CT-Based Radiomics Analysis of Different Machine Learning Models for Discriminating the Risk Stratification of Pheochromocytoma and Paraganglioma: A Multicenter Study.
Academic radiology(2024)
摘要
RATIONALE AND OBJECTIVES:Using different machine learning models CT-based radiomics to integrate clinical radiological features to discriminating the risk stratification of pheochromocytoma/paragangliomas (PPGLs).
MATERIALS AND METHODS:The present study included 201 patients with PPGLs from three hospitals (training set: n = 125; external validation set: n = 45; external test set: n = 31). Patients were divided into low-risk and high-risk groups using a staging system for adrenal pheochromocytoma and paraganglioma (GAPP). We extracted and selected CT radiomics features, and built radiomics models using support vector machines (SVM), k-nearest neighbors, random forests, and multilayer perceptrons. Using receiver operating characteristic curve analysis to select the optimal radiomics model, a combined model was built using the output of the optimal radiomics model and clinical radiological features, and its accuracy and clinical applicability were evaluated using calibration curves and clinical decision curve analysis (DCA).
RESULTS:Finally, 13 radiomics features were selected to construct machine learning models. In the radiomics model, the SVM model demonstrated higher accuracy and stability, with an AUC value of 0.915 in the training set, 0.846 in external validation set, and 0.857 in external test set. Combining the outputs of SVM models with two clinical radiological features, a combined model constructed has demonstrated optimal risk stratification ability for PPGLs with an AUC of 0.926 for the training set, 0.883 for the external validation set, and 0.899 for the external test set. The calibration curve and DCA show good calibration accuracy and clinical effectiveness for the combined model.
CONCLUSION:Combined model that integrates radiomics and clinical radiological features can discriminate the risk stratification of PPGLs.
更多查看译文
AI 理解论文
溯源树
样例
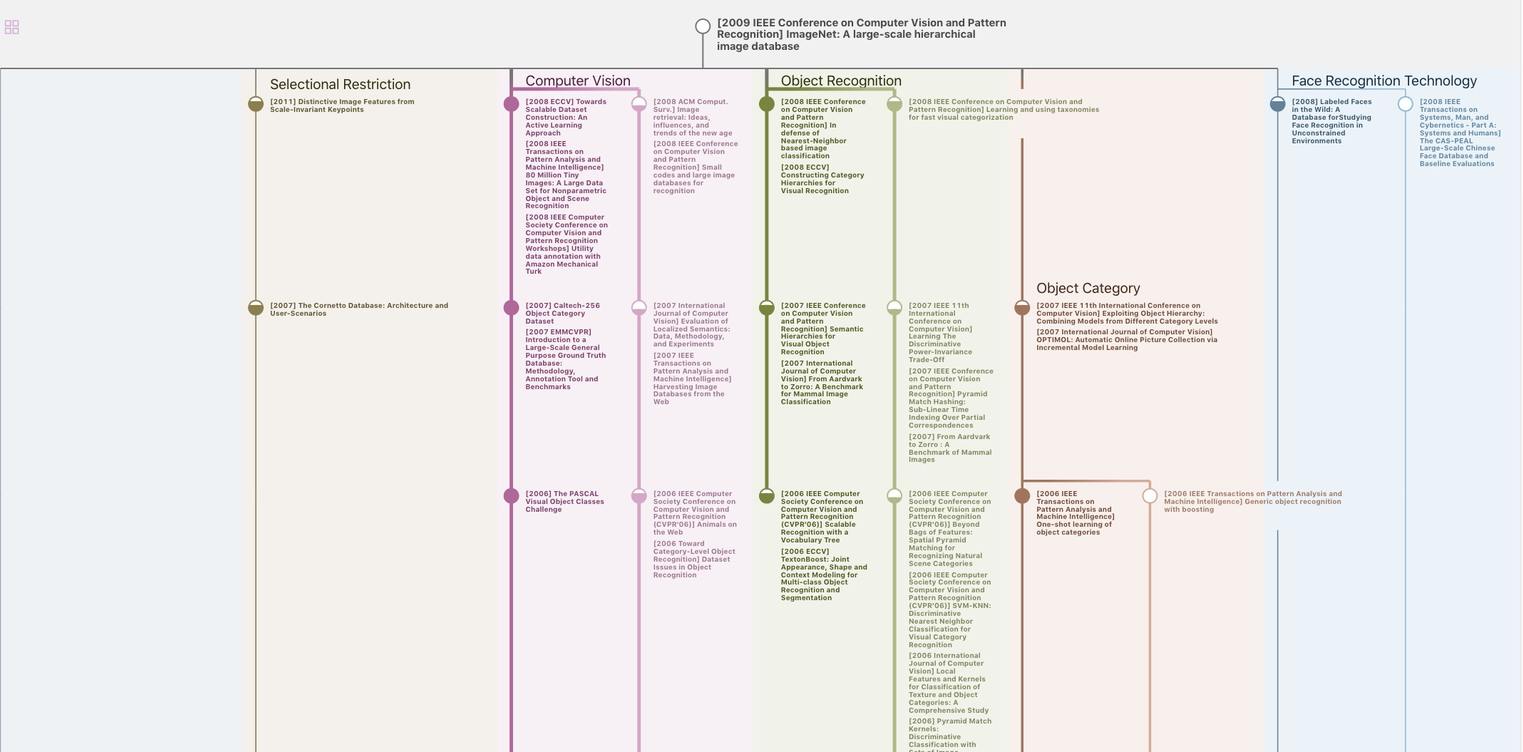
生成溯源树,研究论文发展脉络
Chat Paper
正在生成论文摘要