A Deep Reinforcement Learning Decision-Making Approach for Adaptive Cruise Control in Autonomous Vehicles
2023 21ST INTERNATIONAL CONFERENCE ON ADVANCED ROBOTICS, ICAR(2023)
摘要
In the evolving automobile industry, Adaptive Cruise Control (ACC) is key for aiding autonomous traffic navigation. Ideal ACC systems can decelerate to low speeds in stop-and-go traffic, maintain a safe following distance, minimize rear-end collision risks, and lessen the driver's need to continually adjust vehicle's speed to match traffic flow. In this paper, we offer a Deep Reinforcement Learning-based adaptive cruise control (DRL-ACC) system that creates safe, flexible, and responsive car-following policies agents. Instead of using discrete incremental and decremental values or a continuous action space, we suggest constructing a discrete high-level action space to accelerate, decelerate, and hold the current speed. We also provide a comprehensive, easyto-interpret multi-objective reward function that reflects safe, responsive, and rational traffic behavior. This strategy, trained on a single steady-state flow car-following scenario, promotes steadiness, responsiveness, and shows better generalization to diverse car-following scenarios. Results are also compared to the conventional Intelligent Driver Model (IDM). We further explore the model's potential to avoid rear-end collisions and facilitate future integration of lane-change maneuvers, which will increase its effectiveness in emergency situations.
更多查看译文
关键词
Adaptive Control,Autonomous Vehicles,Deep Reinforcement Learning,Adaptive Cruise Control,International Research Projects,Control Of Autonomous Vehicles,Traffic Flow,Reward Function,Discrete Action,Continuous Action Space,Lane Change Maneuver,Rear-end Collision,Average Speed,Speed Limit,Model Predictive Control,Markov Decision Process,Safe Distance,Observation Space,Driver Assistance,Advanced Driver Assistance Systems,Time Headway,Front Vehicle,Lateral Acceleration,Deep Reinforcement Learning Agent,Maximum Deceleration,Risk Zones,Occupancy Grid,Speed Modulation,Reward Rate,Double Deep Q-network
AI 理解论文
溯源树
样例
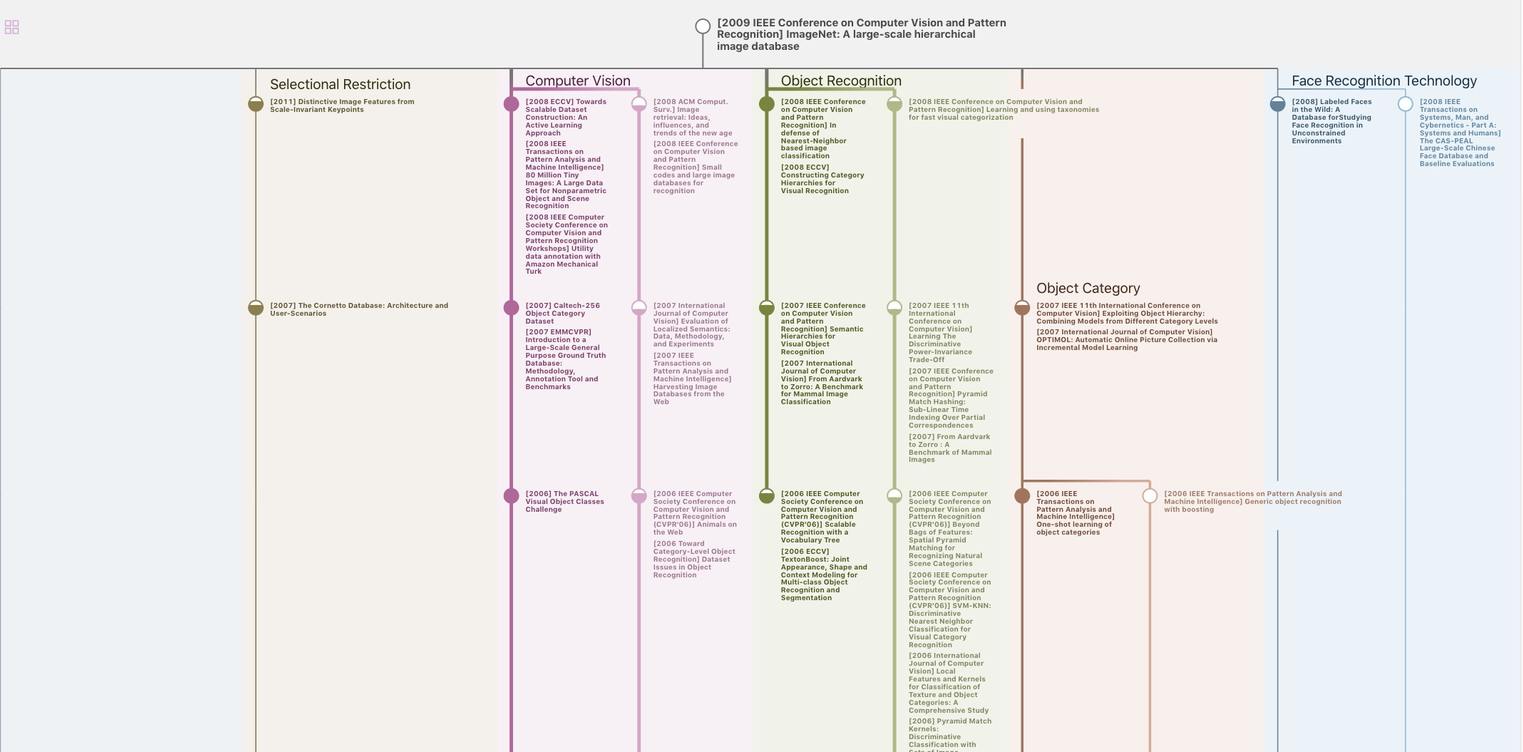
生成溯源树,研究论文发展脉络
Chat Paper
正在生成论文摘要