranslated text
Key words
Big data framework,crowdedness level prediction,random forest classifier,SMOTE
求助PDF
上传PDF
View via Publisher
AI Read Science
Must-Reading Tree
Example
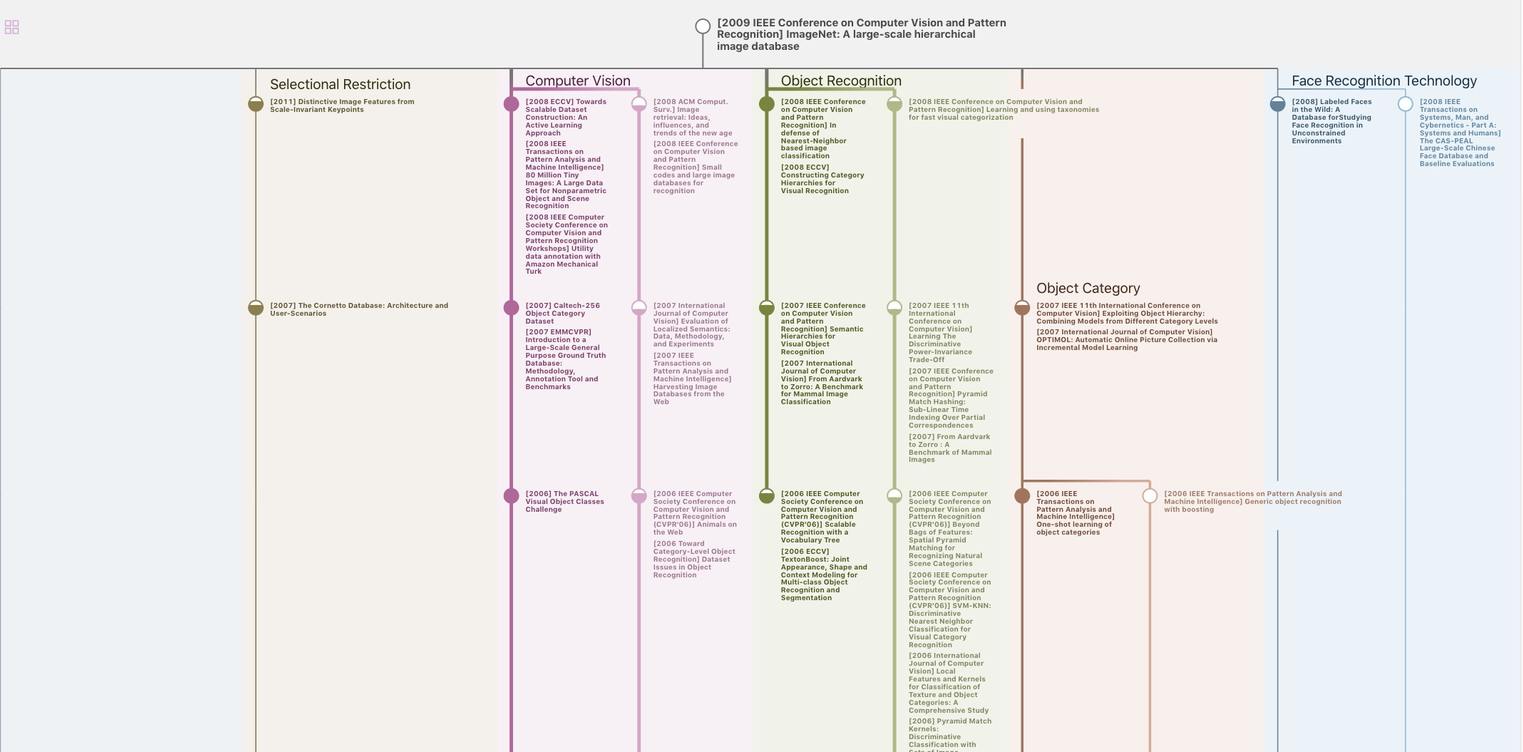
Generate MRT to find the research sequence of this paper
Data Disclaimer
The page data are from open Internet sources, cooperative publishers and automatic analysis results through AI technology. We do not make any commitments and guarantees for the validity, accuracy, correctness, reliability, completeness and timeliness of the page data. If you have any questions, please contact us by email: report@aminer.cn
Chat Paper
Summary is being generated by the instructions you defined