Multiparameter spectral CT-based radiomics in predicting the expression of programmed death ligand 1 in non-small-cell lung cancer
CLINICAL RADIOLOGY(2024)
摘要
AIM: To explore the value of radiomics for predicting the expression of programmed death ligand 1 (PD-L1) in non-small-cell lung cancer (NSCLC) based on multiparameter spectral computed tomography (CT) images. MATERIALS AND METHODS: A total of 220 patients with NSCLC were enrolled retrospectively and divided into the training (n=176) and testing (n=44) cohorts. The radiomics features were extracted from the conventional CT images, mono-energy 40 keV images, iodine density (ID) maps, Z-effective maps, and electron density maps. The logistic regression (LR) and support vector machine (SVM) algorithms were employed to build models based on radiomics signatures. The prediction abilities were qualified by the area under the curve (AUC) obtained from the receiver operating characteristic (ROC) curve. Internal validation was performed on the independent testing dataset. RESULTS: The combined model for PD-L1 >= 1%, which consisted of the radiomics score (rad-score; p<0.0001), white blood cell (WBC; p=0.027) counts, and air bronchogram (p=0.003), reached the highest performance with the AUCs of 0.873 and 0.917 in the training and testing dataset, respectively, which was better than the radiomics model with the AUCs of 0.842 and 0.886. The combined model for PD-L1 >= 50%, which consisted of rad-score (p<0.0001) and WBC counts (p=0.027), achieved the highest performance in the training and testing dataset with AUCs of 0.932 and 0.903, respectively, which was better than the radiomics model with AUCs of 0.920 and 0.892, respectively. CONCLUSION: The radiomics model based on the multiparameter images of spectral CT can predict the expression level of PD-L1 in NSCLC. The combined model can obtain higher prediction efficiency and serves as a promising method for immunotherapy selection. (c) 2024 The Royal College of Radiologists. Published by Elsevier Ltd. All rights reserved.
更多查看译文
AI 理解论文
溯源树
样例
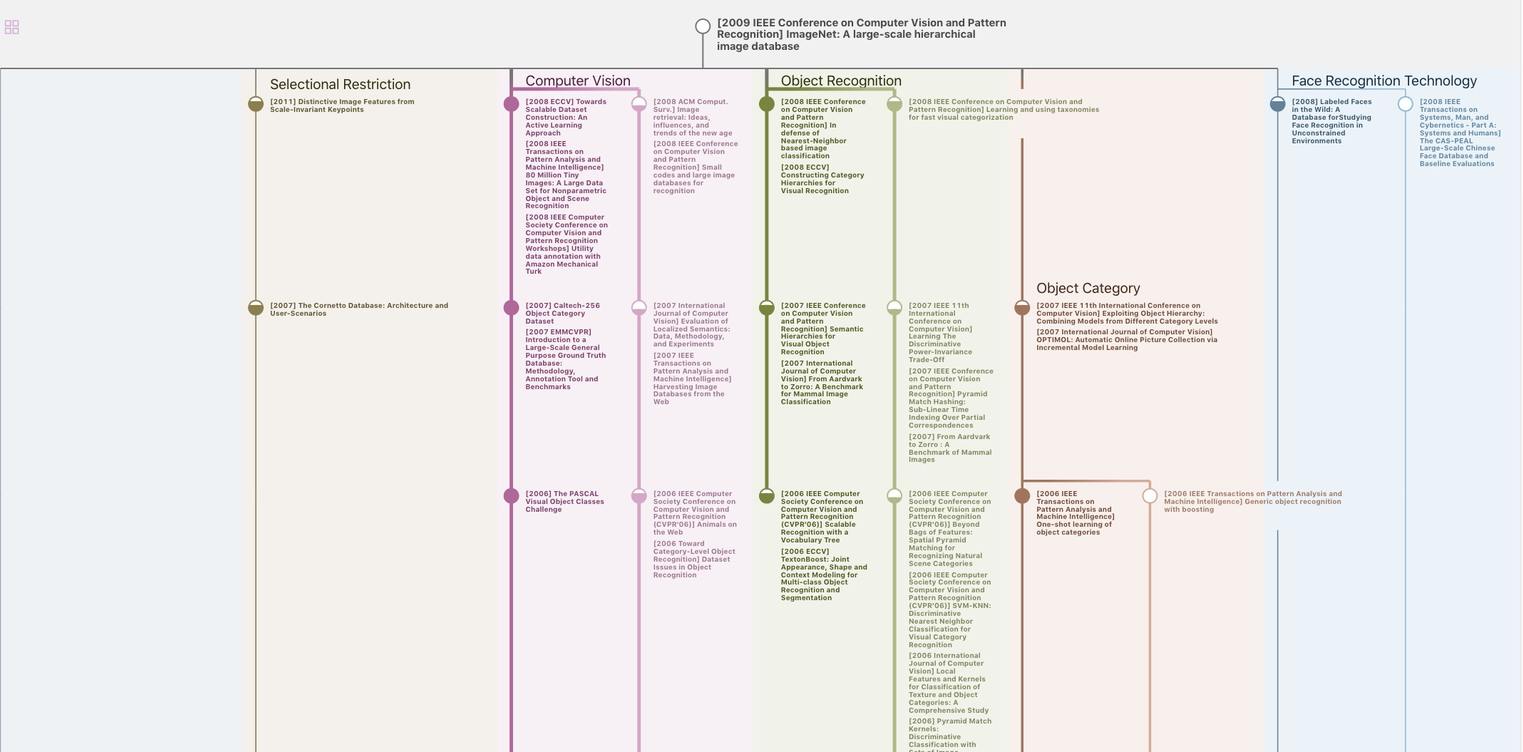
生成溯源树,研究论文发展脉络
Chat Paper
正在生成论文摘要