A fault detection strategy for an ePump during EOL tests based on a knowledge-based vibroacoustic tool and supervised machine learning classifiers
Meccanica(2024)
摘要
This paper presents a methodology for identifying faulty components in an electric pump during the end-of-line test based on accelerations and pressure pulsation data used to train an ensemble learning algorithm based on supervised machine learning classifiers. Despite various quality control measures in pump manufacturing, some out-of-tolerance components can pass through and end up on the assembly line, potentially leading to premature failure or abnormal noise during real-field operation. Because of the high impact, it is very important to put in place actions to mitigate the risk of delivering non-conform units, even if properly working in terms of pressure-flow rate performances. In this paper, an innovative knowledge-based vibroacoustic tool together with a machine learning built-in Python® library have been used to post-process acceleration and pressure pulsations data to generate features, which are then used to train, and test several supervised machine learning algorithms. The ensemble learning algorithm combines the best classifiers to identify healthy electric pump units with high accuracy, achieving above 95% accuracy in an experimental test campaign carried out on eighty electric pumps. Results are compared using principal component analysis for dimensionality reduction, and a sensor sensitivity study is conducted.
更多查看译文
关键词
External gear pump,Fault detection,The end of line (EOL) tests,Condition monitoring,Knowledge-based vibroacoustic model,Ensemble machine learning
AI 理解论文
溯源树
样例
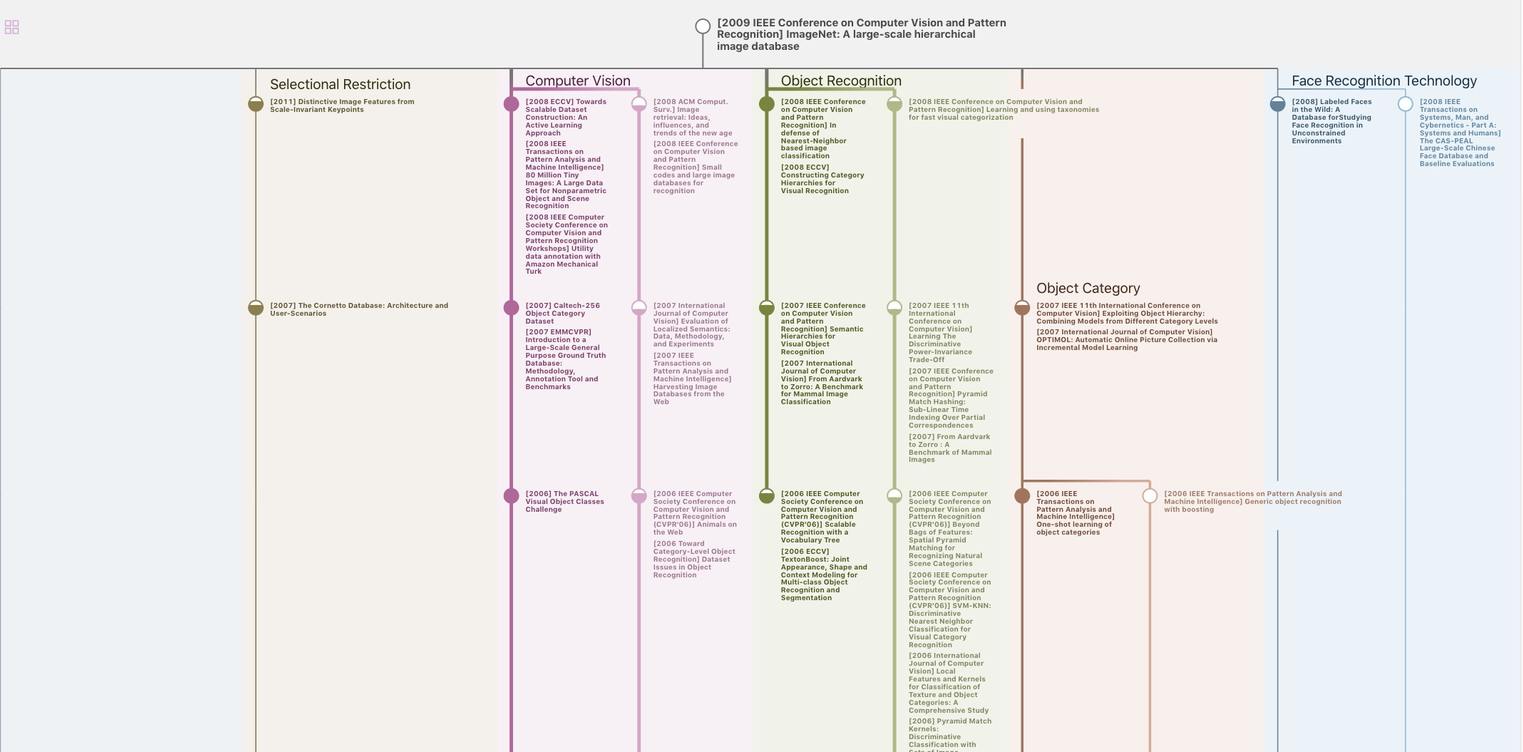
生成溯源树,研究论文发展脉络
Chat Paper
正在生成论文摘要