Feed Forward Cascaded Neural Network Enhanced Stochastic Predictive Model Control for Microgrid Energy Management
2023 IEEE International Conference on Energy Technologies for Future Grids (ETFG)(2023)
摘要
The integration of renewable energy sources, electric vehicles, and energy storage systems in microgrids introduces a higher level of uncertainty and variability in energy generation and consumption patterns. Stochastic Model Predictive Control (SMPC) has emerged as a promising technique for optimizing the operation of microgrids due to its ability to account for uncertainties, constraints, and dynamic interactions among various components. The paper evaluates the integration of wind and solar energy and electric ground support vehicle batteries (EGSVBs) into the green airport energy system. A mixed integer linear programming (MILP) algorithm is used to optimize the power flow between sources in the microgrid and EGSVBs, which can deliver surplus power back to the utility grid and charge and discharge EVBs based on demand. The study implements a novel approach, "Stochastic Model Predictive Control based Cascade Feed-forward Neural Network (SMPC-CFFNN)", on higher-order complex dynamic mathematical models for energy management and integrates the stochastic nature of renewable power generation. The effectiveness of the proposed SMPC-CFFNN approach is examined under different operating conditions and compared with the conventional SMPC controller. The results demonstrate that the proposed control strategy minimizes computing complexity and optimization time, and a zero-emission-based microgrid for a resilient green airport is achievable with the proposed energy management architecture.
更多查看译文
关键词
Stochastic model predictive control,Artificial neural network,cascade feed-forward,mixed-integer linear programming (MILP)
AI 理解论文
溯源树
样例
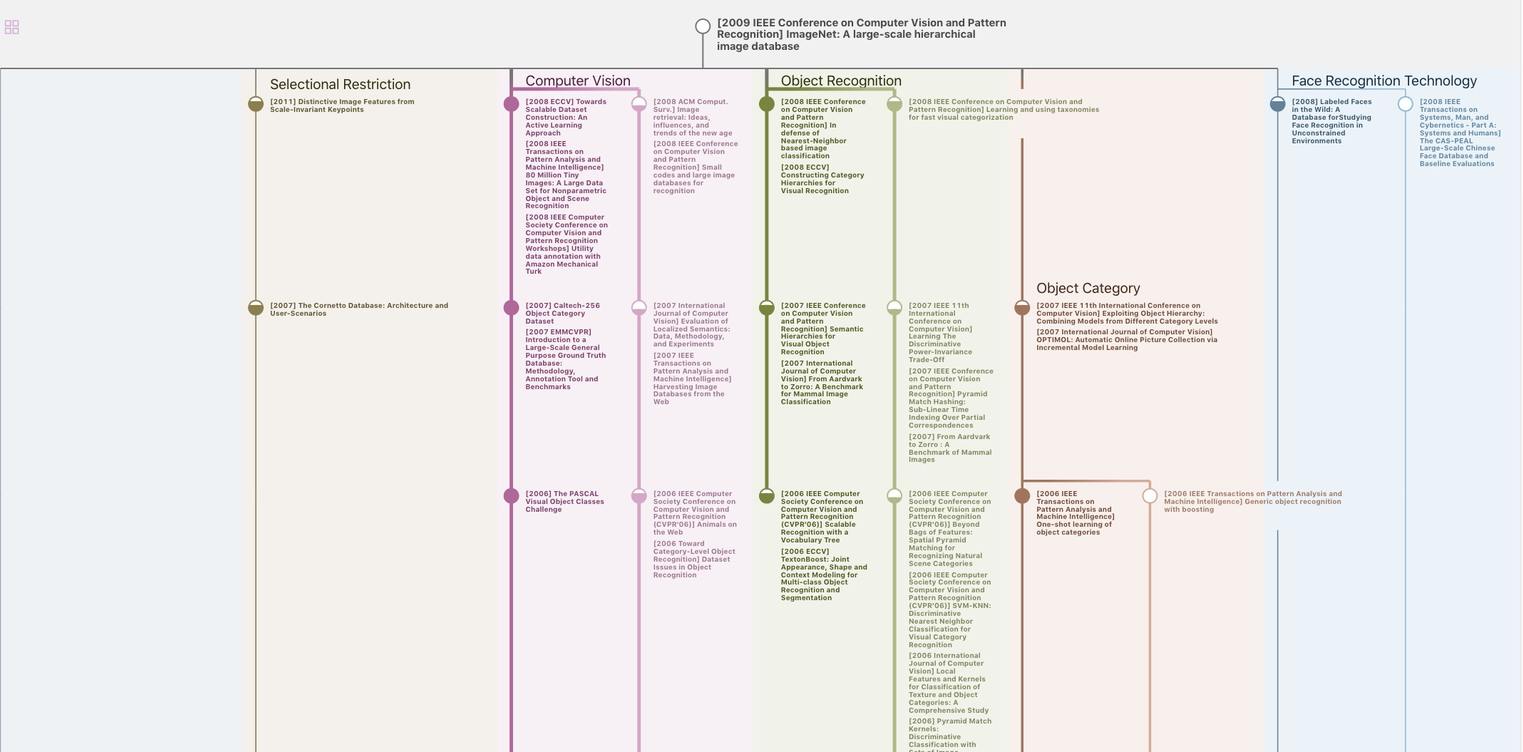
生成溯源树,研究论文发展脉络
Chat Paper
正在生成论文摘要