Peri-lesion Regions in Differentiating Suspicious Breast Calcification-Only Lesions Specifically on Contrast Enhanced Mammography.
Journal of X-ray science and technology(2024)
摘要
PURPOSE: The explore the added value of peri-calcification regions on contrast-enhanced mammography (CEM) in the differential diagnosis of breast lesions presenting as only calcification on routine mammogram. METHODS: Patients who underwent CEM because of suspicious calcification-only lesions were included. The test set included patients between March 2017 and March 2019, while the validation setwas collected between April 2019 and October 2019. The calcifications were automatically detected and grouped by a machine learning-based computer-aided system. In addition to extracting radiomic features on both low-energy (LE) and recombined (RC) images from the calcification areas, the peri-calcification regions, which is generated by extending the annotation margin radially with gradients from 1mm to 9 mm, were attempted. Machine learning (ML) models were built to classify calcifications into malignant and benign groups. The diagnostic matrices were also evaluated by combing ML models with subjective reading. RESULTS: Models for LE (significant features: wavelet-LLL glcm Imc2 MLO; wavelet-HLL firstorder Entropy MLO; wavelet-LHH glcm DifferenceVariance CC; wavelet-HLL glcm SumEntropy MLO;waveletHLH glrlm ShortRunLowGray LevelEmphasis MLO; original firstorder Entropy MLO; original shape Elongation MLO) and RC (significant features: wavelet-HLH glszm GrayLevelNonUniformityNormalized MLO; waveletLLH firstorder 10Percentile CC; original firstorder Maximum MLO; wavelet-HHH glcm Autocorrelation MLO; original shape Elongation MLO; wavelet-LHL glszm GrayLevelNonUniformityNormalized MLO; waveletLLH firstorder RootMeanSquared MLO) images were set up with 7 features. Areas under the curve (AUCs) of RC models are significantly better than those of LE models with compact and expanded boundary (RC v.s. LE, compact: 0.81 v.s. 0.73, p < 0.05; expanded: 0.89 v.s. 0.81, p < 0.05) and RC models with 3mm boundary extension yielded the best performance compared to those with other sizes (AUC= 0.89). Combining with radiologists' reading, the 3mm-boundary RC model achieved a sensitivity of 0.871 and negative predictive value of 0.937 with similar accuracy of 0.843 in predicting malignancy. CONCLUSIONS: The machine learning model integrating intra- and peri-calcification regions on CEM has the potential to aid radiologists' performance in predicting malignancy of suspicious breast calcifications.
更多查看译文
关键词
Breast neoplasms,calcifications,machine learning,mammography,contrast media
AI 理解论文
溯源树
样例
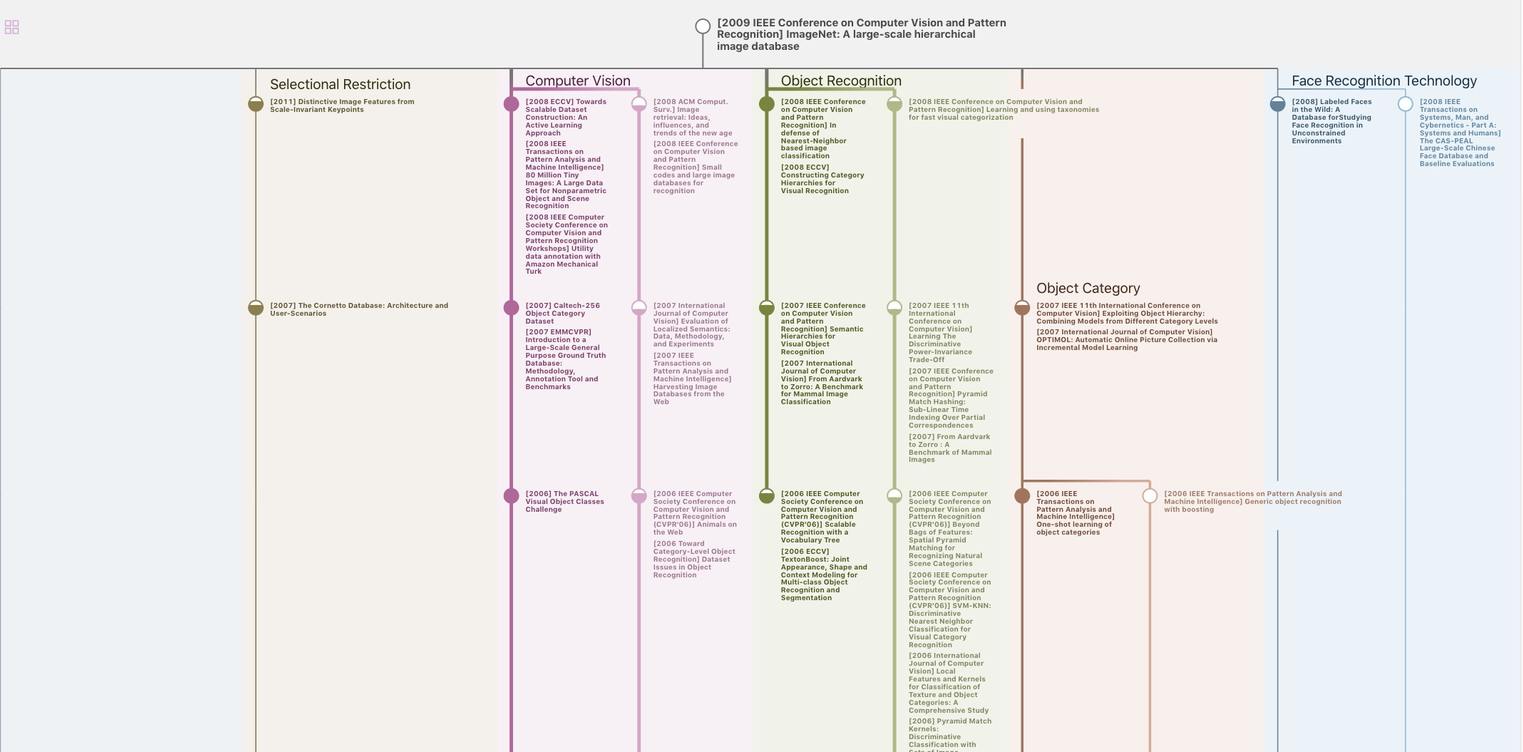
生成溯源树,研究论文发展脉络
Chat Paper
正在生成论文摘要