Deep learning approach for automated segmentation of myocardium using bone scintigraphy single-photon emission computed tomography/computed tomography in patients with suspected cardiac amyloidosis.
Journal of nuclear cardiology : official publication of the American Society of Nuclear Cardiology(2024)
摘要
BACKGROUND:We employed deep learning to automatically detect myocardial bone-seeking uptake as a marker of transthyretin cardiac amyloid cardiomyopathy (ATTR-CM) in patients undergoing 99mTc-pyrophosphate (PYP) or hydroxydiphosphonate (HDP) single-photon emission computed tomography (SPECT)/computed tomography (CT).
METHODS:We identified a primary cohort of 77 subjects at Brigham and Women's Hospital and a validation cohort of 93 consecutive patients imaged at the University of Pennsylvania who underwent SPECT/CT with PYP and HDP, respectively, for evaluation of ATTR-CM. Global heart regions of interest (ROIs) were traced on CT axial slices from the apex of the ventricle to the carina. Myocardial images were visually scored as grade 0 (no uptake), 1 (uptakeribs). A 2D U-net architecture was used to develop whole-heart segmentations for CT scans. Uptake was determined by calculating a heart-to-blood pool (HBP) ratio between the maximal counts value of the total heart region and the maximal counts value of the most superior ROI.
RESULTS:Deep learning and ground truth segmentations were comparable (p=0.63). A total of 42 (55%) patients had abnormal myocardial uptake on visual assessment. Automated quantification of the mean HBP ratio in the primary cohort was 3.1±1.4 versus 1.4±0.2 (p<0.01) for patients with positive and negative cardiac uptake, respectively. The model had 100% accuracy in the primary cohort and 98% in the validation cohort.
CONCLUSION:We have developed a highly accurate diagnostic tool for automatically segmenting and identifying myocardial uptake suggestive of ATTR-CM.
更多查看译文
AI 理解论文
溯源树
样例
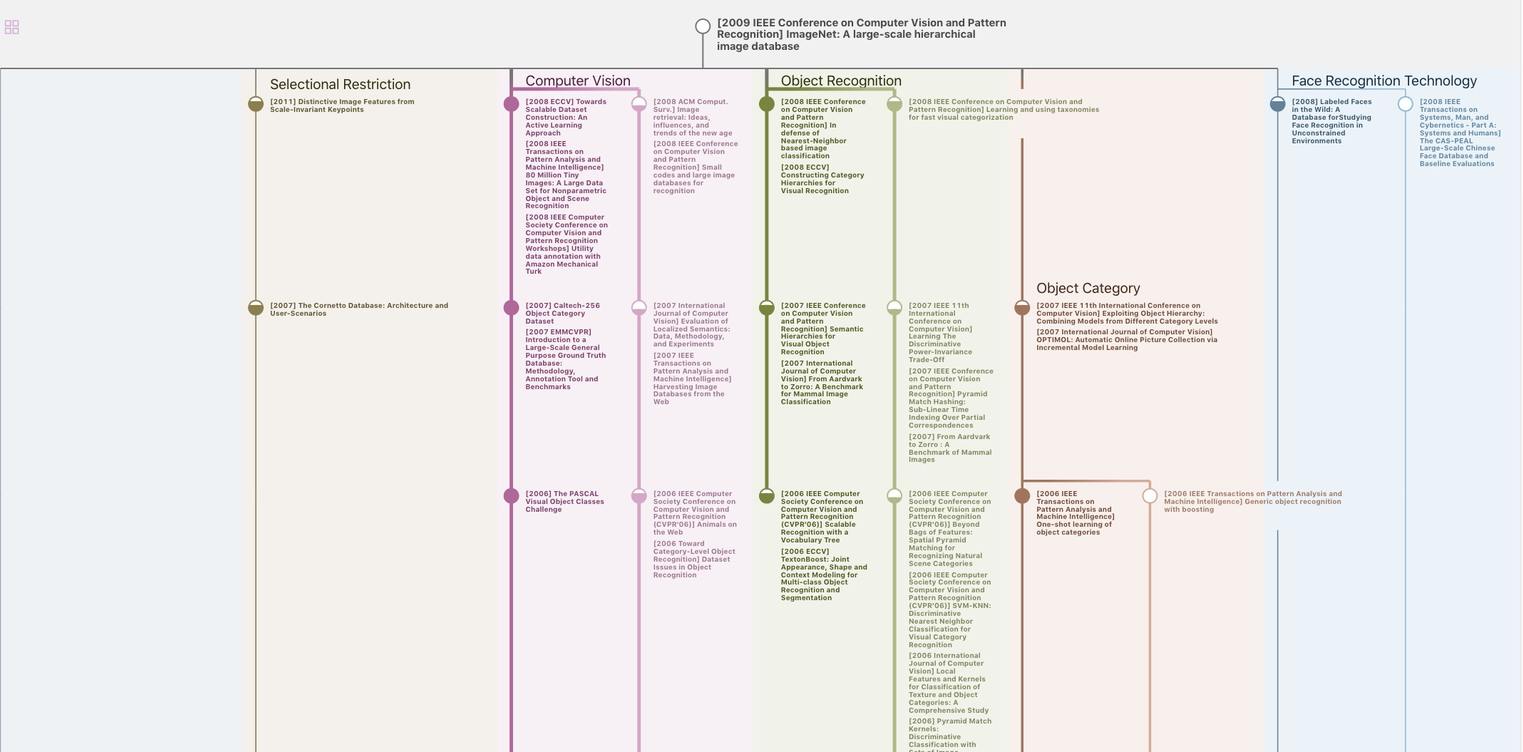
生成溯源树,研究论文发展脉络
Chat Paper
正在生成论文摘要