Bi-Orthogonal Projection Learning for Dimensionality Reduction
2023 3rd International Conference on Intelligent Power and Systems (ICIPS)(2023)
摘要
This paper proposes a novel bi-orthogonal projection learning (BOPL) for dimensionality reduction (DR) methods, which further extends the existing DR to a more flexible, robust, and sparse embedding framework. Unlike conventional methods that use only one kind of projection for learning in DR, the proposed BOPL introduces two kinds of projection, which are orthogonal and provides the true projection the freedom for more accurate data transformation in subspace learning. Inspired by the observation that the two projections share many similar data structures, the projections are expected to preserve the similarity structure of data by using two different reconstruction ways. The proposed method can also handle data corrupted by noises since a sparse item is employed to compensate for the noises during DR. Several novel unsupervised DR methods (i.e., BOPL_PCA, BOPL_NPE, and BOPL_LPP) are derived from the proposed framework. The results of experiments on the natural and synthetic data sets demonstrate that the proposed methods outperform the existing well-established DR methods.
更多查看译文
关键词
dimensionality reduction,sparse representation,structure-consistency,subspace learning
AI 理解论文
溯源树
样例
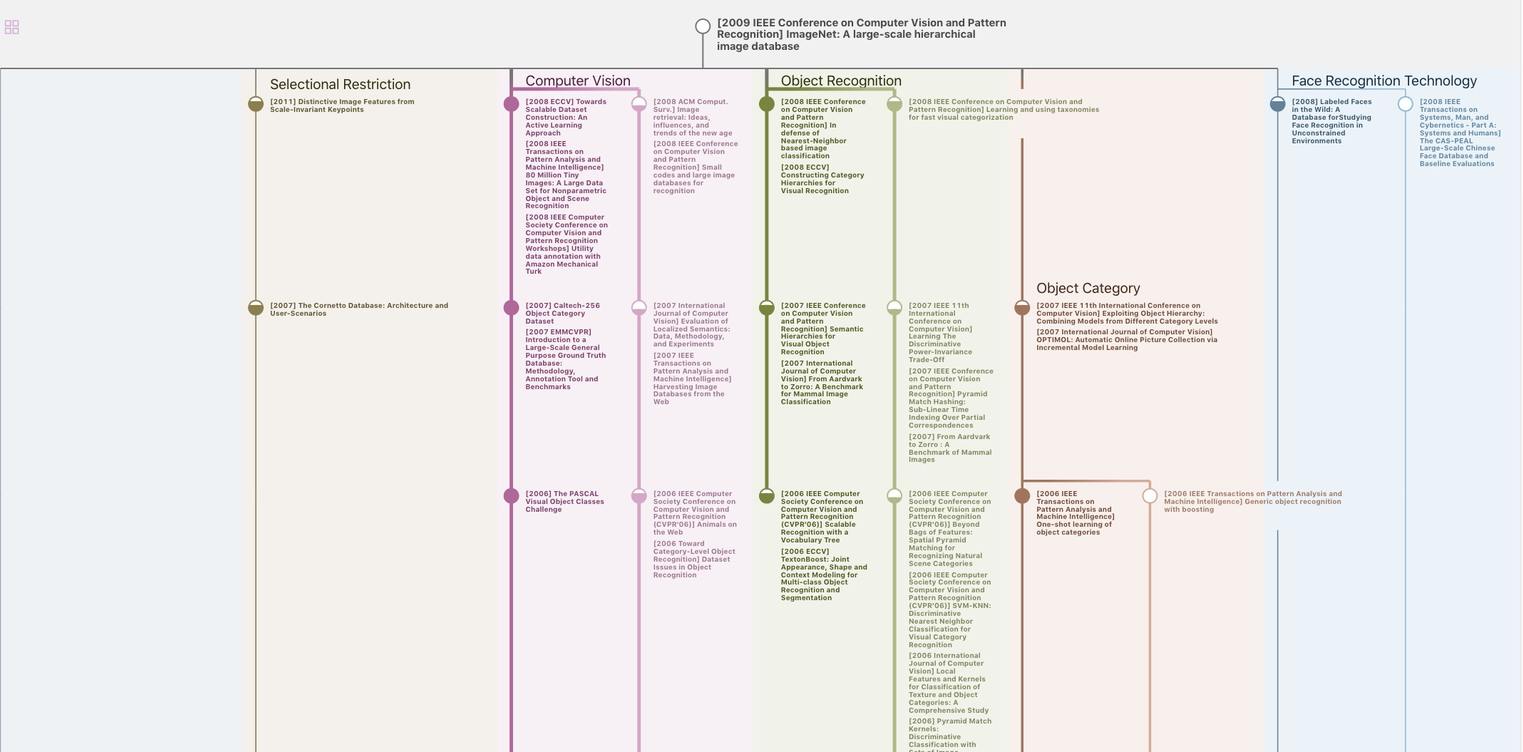
生成溯源树,研究论文发展脉络
Chat Paper
正在生成论文摘要