Global–local shrinkage multivariate logit-beta priors for multiple response-type data
Statistics and Computing(2024)
摘要
Multiple-type outcomes are often encountered in many statistical applications, one may want to study the association between multiple responses and determine the covariates useful for prediction. However, literature on variable selection methods for multiple-type data is arguably underdeveloped. In this article, we develop a novel global–local shrinkage prior in multiple response-types settings, where the observed dataset consists of multiple response-types (e.g., continuous, count-valued, Bernoulli trials, etc.), by combining the perspectives of global–local shrinkage and the conjugate multivaraite distribution. One benefit of our model is that a transformation or a Gaussian approximation on the data is not needed to perform variable selection for multiple response-type data, and thus one can avoid computational difficulties and restrictions on the joint distribution of the responses. Another benefit is that it allows one to parsimoniously model cross-variable dependence. Specifically, our method uses basis functions with random effects, which can be presented as known covariates or pre-defined basis functions, to model dependence between responses and dependence can be detected by our proposed global–local shrinkage model with a sparsity-inducing model. We provide connections to the original horseshoe model and existing basis function models. An efficient block Gibbs sampler is developed, which is found to be effective in obtaining accurate estimates and variable selection results. We also provide a motivating analysis of public health and financial costs from natural disasters in the U.S. using data provided by the National Centers for Environmental Information.
更多查看译文
关键词
Bayesian hierarchical model,Gibbs sampler,Markov Chain Monte Carlo,Multiple response-types,Spike and slab
AI 理解论文
溯源树
样例
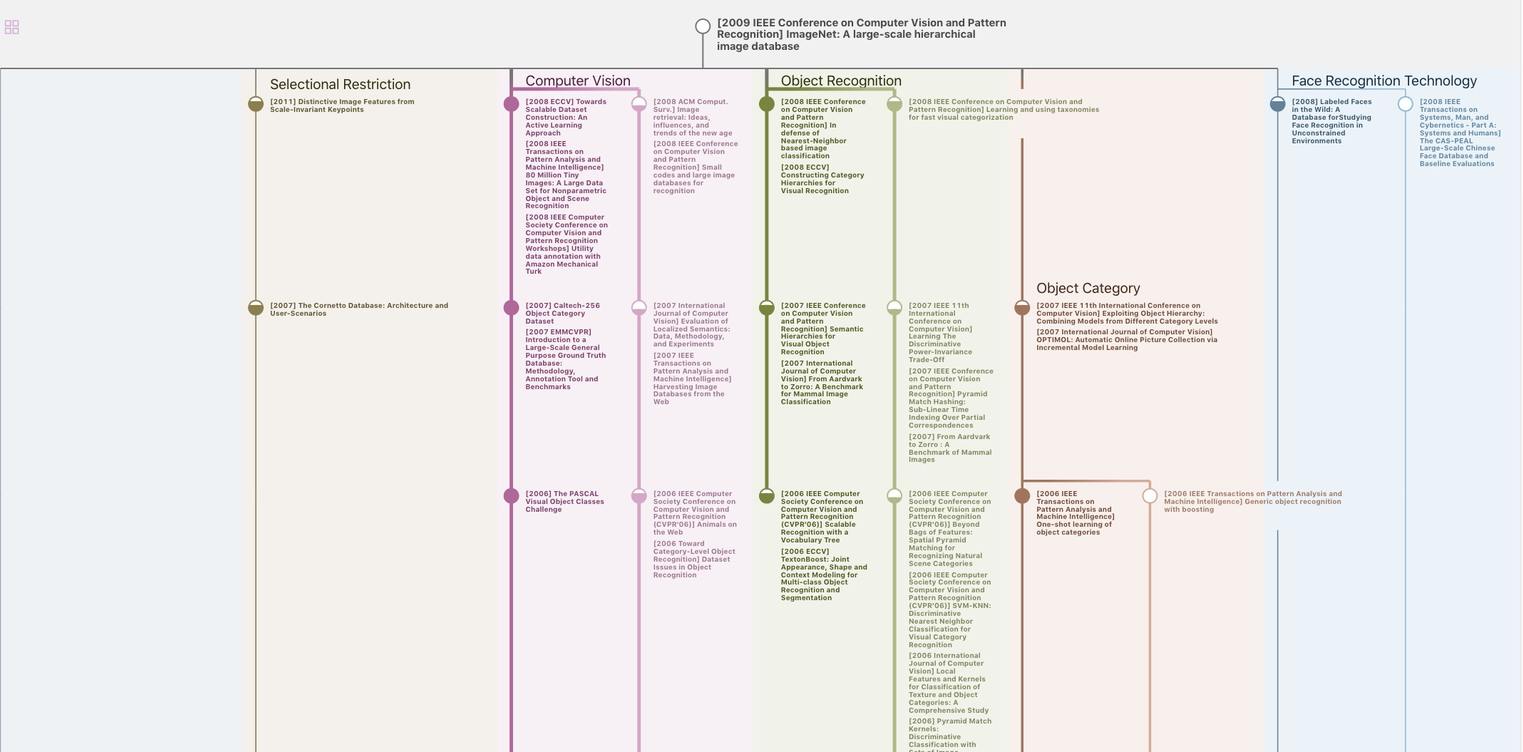
生成溯源树,研究论文发展脉络
Chat Paper
正在生成论文摘要